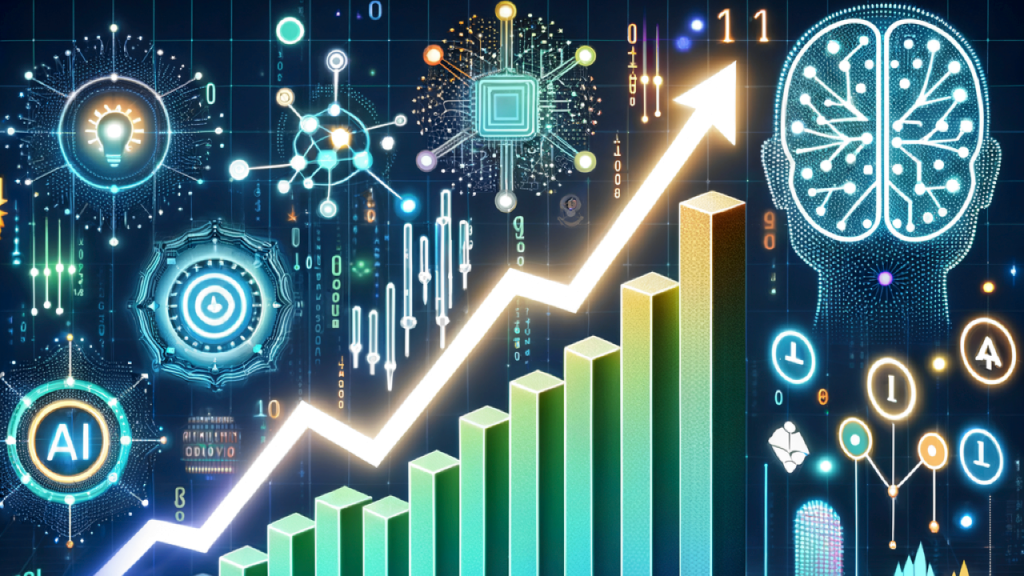
Innovation in machine learning and AI training continues to accelerate, even as more complex generative AI workloads come online.
Today MLCommons released the MLPerf 4.0 training benchmark, once again showing record levels of performance. The MLPerf training benchmark is a vendor neutral standard that enjoys broad industry participation. The MLPerf Training suite measures performance of full AI training systems across a range of workloads. Version 4.0 included over 205 results from 17 organizations. The new update is the first MLPerf training results release since MLPerf 3.1 training in November 2023.
The MLPerf 4.0 training benchmarks include results for image generation with Stable Diffusion and Large Language Model (LLM) training for GPT-3. With the MLPerf 4.0 training benchmarks are a number of first time results including a new LoRA benchmark that fine-tunes the Llama 2 70B large language model on document summarization using a parameter-efficient approach.
As is often the case with MLPerf results, when comparing even to just six months ago, there is significant gain.
VB Transform 2024 Registration is Open
Join enterprise leaders in San Francisco from July 9 to 11 for our flagship AI event. Connect with peers, explore the opportunities and challenges of Generative AI, and learn how to integrate AI applications into your industry. Register Now
“Even if you look at relative to the last cycle, some of our benchmarks have gotten nearly 2x better performance, in particular Stable Diffusion,” MLCommons founder and executive director David Kanter said in a press briefing. “So that’s pretty impressive in six months.”
The actual gain for Stable Diffusion training is 1.8x faster vs November 2023, while training for GPT-3 was up to 1.2x faster.
AI training performance isn’t just about hardware
There are many factors that go into training an AI model.
While hardware is important, so too is software as well as the network that connects clusters together.
“Particularly for AI training, we have access to many different lead levers to help improve performance and efficiency,” Kanter said. “For training, most of these systems are using multiple processors or accelerators and how the work is divided and communicated is absolutely critical.”
Kanter added that not only are vendors taking advantage of better silicon, they are also using better algorithms and better scaling to provide more performance over time.
Nvidia continues to scale training on Hopper
The big results in the MLPerf 4.0 training benchmarks all largely belong to Nvidia.
Across nine different tested workloads, Nvidia claims to have set new performance records on five of them. Perhaps most impressively is that the new records were mostly set using the same core hardware platforms Nvidia used a year ago in June 2023.
In a press briefing David Salvator, director of AI at Nvidia, commented that the Nvidia H100 Hopper architecture continues to deliver value.
“Throughout Nvidia’s history with deep learning in any given generation of product we will typically get two to 2.5x more performance out of an architecture, from software innovation over the course of the life of that particular product,” Salvator said.
For the H100, Nvidia used numerous techniques to improve performance for MLPerf 4.0 training. The various techniques include full stack optimization, highly tuned FP8 kernels, FP8-aware distributed optimizer, optimized cuDNN FlashAttention, improved math and comms execution overlap as well as intelligent GPU power allocation.
Why the MLPerf training benchmarks matter to the enterprise
Aside from providing organizations with standardized benchmarks on training performance, there is more value that the actual numbers provide.
While performance keeps on getting better all the time, Salvator emphasized that it’s getting better also with the same hardware.
Salvator noted that the results are a quantitative demonstration that shows how Nvidia is able to deliver new value on top of existing architectures. As organizations are considering building out new deployments, particularly on-premises, he said they are essentially make a big bet on a technology platform. The fact that an organization can get growing benefits for years after an initial technology debut is important.
“In terms of why we care so much about performance, the simple answer is because for businesses, it drives return on investment,” he said.
Author: Sean Michael Kerner
Source: Venturebeat
Reviewed By: Editorial Team