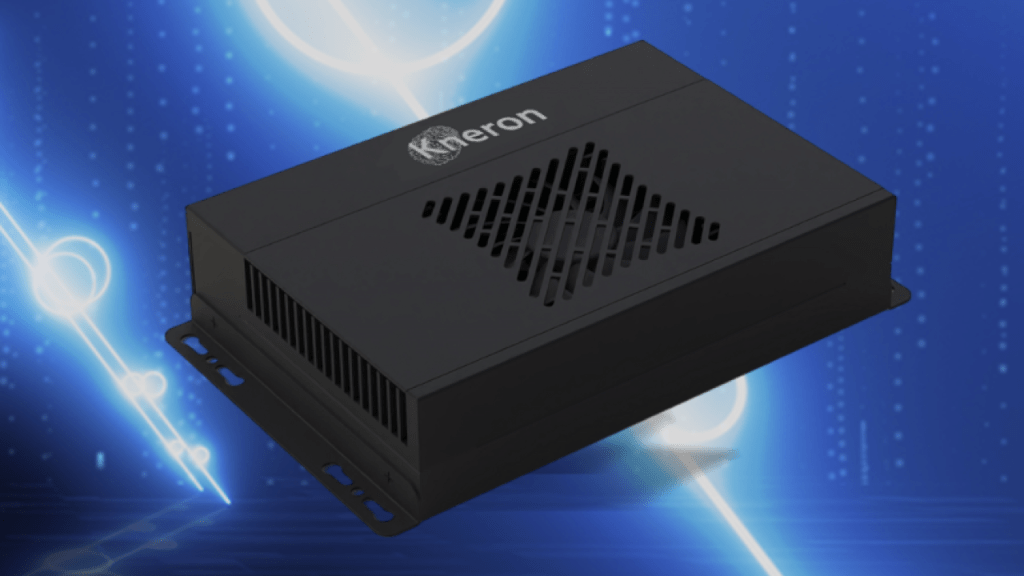
There’s more than one way to handle AI fine tuning, training and inference at the edge.
Among the options beyond just a GPU is using a neural processing unit (NPU), from silicon vendor Kneron.
At the Computex conference in Taiwan today, Kneron detailed its next generation of silicon and server technology to help advance edge AI inference as well as fine tuning. Kneron got its start back in 2015 and includes Qualcomm as well as Sequoia Capital among its investors. In 2023 the company announced its KL730 NPU in a bid to help address the global shortage of GPUs. Now Kneron is rolling out its next generation KL830 and providing a glimpse into the future KL 1140 which is set to debut in 2025. Beyond just new NPU silicon, Kneron is also growing its AI server portfolio with the KNEO 330 Edge GPT server that enables offline inference capabilities.
Kneron’s technology is part of a small but growing number of vendors that includes Groq and SambaNova among others that are looking to use a technology other than a GPU, to help improve power and efficiency of AI workloads.
A growing focus for Kneron with its update is to enable private GPT servers that can run on-premises.
Rather than an organization needing to rely on a large system that has cloud connectivity, a private GPT server can run locally at the edge of a network for inference. That’s the promise of the Kneron KNEO system.
Kneron CEO Albert Liu explained to VentureBeat that the KNEO 330 system integrates multiple KL830 edge AI chips and is a small form factor server. The promise of the system according to Liu is that it allows for affordable on-premises GPT deployments for enterprises. The predecessor KNEO 300 system which is powered by the KL730 is already in use with large organizations including Stanford University in California.
The KL830 chip, which falls between the company’s previous KL730 and the upcoming KL1140, is specifically designed for language models. It can be cascaded to support larger models while maintaining low power consumption.
While hardware is a core focus for Kneron, software is also part of the mix.
Kneron now has multiple capabilities for training and fine-tuning models that run on top of the company’s hardware. Liu said that Kneron is combining multiple open models and then fine tuning them to run on NPUs.
Kneron now also supports transferring trained models onto their chips via a neural compiler. This tool allows users to dump models trained with frameworks like TensorFlow, Caffe or MXNet and compile them for use on Kneron chips.
Kneron’s new hardware can also be used to help support RAG retrieval-augmented generation (RAG) workflows. Liu noted that to reduce memory needs for large vector databases required by RAG, Kneron’s chips use a unique structure compared to GPUs. This allows RAG to function with lower memory and power consumption.
One of the key differentiators for Kneron’s technology is its low power consumption.
“I think the main difference is our power consumption is so low,” Liu said.
According to Kneron its new KL830 has a peak power consumption of only a paltry 2 watts. Even with that low level of power consumption the company claims that the KL830 provides consolidated calculation power (CCP) of up to 10eTOPS@8bit.
Liu said that the low power consumption allows Kneron’s chips to be integrated into various devices, including PCs, without the need for additional cooling solutions.
Time’s almost up! There’s only one week left to request an invite to The AI Impact Tour on June 5th. Don’t miss out on this incredible opportunity to explore various methods for auditing AI models. Find out how you can attend here.
There’s more than one way to handle AI fine tuning, training and inference at the edge.
Among the options beyond just a GPU is using a neural processing unit (NPU), from silicon vendor Kneron.
At the Computex conference in Taiwan today, Kneron detailed its next generation of silicon and server technology to help advance edge AI inference as well as fine tuning. Kneron got its start back in 2015 and includes Qualcomm as well as Sequoia Capital among its investors. In 2023 the company announced its KL730 NPU in a bid to help address the global shortage of GPUs. Now Kneron is rolling out its next generation KL830 and providing a glimpse into the future KL 1140 which is set to debut in 2025. Beyond just new NPU silicon, Kneron is also growing its AI server portfolio with the KNEO 330 Edge GPT server that enables offline inference capabilities.
Kneron’s technology is part of a small but growing number of vendors that includes Groq and SambaNova among others that are looking to use a technology other than a GPU, to help improve power and efficiency of AI workloads.
June 5th: The AI Audit in NYC
Join us next week in NYC to engage with top executive leaders, delving into strategies for auditing AI models to ensure optimal performance and accuracy across your organization. Secure your attendance for this exclusive invite-only event.
Edge AI and Private LLMs powered by NPUs
A growing focus for Kneron with its update is to enable private GPT servers that can run on-premises.
Rather than an organization needing to rely on a large system that has cloud connectivity, a private GPT server can run locally at the edge of a network for inference. That’s the promise of the Kneron KNEO system.
Kneron CEO Albert Liu explained to VentureBeat that the KNEO 330 system integrates multiple KL830 edge AI chips and is a small form factor server. The promise of the system according to Liu is that it allows for affordable on-premises GPT deployments for enterprises. The predecessor KNEO 300 system which is powered by the KL730 is already in use with large organizations including Stanford University in California.
The KL830 chip, which falls between the company’s previous KL730 and the upcoming KL1140, is specifically designed for language models. It can be cascaded to support larger models while maintaining low power consumption.
Kneron bringing new tools for Edge AI training and fine tuning
While hardware is a core focus for Kneron, software is also part of the mix.
Kneron now has multiple capabilities for training and fine-tuning models that run on top of the company’s hardware. Liu said that Kneron is combining multiple open models and then fine tuning them to run on NPUs.
Kneron now also supports transferring trained models onto their chips via a neural compiler. This tool allows users to dump models trained with frameworks like TensorFlow, Caffe or MXNet and compile them for use on Kneron chips.
Kneron’s new hardware can also be used to help support RAG retrieval-augmented generation (RAG) workflows. Liu noted that to reduce memory needs for large vector databases required by RAG, Kneron’s chips use a unique structure compared to GPUs. This allows RAG to function with lower memory and power consumption.
Kneron’s secret sauce: low power consumption
One of the key differentiators for Kneron’s technology is its low power consumption.
“I think the main difference is our power consumption is so low,” Liu said.
According to Kneron its new KL830 has a peak power consumption of only a paltry 2 watts. Even with that low level of power consumption the company claims that the KL830 provides consolidated calculation power (CCP) of up to 10eTOPS@8bit.
Liu said that the low power consumption allows Kneron’s chips to be integrated into various devices, including PCs, without the need for additional cooling solutions.
Author: Sean Michael Kerner
Source: Venturebeat
Reviewed By: Editorial Team