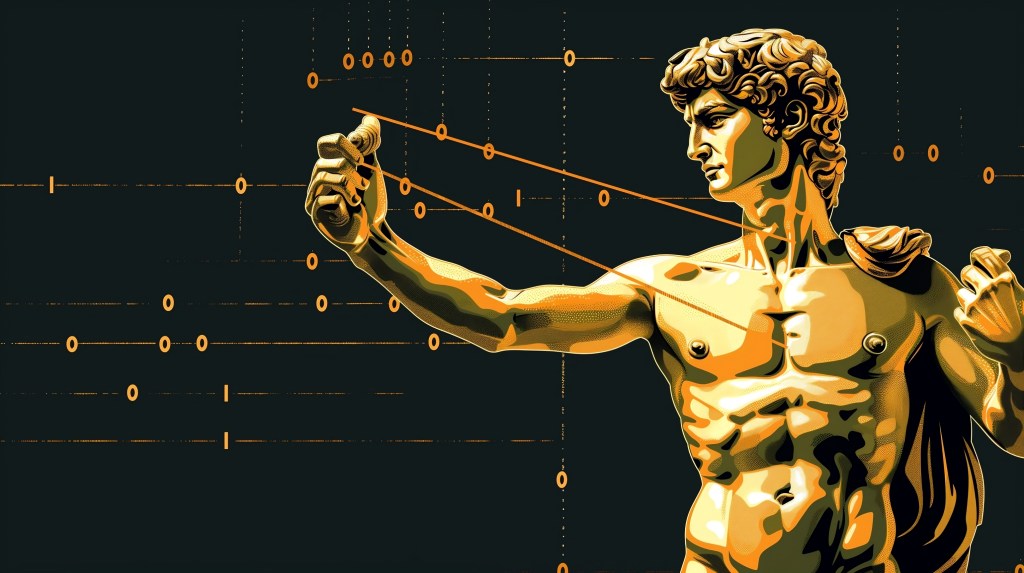
Salesforce has unveiled an AI model that punches well above its weight class, potentially reshaping the landscape of on-device artificial intelligence. The company’s new xLAM-1B model, dubbed the “Tiny Giant,” boasts just 1 billion parameters yet outperforms much larger models in function-calling tasks, including those from industry leaders OpenAI and Anthropic.
Meet Salesforce Einstein “Tiny Giant.” Our 1B parameter model xLAM-1B is now the best micro model for function calling, outperforming models 7x its size, including GPT-3.5 & Claude. On-device agentic AI is here. Congrats Salesforce Research!
Paper: https://t.co/SrntYvgxR5… pic.twitter.com/pPgIzk82xT
This David-versus-Goliath scenario in the AI world stems from Salesforce AI Research‘s innovative approach to data curation. The team developed APIGen, an automated pipeline that generates high-quality, diverse, and verifiable datasets for training AI models in function-calling applications.
“We demonstrate that models trained with our curated datasets, even with only 7B parameters, can achieve state-of-the-art performance on the Berkeley Function-Calling Benchmark, outperforming multiple GPT-4 models,” the researchers write in their paper. “Moreover, our 1B model achieves exceptional performance, surpassing GPT-3.5-Turbo and Claude-3 Haiku.”
This achievement is particularly noteworthy given the model’s compact size, which makes it suitable for on-device applications where larger models would be impractical. The implications for enterprise AI are significant, potentially allowing for more powerful and responsive AI assistants that can run locally on smartphones or other devices with limited computing resources.
The key to xLAM-1B’s performance lies in the quality and diversity of its training data. The APIGen pipeline leverages 3,673 executable APIs across 21 different categories, subjecting each data point to a rigorous three-stage verification process: format checking, actual function executions, and semantic verification.
This approach represents a significant shift in AI development strategy. While many companies have been racing to build ever-larger models, Salesforce’s method suggests that smarter data curation can lead to more efficient and effective AI systems. By focusing on data quality over model size, Salesforce has created a model that can perform complex tasks with far fewer parameters than its competitors.
The potential impact of this breakthrough extends beyond just Salesforce. By demonstrating that smaller, more efficient models can compete with larger ones, Salesforce is challenging the prevailing wisdom in the AI industry. This could lead to a new wave of research focused on optimizing AI models rather than simply making them bigger, potentially reducing the enormous computational resources currently required for advanced AI capabilities.
Moreover, the success of xLAM-1B could accelerate the development of on-device AI applications. Currently, many advanced AI features rely on cloud computing due to the size and complexity of the models involved. If smaller models like xLAM-1B can provide similar capabilities, it could enable more powerful AI assistants that run directly on users’ devices, improving response times and addressing privacy concerns associated with cloud-based AI.
The research team has made their dataset of 60,000 high-quality function-calling examples publicly available, a move that could accelerate progress in the field. “By making this dataset publicly available, we aim to benefit the research community and facilitate future work in this area,” the researchers explained.
Salesforce CEO Marc Benioff celebrated the achievement on Twitter, highlighting the potential for “on-device agentic AI.” This development could mark a major shift in the AI landscape, challenging the notion that bigger models are always better and opening new possibilities for AI applications in resource-constrained environments.
The implications of this breakthrough extend far beyond Salesforce’s immediate product lineup. As edge computing and IoT devices proliferate, the demand for powerful, on-device AI capabilities is set to skyrocket. xLAM-1B’s success could catalyze a new wave of AI development focused on creating hyper-efficient models tailored for specific tasks, rather than one-size-fits-all behemoths. This could lead to a more distributed AI ecosystem, where specialized models work in concert across a network of devices, potentially offering more robust, responsive, and privacy-preserving AI services.
Moreover, this development could democratize AI capabilities, allowing smaller companies and developers to create sophisticated AI applications without the need for massive computational resources. It may also address growing concerns about AI’s carbon footprint, as smaller models require significantly less energy to train and run.
As the industry digests the implications of Salesforce’s achievement, one thing is clear: in the world of AI, David has just proven he can not only compete with Goliath but potentially render him obsolete. The future of AI might not be in the cloud after all—it could be right in the palm of your hand.
We want to hear from you! Take our quick AI survey and share your insights on the current state of AI, how you’re implementing it, and what you expect to see in the future. Learn More
Salesforce has unveiled an AI model that punches well above its weight class, potentially reshaping the landscape of on-device artificial intelligence. The company’s new xLAM-1B model, dubbed the “Tiny Giant,” boasts just 1 billion parameters yet outperforms much larger models in function-calling tasks, including those from industry leaders OpenAI and Anthropic.
This David-versus-Goliath scenario in the AI world stems from Salesforce AI Research‘s innovative approach to data curation. The team developed APIGen, an automated pipeline that generates high-quality, diverse, and verifiable datasets for training AI models in function-calling applications.
“We demonstrate that models trained with our curated datasets, even with only 7B parameters, can achieve state-of-the-art performance on the Berkeley Function-Calling Benchmark, outperforming multiple GPT-4 models,” the researchers write in their paper. “Moreover, our 1B model achieves exceptional performance, surpassing GPT-3.5-Turbo and Claude-3 Haiku.”
Small but mighty: The power of efficient AI
This achievement is particularly noteworthy given the model’s compact size, which makes it suitable for on-device applications where larger models would be impractical. The implications for enterprise AI are significant, potentially allowing for more powerful and responsive AI assistants that can run locally on smartphones or other devices with limited computing resources.
Countdown to VB Transform 2024
Join enterprise leaders in San Francisco from July 9 to 11 for our flagship AI event. Connect with peers, explore the opportunities and challenges of Generative AI, and learn how to integrate AI applications into your industry. Register Now
The key to xLAM-1B’s performance lies in the quality and diversity of its training data. The APIGen pipeline leverages 3,673 executable APIs across 21 different categories, subjecting each data point to a rigorous three-stage verification process: format checking, actual function executions, and semantic verification.

This approach represents a significant shift in AI development strategy. While many companies have been racing to build ever-larger models, Salesforce’s method suggests that smarter data curation can lead to more efficient and effective AI systems. By focusing on data quality over model size, Salesforce has created a model that can perform complex tasks with far fewer parameters than its competitors.
Disrupting the AI status quo: A new era of research
The potential impact of this breakthrough extends beyond just Salesforce. By demonstrating that smaller, more efficient models can compete with larger ones, Salesforce is challenging the prevailing wisdom in the AI industry. This could lead to a new wave of research focused on optimizing AI models rather than simply making them bigger, potentially reducing the enormous computational resources currently required for advanced AI capabilities.
Moreover, the success of xLAM-1B could accelerate the development of on-device AI applications. Currently, many advanced AI features rely on cloud computing due to the size and complexity of the models involved. If smaller models like xLAM-1B can provide similar capabilities, it could enable more powerful AI assistants that run directly on users’ devices, improving response times and addressing privacy concerns associated with cloud-based AI.
The research team has made their dataset of 60,000 high-quality function-calling examples publicly available, a move that could accelerate progress in the field. “By making this dataset publicly available, we aim to benefit the research community and facilitate future work in this area,” the researchers explained.
Reimagining AI’s future: From cloud to device
Salesforce CEO Marc Benioff celebrated the achievement on Twitter, highlighting the potential for “on-device agentic AI.” This development could mark a major shift in the AI landscape, challenging the notion that bigger models are always better and opening new possibilities for AI applications in resource-constrained environments.
The implications of this breakthrough extend far beyond Salesforce’s immediate product lineup. As edge computing and IoT devices proliferate, the demand for powerful, on-device AI capabilities is set to skyrocket. xLAM-1B’s success could catalyze a new wave of AI development focused on creating hyper-efficient models tailored for specific tasks, rather than one-size-fits-all behemoths. This could lead to a more distributed AI ecosystem, where specialized models work in concert across a network of devices, potentially offering more robust, responsive, and privacy-preserving AI services.
Moreover, this development could democratize AI capabilities, allowing smaller companies and developers to create sophisticated AI applications without the need for massive computational resources. It may also address growing concerns about AI’s carbon footprint, as smaller models require significantly less energy to train and run.
As the industry digests the implications of Salesforce’s achievement, one thing is clear: in the world of AI, David has just proven he can not only compete with Goliath but potentially render him obsolete. The future of AI might not be in the cloud after all—it could be right in the palm of your hand.
Author: Michael Nuñez
Source: Venturebeat
Reviewed By: Editorial Team