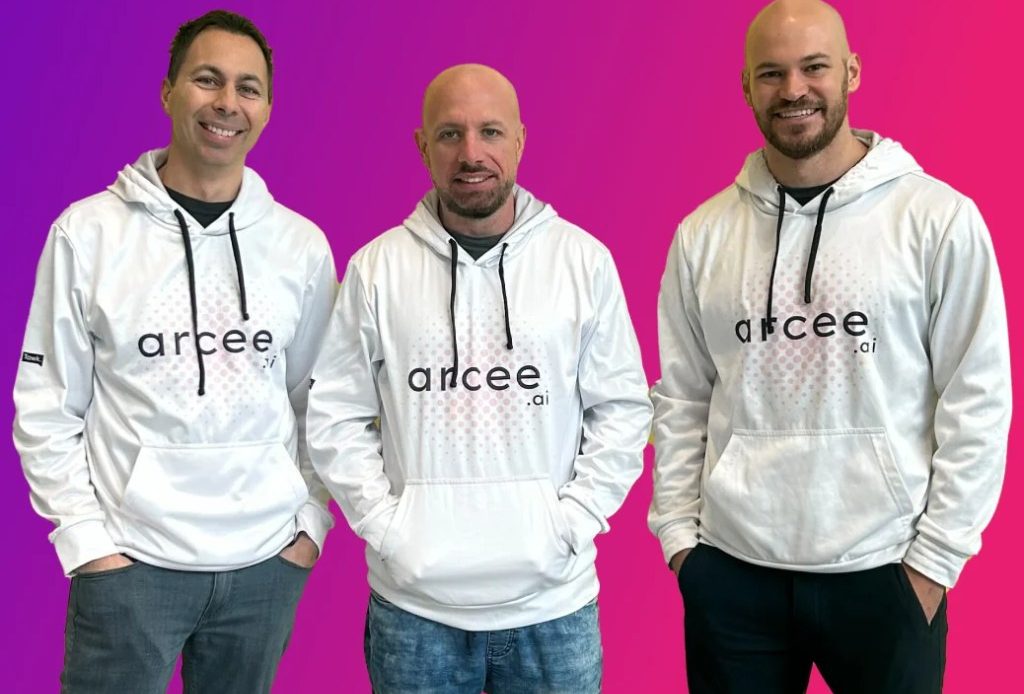
The trend toward small language models is accelerating as Arcee AI announced its $24M Series A funding only 6 months after announcing its $5.5M seed round in January 2024. The company also announced the launch of Arcee Cloud, a hosted SaaS version of their platform. This new offering complements their existing in-VPC deployment option, Arcee Enterprise.
The new round, led by Emergence Capital, signals growing investor confidence in the potential of smaller, more efficient AI models. “The Series A gives us the resources to bring our solution to the masses via our new cloud platform,” said Arcee AI Co-Founder and CEO Mark McQuade in an exclusive interview with VentureBeat.
Small language models (SLMs) are quickly becoming a go-to solution for enterprises in specific domains, particularly for question-answering applications. “If you want a model for your HR use case, you don’t care that it knows who won the Academy Awards for Best Picture in 1967,” McQuade said. “We’ve seen great success with models that are as small as 7 billion parameters.”
McQuade highlighted several use cases, including tax assistance, educational support, HR inquiries, and medical question-answering. Unlike data extraction or automated analysis tasks, these applications focus on providing accurate, context-aware responses to user queries. The versatility of SLMs in handling these specialized Q&A tasks efficiently makes them attractive across diverse industries, from finance to healthcare.
As we noted back in April, SLMs are beginning to challenge the “bigger is always better” approach, offering benefits in cost, energy efficiency, and specialized applications. McQuade says Arcee can train a GPT-3-like model for as little as $20,000.
“You don’t need to go that big for business use cases,” he explained. SLMs are faster to deploy, more easily customizable, and can run on smaller hardware setups. SLMs can also be more secure and less prone to hallucinations within their specialized domains.
Microsoft and Google are also rapidly advancing SLM technology, challenging the notion that AI requires massive scale. Microsoft’s Phi series includes the 2.7 billion parameter Phi-2 and the Phi-3 family, which ranges up to 14 billion parameters. In February 2024, Google released its Gemma series, optimized for consumer hardware. Gemma offers two variants: a 2 billion parameter model and a 7 billion parameter version. Both models run on standard laptops and desktops, broadening access to advanced AI capabilities.
These developments signal a shift in AI strategy, emphasizing efficiency and accessibility alongside raw power. “We’re seeing massive customer appreciation for Model Merging and Spectrum, and overall demand for our SLMs,” said McQuade.
Arcee AI enters this competitive landscape with a unique approach. While Microsoft and Google focus on general-purpose SLMs, Arcee specializes in domain-specific models and tools for enterprises to create their own. “We’re enabling organizations to expand beyond one high ROI use case,” McQuade explained. “With our efficiency, they can tackle 10 or 20 use cases.”
This strategy aligns with the growing demand for cost-effective, energy-efficient AI solutions that can also be deployed at the edge. Arcee’s Model Merging and Spectrum technologies aim to deliver these benefits while allowing for greater customization than off-the-shelf models.
Model merging, a key training technique in Arcee’s solution, allows the combination of multiple AI models into a single, more capable model without increasing its size. “We look at model merging as the next form of transfer learning,” McQuade explained. The process involves fusing the layers of different models, taking the best aspects of each to create a hybrid.
For example, when merging two 7 billion parameter models, the result is still a 7 billion parameter model, not a 14 billion parameter one. “You take the best pieces of the layers of each model and fuse them into one,” McQuade said. This technique allows Arcee to create models that possess the strengths of multiple specialized models while maintaining the efficiency and lower computational requirements of a smaller model.
The company’s approach allows users to set the weight and density of the fusion, controlling how much is taken from each input model. This flexibility enables the creation of highly tailored models that can outperform larger, more general models in specific domains.
Arcee’s Spectrum represents a significant advancement in the efficiency of language model training. This technique targets specific layer modules within the model based on their signal-to-noise ratio (SNR), while keeping others frozen. “Spectrum optimizes training time up to 42% and reduces catastrophic forgetting, without any performance degradation,” explained Lucas Atkins, Research Engineer at Arcee AI.
The importance of Spectrum lies in its ability to dramatically reduce the resources required for model training. Traditional methods often involve updating all parameters of a model during training, which can be computationally expensive and time-consuming. Spectrum’s selective approach allows for more efficient use of computational resources, potentially lowering the barriers to entry for organizations looking to develop custom AI models.
“We’ve built Spectrum into our training pipeline from the ground up, offering industry-leading training speed without sacrificing model quality,” Atkins added. This efficiency could enable faster iteration and more cost-effective model development for enterprises.
As performance gains in LLMs show signs of plateauing, the future of AI may increasingly lie in these more efficient, specialized models. McQuade said, “It no longer has to be one high ROI use case. You can do 10 use cases, 20 use cases, because we’re so efficient.” This shift towards SLMs could potentially democratize AI access across industries, making advanced AI capabilities more accessible and tailored to specific business needs.
McQuade emphasized, “Everything’s an annual contract, which is pretty unique in this space.” The pricing model is based on software licensing, moving away from the traditional usage-based pricing common in AI services. McQuade described it as “a software license” and mentioned that they also charge for inference.
Arcee AI offers its technology through two main product offerings:
Arcee has two delivery methods:
McQuade noted that the VPC option “really resonates with the larger companies.” The annual contract model allows for expansion. As McQuade put it, “if you want new models or whatever, then we expand you.” They also offer additional support and managed services for customers who want a more hands-off approach.
This pricing and sales model is designed to provide continuous value to customers while ensuring a steady, predictable revenue stream for Arcee AI. “We’re at $2 million in revenue now, and there’s a good chance we could turn profitable by early 2025,” McQuade revealed. The company, currently at 25 employees, plans to grow to about 50 within 18 months.
The true power of SLMs lies not just in their domain specificity, but in empowering companies to experiment, learn, and optimize AI continuously. The ability to rapidly iterate and develop models at a lower cost could become the decisive factor in successful AI adoption.
AI development may begin to resemble a more iterative, experimental process, with companies treating their AI models as living systems that evolve and adapt. Agility could soon become as important as model performance. This mirrors the evolution of software development, where agile methodologies and continuous integration/continuous deployment (CI/CD) practices have now become standard.
A faster iteration cycle creates real competitive advantages. Companies using SLMs can swiftly adapt to changing user needs, refining models based on real-world feedback. Instead of putting all resources into one high-stakes AI implementation, companies can explore multiple use cases simultaneously, identifying the most impactful applications for their business without breaking the bank.
If Arcee is successful in delivering its vision of efficient, domain-specific small language models that can be rapidly iterated and customized, it could be well-positioned just at the right time when agility is becoming critical in AI development. This could transform the company into a highly valuable enterprise. The coming months will reveal whether small language models truly have an edge in the competitive AI landscape, potentially reshaping the industry’s approach to model development and deployment.
Join our daily and weekly newsletters for the latest updates and exclusive content on industry-leading AI coverage. Learn More
The trend toward small language models is accelerating as Arcee AI announced its $24M Series A funding only 6 months after announcing its $5.5M seed round in January 2024. The company also announced the launch of Arcee Cloud, a hosted SaaS version of their platform. This new offering complements their existing in-VPC deployment option, Arcee Enterprise.
The new round, led by Emergence Capital, signals growing investor confidence in the potential of smaller, more efficient AI models. “The Series A gives us the resources to bring our solution to the masses via our new cloud platform,” said Arcee AI Co-Founder and CEO Mark McQuade in an exclusive interview with VentureBeat.
Small language models (SLMs) are quickly becoming a go-to solution for enterprises in specific domains, particularly for question-answering applications. “If you want a model for your HR use case, you don’t care that it knows who won the Academy Awards for Best Picture in 1967,” McQuade said. “We’ve seen great success with models that are as small as 7 billion parameters.”
McQuade highlighted several use cases, including tax assistance, educational support, HR inquiries, and medical question-answering. Unlike data extraction or automated analysis tasks, these applications focus on providing accurate, context-aware responses to user queries. The versatility of SLMs in handling these specialized Q&A tasks efficiently makes them attractive across diverse industries, from finance to healthcare.
The rapid rise of SLMs
As we noted back in April, SLMs are beginning to challenge the “bigger is always better” approach, offering benefits in cost, energy efficiency, and specialized applications. McQuade says Arcee can train a GPT-3-like model for as little as $20,000.
“You don’t need to go that big for business use cases,” he explained. SLMs are faster to deploy, more easily customizable, and can run on smaller hardware setups. SLMs can also be more secure and less prone to hallucinations within their specialized domains.
Microsoft and Google are also rapidly advancing SLM technology, challenging the notion that AI requires massive scale. Microsoft’s Phi series includes the 2.7 billion parameter Phi-2 and the Phi-3 family, which ranges up to 14 billion parameters. In February 2024, Google released its Gemma series, optimized for consumer hardware. Gemma offers two variants: a 2 billion parameter model and a 7 billion parameter version. Both models run on standard laptops and desktops, broadening access to advanced AI capabilities.
These developments signal a shift in AI strategy, emphasizing efficiency and accessibility alongside raw power. “We’re seeing massive customer appreciation for Model Merging and Spectrum, and overall demand for our SLMs,” said McQuade.
Arcee AI enters this competitive landscape with a unique approach. While Microsoft and Google focus on general-purpose SLMs, Arcee specializes in domain-specific models and tools for enterprises to create their own. “We’re enabling organizations to expand beyond one high ROI use case,” McQuade explained. “With our efficiency, they can tackle 10 or 20 use cases.”
This strategy aligns with the growing demand for cost-effective, energy-efficient AI solutions that can also be deployed at the edge. Arcee’s Model Merging and Spectrum technologies aim to deliver these benefits while allowing for greater customization than off-the-shelf models.
Model Merging: Combining the best from multiple AI models
Model merging, a key training technique in Arcee’s solution, allows the combination of multiple AI models into a single, more capable model without increasing its size. “We look at model merging as the next form of transfer learning,” McQuade explained. The process involves fusing the layers of different models, taking the best aspects of each to create a hybrid.
For example, when merging two 7 billion parameter models, the result is still a 7 billion parameter model, not a 14 billion parameter one. “You take the best pieces of the layers of each model and fuse them into one,” McQuade said. This technique allows Arcee to create models that possess the strengths of multiple specialized models while maintaining the efficiency and lower computational requirements of a smaller model.
The company’s approach allows users to set the weight and density of the fusion, controlling how much is taken from each input model. This flexibility enables the creation of highly tailored models that can outperform larger, more general models in specific domains.

Spectrum: Targeted training for faster, leaner models
Arcee’s Spectrum represents a significant advancement in the efficiency of language model training. This technique targets specific layer modules within the model based on their signal-to-noise ratio (SNR), while keeping others frozen. “Spectrum optimizes training time up to 42% and reduces catastrophic forgetting, without any performance degradation,” explained Lucas Atkins, Research Engineer at Arcee AI.
The importance of Spectrum lies in its ability to dramatically reduce the resources required for model training. Traditional methods often involve updating all parameters of a model during training, which can be computationally expensive and time-consuming. Spectrum’s selective approach allows for more efficient use of computational resources, potentially lowering the barriers to entry for organizations looking to develop custom AI models.
“We’ve built Spectrum into our training pipeline from the ground up, offering industry-leading training speed without sacrificing model quality,” Atkins added. This efficiency could enable faster iteration and more cost-effective model development for enterprises.
As performance gains in LLMs show signs of plateauing, the future of AI may increasingly lie in these more efficient, specialized models. McQuade said, “It no longer has to be one high ROI use case. You can do 10 use cases, 20 use cases, because we’re so efficient.” This shift towards SLMs could potentially democratize AI access across industries, making advanced AI capabilities more accessible and tailored to specific business needs.
Annual contracts in a usage-based world
McQuade emphasized, “Everything’s an annual contract, which is pretty unique in this space.” The pricing model is based on software licensing, moving away from the traditional usage-based pricing common in AI services. McQuade described it as “a software license” and mentioned that they also charge for inference.
Arcee AI offers its technology through two main product offerings:
- A set of tools for customers to train their own models
- Pre-trained models provided to customers using Arcee’s software
Arcee has two delivery methods:
- Arcee Cloud: A SaaS offering where customers can log in and train or merge models
- Arcee Enterprise: An offering deployable in the customer’s Virtual Private Cloud (VPC)
McQuade noted that the VPC option “really resonates with the larger companies.” The annual contract model allows for expansion. As McQuade put it, “if you want new models or whatever, then we expand you.” They also offer additional support and managed services for customers who want a more hands-off approach.
This pricing and sales model is designed to provide continuous value to customers while ensuring a steady, predictable revenue stream for Arcee AI. “We’re at $2 million in revenue now, and there’s a good chance we could turn profitable by early 2025,” McQuade revealed. The company, currently at 25 employees, plans to grow to about 50 within 18 months.
Arcee’s vision of efficient, customizable SLMs
The true power of SLMs lies not just in their domain specificity, but in empowering companies to experiment, learn, and optimize AI continuously. The ability to rapidly iterate and develop models at a lower cost could become the decisive factor in successful AI adoption.
AI development may begin to resemble a more iterative, experimental process, with companies treating their AI models as living systems that evolve and adapt. Agility could soon become as important as model performance. This mirrors the evolution of software development, where agile methodologies and continuous integration/continuous deployment (CI/CD) practices have now become standard.
A faster iteration cycle creates real competitive advantages. Companies using SLMs can swiftly adapt to changing user needs, refining models based on real-world feedback. Instead of putting all resources into one high-stakes AI implementation, companies can explore multiple use cases simultaneously, identifying the most impactful applications for their business without breaking the bank.
If Arcee is successful in delivering its vision of efficient, domain-specific small language models that can be rapidly iterated and customized, it could be well-positioned just at the right time when agility is becoming critical in AI development. This could transform the company into a highly valuable enterprise. The coming months will reveal whether small language models truly have an edge in the competitive AI landscape, potentially reshaping the industry’s approach to model development and deployment.
Author: James Thomason
Source: Venturebeat
Reviewed By: Editorial Team