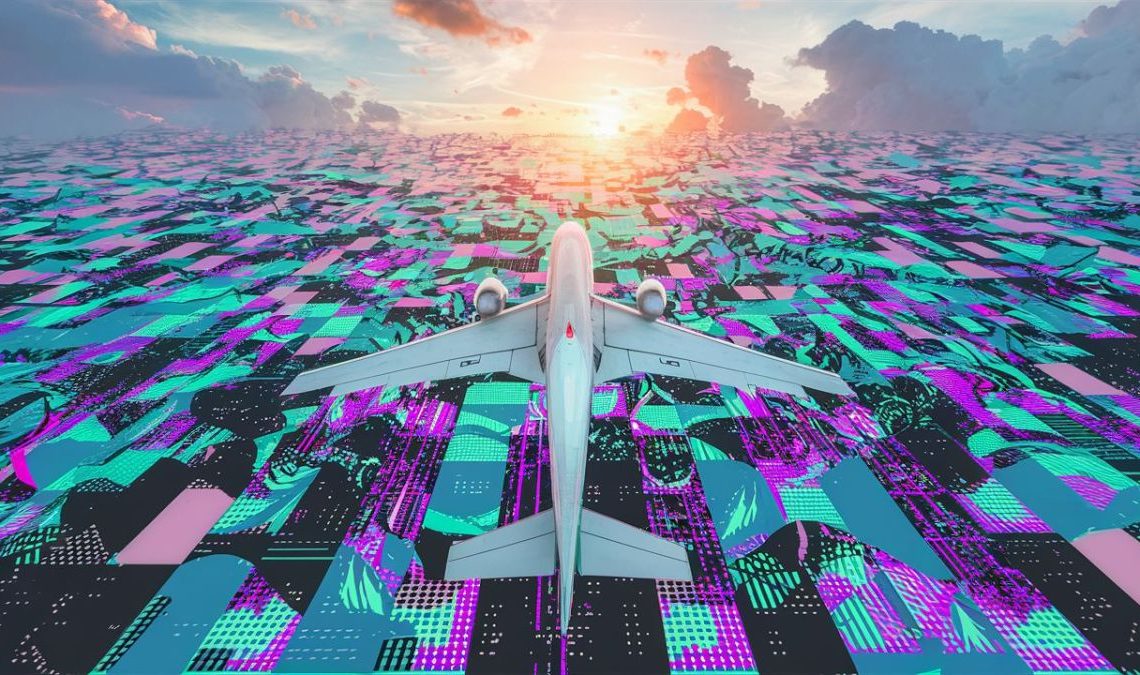
Today, Boston-based Metaplane, a startup looking to improve and rectify data quality issues for enterprises, announced it has raised $13.8 million in a series A round of funding. Venture Capital firm Felicis led the investment with participation from Khosla Ventures, Flybridge, Y Combinator, Stage 2 Capital, B37 and SNR.
Metaplane said it plans to use the round to develop its AI-powered data observability platform further and become the “indisputably most powerful, configurable and magical-to-set-up solution to trust data.”
The company, founded by MIT graduate Kevin Hu, former HubSpot engineer Peter Casinelli and ex-Appcues developer Guru Mahendran, is taking on the heavily funded players like Monte Carlo, Observe and Acceldata in the rapidly evolving data observability space. It has grown its customer base three-fold over the past year and is already working with brands such as Bose, Sigma, Klaviyo and ClickUp.
Data has become the driving force of modern businesses, enabling teams to not only analyze historical patterns for decision-making but also predict growth-critical aspect — such as the inventory plan for a particular event.
The surge of generative AI applications has also motivated companies to stitch data together from different sources and hopefully, drive further value.
However, given this dramatic shift to data-driven efforts, it has become difficult for teams to keep tabs on all the information they have for quality issues.
The pipelines have become more complex, with sometimes hundreds or thousands of sources to wrangle.
Metaplane applies AI to the problem, which it says enables enterprises to proactively watch out for data incidents at different layers of their data ecosystem.
“We integrate with as much of the data stack as possible, whether that’s ingestion tools like Fivetran, cloud data warehouses like Snowflake and BigQuery, transformation and orchestration layers like dbt and Airflow, reverse ETL tools like Census and Hightouch, and BI tools like Sigma, Tableau, and Looker. We go even further by being the only data observability product to integrate with transactional databases like Postgres and MySQL, and catch issues within dbt pull requests in Github,” Hu, who started the company from an MIT project in 2019, told VentureBeat.
Once the platform integrates with the data stack, the user can set up monitors on heavily used/updated tables to keep an eye on different data quality metrics such as freshness, row count, uniqueness and nullness. The entire process takes about 15 minutes, following which the product gets to work with AI.
As Hu explained, the system’s machine learning (ML) model trains on the data profile, using historical metadata, and then starts flagging data anomalies (even schema changes) within a day or two. The whole thing is fully automated, with alerts going directly to concerned data teams on the preferred destination for alerts.
“We use the most historical data to train our models, ensuring that we can capture seasonality and avoid repetitive alerts. Every business is unique and simply applying a one-size-fits-all model to each customer introduces a lot of inaccuracy. Unlike other monitoring tools, we also make it easy for users to tweak models to ignore one-offs or learn new trends to account for seasonal patterns and factors specific to their industry. Customers go with us because we catch issues that others can’t while keeping the noise to a minimum,” Hu explained.
Notably, in addition to monitoring metrics like freshness and volume of data, Metaplane can also go deeper to detect data problems that are very domain-specific with finer-grain controls, including monitoring for changes in data usage and cloud warehouse spend. Plus, the coverage of the data stack allows the platform to create a complete picture of column-level lineage from data source to destination and provide context on the downstream impact of issues as well as upstream root causes.
While Metaplane is not as heavily funded as its competitors Observe, Acceldata and Monte Carlo, the company has been doing pretty well in the data observability space. In 2023, its ARR grew six-fold while the customer base grew three-fold to over 100 enterprises – with known names like Klaviyo, Bose, ClickUp, Sigma, Census, GoFundMe and Ramp coming on board.
As of January 2024, the company said, these customers had run 500 million data quality checks on over 40 million data assets and over 30 million data lineage connections, detecting and resolving as many as 80,000 incidents.
“We believe that all companies should be able to trust their data, and so we enable teams to sign up and use it for free. As a result, we’ve benefited greatly from organic growth and more users have used Metaplane than any other data observability tool,” the founder emphasized.
In addition to the self-serve approach to adoption, Hu claimed that the platform’s ability to detect important issues while keeping noise to the minimum and give a complete view of the data stack makes it better than all other observability tools out there.
“Am I monitoring everything that could possibly introduce errors into the data? How many issues stem from transactional databases? How many could be stopped by preventing a code change? The only way to answer these questions is to integrate deeply across the data stack, in all the places that data issues can be produced or impacted. We recently announced our integrations with Census and Hightouch, the two leading reverse ETL platforms, and have more announcements there coming soon,” Hu added.
Moving ahead, the company plans to use the capital to focus on R&D and further develop its data observability platform for enterprise teams looking to use their data assets with confidence. Part of this will go towards automating more of the monitoring architecture while introducing support for observing even more metrics, sources and connections between the sources.
“Our vision is that our platform will learn from each customer’s unique requirements and recommend an ideal monitoring and alerting architecture based on their evolving needs over time. We will couple this with a broad expansion of what we monitor, adding both deeper metrics and a wider scope of metrics, to observe everything in the data stack so our customers always have the necessary context to find and fix data quality issues,” Hu noted.
Join leaders in Boston on March 27 for an exclusive night of networking, insights, and conversation. Request an invite here.
Today, Boston-based Metaplane, a startup looking to improve and rectify data quality issues for enterprises, announced it has raised $13.8 million in a series A round of funding. Venture Capital firm Felicis led the investment with participation from Khosla Ventures, Flybridge, Y Combinator, Stage 2 Capital, B37 and SNR.
Metaplane said it plans to use the round to develop its AI-powered data observability platform further and become the “indisputably most powerful, configurable and magical-to-set-up solution to trust data.”
The company, founded by MIT graduate Kevin Hu, former HubSpot engineer Peter Casinelli and ex-Appcues developer Guru Mahendran, is taking on the heavily funded players like Monte Carlo, Observe and Acceldata in the rapidly evolving data observability space. It has grown its customer base three-fold over the past year and is already working with brands such as Bose, Sigma, Klaviyo and ClickUp.
Monitoring and flagging issues across the data stack
Data has become the driving force of modern businesses, enabling teams to not only analyze historical patterns for decision-making but also predict growth-critical aspect — such as the inventory plan for a particular event.
The surge of generative AI applications has also motivated companies to stitch data together from different sources and hopefully, drive further value.
However, given this dramatic shift to data-driven efforts, it has become difficult for teams to keep tabs on all the information they have for quality issues.
The pipelines have become more complex, with sometimes hundreds or thousands of sources to wrangle.
Metaplane applies AI to the problem, which it says enables enterprises to proactively watch out for data incidents at different layers of their data ecosystem.
“We integrate with as much of the data stack as possible, whether that’s ingestion tools like Fivetran, cloud data warehouses like Snowflake and BigQuery, transformation and orchestration layers like dbt and Airflow, reverse ETL tools like Census and Hightouch, and BI tools like Sigma, Tableau, and Looker. We go even further by being the only data observability product to integrate with transactional databases like Postgres and MySQL, and catch issues within dbt pull requests in Github,” Hu, who started the company from an MIT project in 2019, told VentureBeat.
Monitoring data quality with machine learning
Once the platform integrates with the data stack, the user can set up monitors on heavily used/updated tables to keep an eye on different data quality metrics such as freshness, row count, uniqueness and nullness. The entire process takes about 15 minutes, following which the product gets to work with AI.
As Hu explained, the system’s machine learning (ML) model trains on the data profile, using historical metadata, and then starts flagging data anomalies (even schema changes) within a day or two. The whole thing is fully automated, with alerts going directly to concerned data teams on the preferred destination for alerts.
“We use the most historical data to train our models, ensuring that we can capture seasonality and avoid repetitive alerts. Every business is unique and simply applying a one-size-fits-all model to each customer introduces a lot of inaccuracy. Unlike other monitoring tools, we also make it easy for users to tweak models to ignore one-offs or learn new trends to account for seasonal patterns and factors specific to their industry. Customers go with us because we catch issues that others can’t while keeping the noise to a minimum,” Hu explained.
Notably, in addition to monitoring metrics like freshness and volume of data, Metaplane can also go deeper to detect data problems that are very domain-specific with finer-grain controls, including monitoring for changes in data usage and cloud warehouse spend. Plus, the coverage of the data stack allows the platform to create a complete picture of column-level lineage from data source to destination and provide context on the downstream impact of issues as well as upstream root causes.
80,000 data quality incidents resolved
While Metaplane is not as heavily funded as its competitors Observe, Acceldata and Monte Carlo, the company has been doing pretty well in the data observability space. In 2023, its ARR grew six-fold while the customer base grew three-fold to over 100 enterprises – with known names like Klaviyo, Bose, ClickUp, Sigma, Census, GoFundMe and Ramp coming on board.
As of January 2024, the company said, these customers had run 500 million data quality checks on over 40 million data assets and over 30 million data lineage connections, detecting and resolving as many as 80,000 incidents.
“We believe that all companies should be able to trust their data, and so we enable teams to sign up and use it for free. As a result, we’ve benefited greatly from organic growth and more users have used Metaplane than any other data observability tool,” the founder emphasized.
In addition to the self-serve approach to adoption, Hu claimed that the platform’s ability to detect important issues while keeping noise to the minimum and give a complete view of the data stack makes it better than all other observability tools out there.
“Am I monitoring everything that could possibly introduce errors into the data? How many issues stem from transactional databases? How many could be stopped by preventing a code change? The only way to answer these questions is to integrate deeply across the data stack, in all the places that data issues can be produced or impacted. We recently announced our integrations with Census and Hightouch, the two leading reverse ETL platforms, and have more announcements there coming soon,” Hu added.
Moving ahead, the company plans to use the capital to focus on R&D and further develop its data observability platform for enterprise teams looking to use their data assets with confidence. Part of this will go towards automating more of the monitoring architecture while introducing support for observing even more metrics, sources and connections between the sources.
“Our vision is that our platform will learn from each customer’s unique requirements and recommend an ideal monitoring and alerting architecture based on their evolving needs over time. We will couple this with a broad expansion of what we monitor, adding both deeper metrics and a wider scope of metrics, to observe everything in the data stack so our customers always have the necessary context to find and fix data quality issues,” Hu noted.
VentureBeat’s mission is to be a digital town square for technical decision-makers to gain knowledge about transformative enterprise technology and transact. Discover our Briefings.
Author: Shubham Sharma
Source: Venturebeat
Reviewed By: Editorial Team