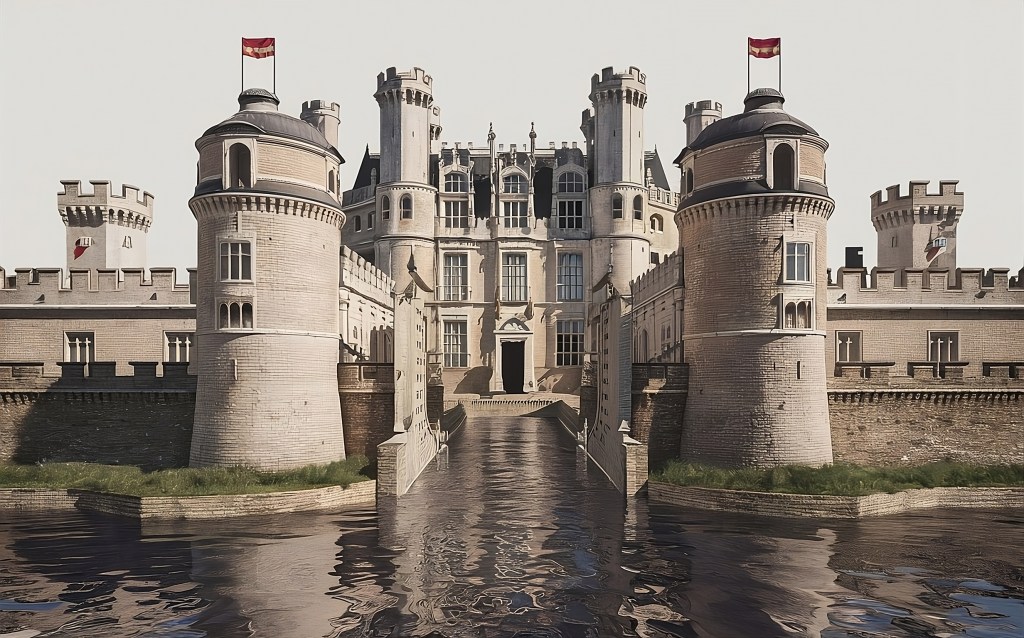
Research and development (R&D) is really a chimera — the mythological creature with two distinctive heads on one body.
Researchers have strong academic backgrounds and regularly publish papers, apply for patents and work on ideas that are likely to come to fruition over the course of years. Research departments deliver long-term value, discovering the future by asking tough questions and finding innovative answers.
Developers are valued (and hired) for their practical skills and problem solving abilities. Development teams work in rapid cycles focused on producing clear and measurable results. While critics of development teams claim they are simply packaging and repackaging products, it is actually the nuts and bolts of a product that drives adoption.
If R&D was a basketball team, the players would come from the development department. The research team would spend their time asking whether they can alter the rules of the game and whether basketball is even the best game for them to play.
June 5th: The AI Audit in NYC
Join us next week in NYC to engage with top executive leaders, delving into strategies for auditing AI models to ensure optimal performance and accuracy across your organization. Secure your attendance for this exclusive invite-only event.
The shift in AI barriers and value drivers
We’re seeing a shift in the AI space. Even as S&P or Fortune 500 companies are still focused on hiring AI researchers, the rules of the game are changing.
And as the rules change, the rest of the game (including players and tactics) is changing, too. Consider any large software company. Their core assets — those that they have spent millions of man-hours building and which are valued in billions on their financial statements — aren’t homes, buildings, factories or supply chains. Rather, they are enormous lumps of code that used to take decades to replicate. Not anymore. AI-powered auto coding is the equivalent of robots that build new homes in a few hours, at 1% of a home’s typical cost.
Suddenly, we’re seeing barriers to entry and value drivers have shifted dramatically. This means that the AI moat — the metaphoric barrier that protects a business from competition — has shifted, too.
Today, a long term and defensible business moat comes from the product, users and surrounding capabilities rather than research breakthroughs. The best sports teams in the world may have been those who came up with innovative strategies — but it is their community, brand and product offering that keeps them at the top of their league.
Where will AI dollars deliver good returns?
OpenAI, Google, Meta, Anthropic, Cohere, Mosaic Salesforce and at least a dozen others have hired, at enormous cost, large research teams to build better LLMs (large language models) — in other words, to figure out the new rules of the game. These invested dollars are arguably of crucial importance to society, yet netting patents and prizes does not ensure strong return on investment (ROI) for an AI startup.
Today, it is the development side, which turns new LLMs into products, that will make the difference. Whether it’s a new start-up building something that was once impossible, or a current company that integrates this new technology to offer something exceptional — long term and lasting value is being created by new AI capabilities in three core domains:
- Infrastructure for AI: As AI is adopted across organizations, companies need to adapt their infrastructure to accommodate evolving computational requirements. This starts with chips (dedicated or otherwise) and continues through the data network layers that allow AI data to flow throughout the organization. Similar to how Snowflake rose to deal with cloud computation, we envision others following a similar path in the organizational AI stack.
- Utility: We increasingly see a narrowing gap between LLMs learning and poaching talent from others. On the other hand, in large organizations, the challenge is not choosing best-of-breed tech, but applying this technology to specific use cases. Similar to Figma in front end design, we believe there is room for companies that allow many of the millions of coders who are not AI specialists to easily harness the benefits of LLMs.
- Vertically-focused LLM products: Naturally, when the rules of the game change, new products become possible. Similar to the way Uber could only work once smartphones were prolific, we imagine that creative founders will enhance our world with new products that beforehand were not possible.
The bottom line
The key to success in AI has moved from groundbreaking research to building practical applications. While research and development paves the way for future advancements, development translates those ideas into value.
The new AI moat lies in exceptional AI-powered products, not in groundbreaking research. Companies that excel in building user-friendly tools, infrastructure for smooth AI integration and entirely new LLM-powered products will be the future winners. As the focus shifts from defining the game’s rules to mastering them, the race is on to develop the most impactful applications of AI.
Author: Judah Taub, Hetz Ventures
Source: Venturebeat
Reviewed By: Editorial Team