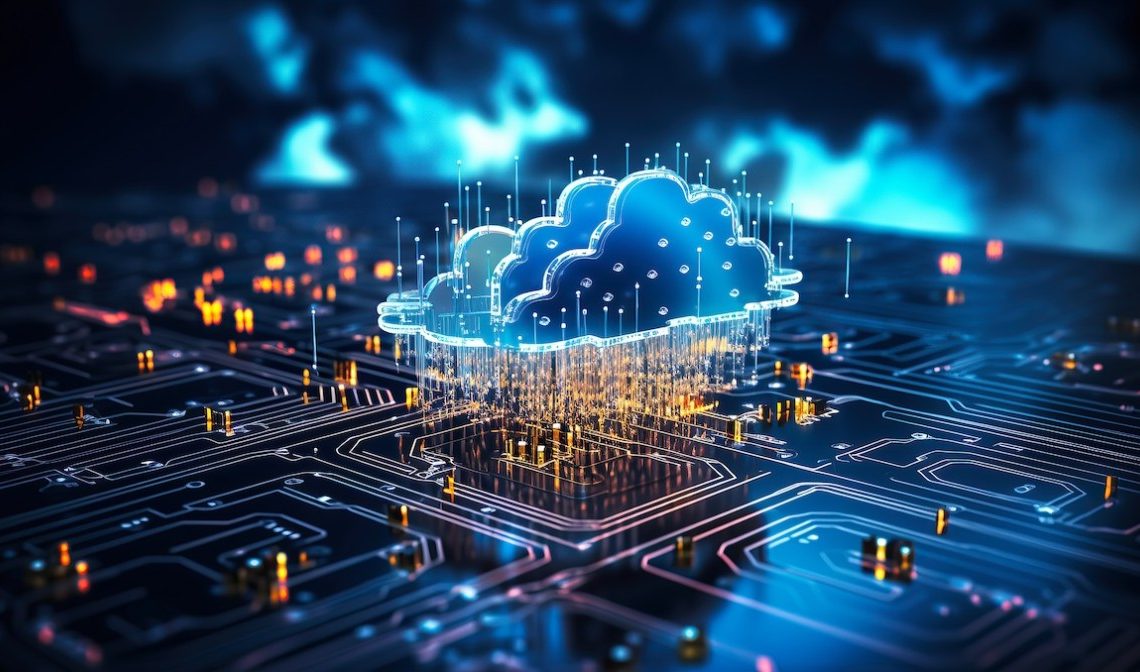
Generative AI offers real ROI — but also consumes a huge amount of compute and resources. In this VB Spotlight event, leaders from NVIDIA and Supermicro share how to identify critical use cases and the build the AI-ready platform that’s crucial for success.
Generative AI could add the equivalent of $2.6 trillion to $4.4 trillion annually across industries. But it’s also resource-hungry, consuming exponentially more compute, resources, networking and storage than any technology that’s come before. Accessing and processing data, customizing pre-trained models and running them optimally and at scale requires a complete AI-ready hardware and software stack, along with new technical expertise.
Anthony Larijani, senior product marketing manager at NVIDIA, and Yusuke Kondo, senior product marketing manager at Supermicro, spoke with Luis Ceze, co-founder and CEO of OctoML about how to determine where generative AI can benefit an organization, how to experiment with use cases, and the technology that’s critical to underpin a gen AI strategy.
Infrastructure decisions and workload considerations
Matching needs to infrastructure is the first major requirement, Larijani says.
“The way to go about this is to start with the end goal in mind,” he explains. “Try to visualize what you imagine this infrastructure will be used for, how you see the types of workloads running on it. If it’s along the lines of training a very large-scale foundational model, for instance, it’s going to have different computational requirements to delivering an inference application that needs to deliver real-time performance for a large number of users.”
That’s where scalability comes into it as well. Not only do you need to assess the model’s workload, you also have to anticipate the type of demand on the application you may be running. This overlaps with other considerations around the types of inference workloads you’ll be running, whether it’s a batch-type use case or a real-time use case, like a chatbot.
Cloud versus on-prem considerations
Gen AI applications usually require scale, and that means the cloud vs. on-prem consideration enters the conversation. Kondo explains that it clearly depends on the use case and scale required, but it’s still a critical, foundational decision.
“Using the cloud, obviously, you have more elasticity and coverage. When you need to scale up, you can just do it,” he says. “When you go with on-prem, you have to plan it out and predict how you’re going to scale before deciding on how much you need to invest in compute for yourself. That’s going to require a big initial cost.”
But generative AI also introduces a whole new level of data privacy considerations, especially when feeding data into a public API like ChatGPT, as well as control issues — do you want to control the workload end to end, or is just leveraging the API enough? And then of course there’s cost, which comes down to where you are in your generative AI journey — just starting out with some smaller experiments, or eager to start scaling.
Read More: Navigating Generative AI Use Cases Safely
“You have to judge the size of the project that you’re looking at. Does it make sense to just use the GPU cloud?” he says. “The cost is going down, that’s what we’re predicting, while compute ability just goes up. Does it make sense, looking at the current price of infrastructure to just use GPU cloud instances? Instead of spending a lot of capital on your own AI infrastructure, you might want to test it out using the GPU cloud.”
Open source versus proprietary models
There’s currently a trend toward smaller scale, more customized, specialized types of models for deployments across use cases within the enterprise, Larijani says. Thanks to techniques like retrieval augmented generation, efficient ways to take advantage of LLMs that can use proprietary data are emerging — and that directly impacts the choice of infrastructure. These specialized models involve fewer training requirements.
“Being able to only retrain a portion of that model that’s applicable to your use case reduces the training time and cost,” he explains. “It allows customers to reserve the types of resources that are either prohibitive from a cost standpoint for workloads that truly necessitate that type of performance, and allows them to take advantage of more cost-optimized solutions to run these types of workloads.”
How do you size the model for your needs, whether you’re open-source or proprietary?
“It comes down to fine-tuning the foundational models into a more specialized state, if you’re using open-source models,” Kondo says. “That’s going to affect the optimization for your cost and optimization for your infrastructure utilization of GPUs. You don’t want to waste what you invested in.”
Maximizing hardware with your software stack
Making the most of the hardware you choose also means a complex system software stack all the way down.
“It’s not just one level — there’s the rack scale and then the cluster-level implementation,” Kondo says. “When it comes to the large-scale infrastructure, obviously that’s way more complicated than just running an open-source model with one system. Often what we see is that we’re involving the NVIDIA subject matter experts from the early stages, even designing the racks, designing the cluster based on the software libraries and architecture that NVIDIA has put together. We design the racks based on their requirements, working closely with NVIDIA to establish the right solution for customers.”
Building a complete AI software stack is a complex and resource-intensive undertaking, Larijani adds, which is why NVIDIA has invested in becoming a full-stack computing company, from the infrastructure to the software that runs on top of it. For instance, the Nemo framework, which is part of the NVIDIA AI enterprise platform, offers an end-to-end solution to help customers build, customize and deploy an array of generative AI models and applications. It can help optimize the model training process and efficiently allocate GPU resources across tens of thousands of nodes. And once models are trained, it can customize them, adapting to a variety of tasks in specific domains.
“When an enterprise is ready to deploy this at scale, the Nemo framework integrates with the familiar tools that a lot of our customers have been using and are familiar with, like our Triton inference server,” he adds. “The optimized compiler to help our customers deploy efficiently with high throughput and low latency, it’s all done through that same familiar platform as well, and it’s all optimized to run perfectly on NVIDIA certified Supermicro systems.”
Future proofing against the growing complexity of LLMs
LLMs are getting bigger every day, Kondo says, and that growth doesn’t seem to be slowing down. The biggest issue is sustainability — and the power requirements of these servers are concerning.
“If you look at HGXH100, it’s 700 watts per GPU I believe. We’re expecting that that’s going to eventually hit 1000 watts per GPU,” he says. “When you compare this to 10 years ago, it’s nuts. How do we address that? That’s one of the reasons we’re working on our fully liquid-cooled integrated solution. In terms of the power usage, the liquid cooling infrastructure alone is going to save you more than 40 percent power usage. Green computing is one of our initiatives, and we truly believe that’s going to facilitate our innovation.”
On the parallel side, there are also continued efficiencies in terms of development of software to optimize deployments, whether it be for training models or serving inference to customers. New techniques that are emerging to help organizations take advantage of these capabilities in a cost-effective and sustainable way, Larijani says.
“Certainly, we see that there is an expanding need for more optimized, highly capable systems to train these types of models, but we’re seeing new methods of accessing and implementing them emerge,” he says. “As frequently as every week we see a new use case for AI. There’s certainly a host of interesting things happening in the space. We’ll be working toward optimizing and making them more efficient going forward from a software perspective as well.”
For more on how organizations can maximize their generative AI investments and build a tech stack positioned for success, don’t miss this VB Spotlight event!
Watch free on-demand here.
Agenda
- Identify use-cases for enterprise and what’s required for success
- How to leverage existing models and internal data for customized solutions
- How accelerated computing can improve time to results and business decision-making
- How to optimize your infrastructure and architecture for speed, cost and performance
- Which hardware and software solutions are right for your workloads
Presenters
- Yusuke Kondo, Senior Product Marketing Manager, Supermicro
- Anthony Larijani, Senior Product Marketing Manager, NVIDIA
- Luis Ceze, Co-founder & CEO, OctoML; Professor, University of Washington (Moderator)
Author: VB Staff
Source: Venturebeat
Reviewed By: Editorial Team