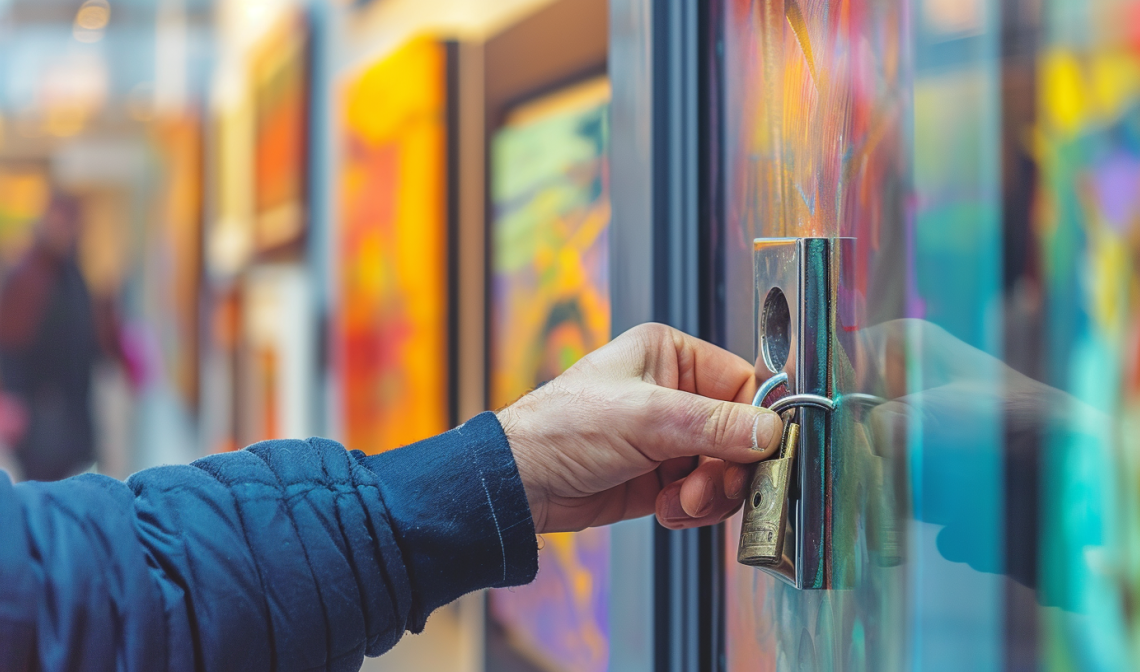
As the generative AI era has ushered in a wave of image generation models trained on data scraped from other artists across the internet, some artists who object to this practice have sought ways to defend their work from AI. (Full disclosure: VentureBeat uses AI art generation tools to create header art for articles, including this one.)
Now there’s a new tool on the block promising artists a defense not only for one image at a time, but their entire portfolio of work (or as many images as they’d like to upload to the web).
The new tool, Kin.art, is actually part of a new online art hosting platform of the same name that promises fast, easily accessible built-in defenses from AI whenever an artist uploads one or more of their images to its servers.
Announced today by its co-founder and chief technology officer Flor Ronsmans De Vry, Kin.art’s AI defensive method differs from others previously fielded by other companies and researchers, such as the University of Chicago Glaze Project team, which last year launched Glaze — free downloadable tool for artists that sought to protect their unique style — and followed it up just last week with Nightshade, a tool that “poisons” AI models by subtly altering pixels in an artwork to confuse the model into learning the wrong names and forms for objects contained therein.
For one thing, it uses a different machine learning technique — a pair of them, in fact. More on this in the next section. For another, it promises to be much faster than other rivals, taking only “milliseconds” to apply the defense to a given image.
“You can think of Kin.art as the first line of defense for your artwork,” Ronsmans De Vry said in a press release emailed to VentureBeat ahead of the launch. “While other tools such as Nightshade and Glaze try to mitigate the damage from your artwork already being included in a dataset, Kin.art prevents it from happening to begin with.”
Ronsmans De Vry and much of the founding team of Kin.art were previously behind Curious Addys Trading Club, an NFT artwork collection and platform for users to generate their own NFT art collections.
According to Ronsmans De Vry, Kin.art’s defense mechanism for artists against AI works on two fronts: the first, image segmentation, is a longstanding technique that uses machine learning (ML) algorithms to break apart the artist’s image into smaller pieces and then analyzes what is contained within each segment.
In this case, the technique is used to “scramble” the image for an algorithms that may wish to scrape it, so that it looks disordered to a machine’s eye, but looks the same as the artist intended to the human eye. Except, if the image is downloaded or saved without authorization — it too will appear to have an additional layer of scrambling atop it.
The other front, “label fuzzing,” scrambles the label associated with the image, such as its title or description or other metadata and text attached to it.
Typically, AI training algorithms rely on pairs of both images and text metadata in order to train, learning that a furry creature with four legs, a tail, and a snout tends to be a dog, for example.
By disrupting either the image composition itself or the label, and offering scrambled versions of both, Kin.art seeks to make it technically impossible for AI training algorithms to accurately learn what is in any images that their creators scrape and feed to them, and thereby discard the data and not put it into the model in the first place.
“This dual approach guarantees that artists who showcase their portfolios on Kin.art are fully shielded from unauthorized AI training of their work,” Ronsmans De Vry stated in Kin.art’s press release.
Like the rival tools from the University of Chicago Glaze Project team, Kin.art is free for artists to use: they simply need to create an account on the Kin.art website and upload their works. There, they will have the option to turn AI protection on or off for any works they choose.
How does Kin.art plan to make money then? Simple: by attaching a “low fee” to any artworks that are sold or monetized using e-commerce features already built into its online platform, such as custom commission-based works.
“In the future, we’ll charge a low fee on top of any commission processed by our platform to fuel our growth and allow us to keep building products for the people we care about,” Ronsmans De Vry stated in a follow-up email to VentureBeat.
VentureBeat had the opportunity to email a set of questions to Ronsmans De Vry ahead of the announcement of Kin.art’s platform today that go into greater detail about the company’s approach, tech, and even the origin of its name. Here is the creator’s answers, edited and condensed for clarity.
VentureBeat: How did you come up with the idea to pair image segmentation with label fuzzing to prevent AI databases from ingesting artists’ works hosted on the Kin.art platform?
Ronsmans De Vry: Our journey with Kin.art began last year when we tried to commission an art piece for a friend’s birthday. We posted our commission request on an online forum and were quickly flooded by hundreds of replies, with no way to manage them. We spent hours on hours going through them, following up, asking for portfolios, and requesting quotes. As both engineers and art enthusiasts, we thought there had to be a better way, so we set out to build one.
This was around the time when image generation models started becoming scarily capable. Because we were following the progress so closely, it didn’t take long for us to catch wind of the infringements on artists’ rights that went into the training of these models. Faced with this new issue, we decided to put our heads together once again to try and figure out a way to help these artists.
While digging deeper into the training process of these new generative models, we were happy to discover that the damage done was not irreversible. It turned out that the most popular dataset for training generative models, Common Crawl, did not include the actual image files due to size constraints. This meant that not all hope was lost and that we could help artists whose art was included without permission by disrupting the images.
At the time, there were a few teams already working on this problem. We chose to target a different stage of AI training from most of them, playing into prevention by ensuring that the image-label pairs are never inserted correctly in the first place.
This approach led us to the techniques we ended up settling on, which seemed like a natural fit for the problem for us. We decided to disrupt both inputs, rather than just targeting the image or the label independently.
Is this solution applied uniquely to each image — or do all images get the same segmentation and fuzzing treatment?
Great question! All images go through the same segmentation/fuzzing pipeline, but not all of them come out with the same mutations. We’ve implemented some additional parameters internally which we’re currently experimenting with to find the perfect balance between the level of protection and user-friendliness. In the future, we might make the level of protection your artwork receives configurable for our power users.
How long does the segmentation and fuzzing process take on each image?
The process only takes a few hundred milliseconds and is done on our servers as soon as the image is uploaded. By the time your artwork is uploaded most of the work has already been done, meaning that there’s no waiting around later.
How does the image segmentation and label fuzzing appear to ordinary web users who wish to view the artwork on the portfolios?
As a visitor, you’ll almost never notice that the protection layer is there. We’ve done our best to make the experience as seamless as possible, with the only way to tell being when you try to download an image. Important to note is that we allow artists to opt out of the protection, so if they want their users to be able to freely download their images they can.
Do artists have the option to turn off these anti-AI features on Kin.art? If so, how? If not, why not?
When uploading art to the platform, users will have the option to opt out of the protection through a simple toggle. We recognize that everyone has a different level of comfort with their data being used for things like AI training, so we welcome users to enable/disable the protection as they please.
How much does the Kin.art platform cost artists who use it?
Anyone will be able to use the portfolio platform and its AI protection features completely free of charge and we don’t intend to ever monetize these features.
How many users are currently using Kin.art to host their art portfolios and will the automatically have the new AI defenses applied to their current work hosted on Kin.art?
This is such an amazing question! We worked with a select few artists to develop the platform and are announcing it to the public tomorrow for the first time ever, so we don’t have a substantial number of portfolios already created. We respect the preferences of our community a lot, so we didn’t want to forcibly migrate them to use our protection. They’ll have the option to re-upload their work to enable the AI protection features and we’ll be introducing a feature to make this easier by including the option in the edit window.
Where did the name Kin.art come from?
This is one I really wanted someone to ask, thanks! We chose the name Kin.art based on both the English and Japanese meanings of the word. In English, kin refers to family, while in Japense, kin can be interpreted as gold. With our goal being creating a community of thriving artists, we thought it was a perfect fit.
How does Kin.art make money/monetize?
We won’t be charging anything while we refine our product in its beta phase and even beyond that, our portfolio and AI protection features will remain free for anyone to use. In the future, we’ll charge a low fee on top of any commission processed by our platform to fuel our growth and allow us to keep building products for the people we care about.
Does Kin.art allow AI artists to upload their works to the platform and benefit from the new AI defense tools? Why or why not?
As much as we would prefer to keep the art landscape as it was, it’s unlikely that AI is going anywhere. The best we can do as a community is to create a way for both human and non-human art to co-exist, with both of them being clearly labeled to avoid any misrepresentation. While we work towards a solution, we take a neutral stance on this and allow generative artists to share their art on our platform when it is labeled as such. We recognize that there are people who have learned to harness AI in unexpected ways to create amazing work that was not possible before, but take issue with the ethical concerns surrounding the training data of these models.
Why would someone use Kin.art over Nightshade, which is free and user-controlled, and could be applied to an artwork hosted on any website? Your release notes that “Unlike previous solutions that assume artwork has already been added to a dataset and attempt to poison the dataset after the fact, Kin.art prevents artists’ work from successfully being entered into a dataset in the first place.”
Yet Nightshade itself also allows artists to apply a shade before uploading their work to the web, which would prevent their work from being accurately scraped and trained on. While it is true that Nightshade still enables AI models to scrape, the point is that the scraped material would not accurately reflect the artwork and cause the model to mislearn what it has trained on.
Thanks for bringing up Nightshade/Glaze! We love what the team at uChicago has built and encourage anyone to help us tackle this problem.
We believe prevention is always the most important thing to strive for, as not having your data included in the first place is the safest position you can be in.
We have a lot of respect for the team behind Nightshade and there’s no doubt that they’ve done some amazing research, but mutating images to poison datasets at scale remains extremely expensive.
For context: I just downloaded the recently released version of Nightshade and after downloading 5GB+ of dependencies it looks like shading one image on default settings will take anywhere from 30-180 minutes on an M1 Pro device.
We hope to see this change in the future, but for now, the poisoning approach does not seem viable at scale. Because we target different stages of the AI learning process, however, artists who have the means to run utilize Nightshade can use it together with our platform for added protection.
I see that the Kin.art website contains a list of press mentions in the middle (screenshot attached), with logos for Wired, Elle, Forbes, PBS, and Nas Daily. I searched for your name and Kin.art on several of these websites but did not find any articles about you, Kin.art, or Curious Addys (which I gather is your previous project) on these publications. Do you have links to the prior press coverage you can send me?
Those media platforms have all covered our co-founder team before so we decided to include them on our homepage, I’ve included links to most of them below.
Forbes
Wired Japan
PBS SoCal
Nas Daily
As the generative AI era has ushered in a wave of image generation models trained on data scraped from other artists across the internet, some artists who object to this practice have sought ways to defend their work from AI. (Full disclosure: VentureBeat uses AI art generation tools to create header art for articles, including this one.)
Now there’s a new tool on the block promising artists a defense not only for one image at a time, but their entire portfolio of work (or as many images as they’d like to upload to the web).
The new tool, Kin.art, is actually part of a new online art hosting platform of the same name that promises fast, easily accessible built-in defenses from AI whenever an artist uploads one or more of their images to its servers.
Announced today by its co-founder and chief technology officer Flor Ronsmans De Vry, Kin.art’s AI defensive method differs from others previously fielded by other companies and researchers, such as the University of Chicago Glaze Project team, which last year launched Glaze — free downloadable tool for artists that sought to protect their unique style — and followed it up just last week with Nightshade, a tool that “poisons” AI models by subtly altering pixels in an artwork to confuse the model into learning the wrong names and forms for objects contained therein.
For one thing, it uses a different machine learning technique — a pair of them, in fact. More on this in the next section. For another, it promises to be much faster than other rivals, taking only “milliseconds” to apply the defense to a given image.
“You can think of Kin.art as the first line of defense for your artwork,” Ronsmans De Vry said in a press release emailed to VentureBeat ahead of the launch. “While other tools such as Nightshade and Glaze try to mitigate the damage from your artwork already being included in a dataset, Kin.art prevents it from happening to begin with.”
Ronsmans De Vry and much of the founding team of Kin.art were previously behind Curious Addys Trading Club, an NFT artwork collection and platform for users to generate their own NFT art collections.
How Kin.art works and differs from other AI art defense mechanisms
According to Ronsmans De Vry, Kin.art’s defense mechanism for artists against AI works on two fronts: the first, image segmentation, is a longstanding technique that uses machine learning (ML) algorithms to break apart the artist’s image into smaller pieces and then analyzes what is contained within each segment.
In this case, the technique is used to “scramble” the image for an algorithms that may wish to scrape it, so that it looks disordered to a machine’s eye, but looks the same as the artist intended to the human eye. Except, if the image is downloaded or saved without authorization — it too will appear to have an additional layer of scrambling atop it.
The other front, “label fuzzing,” scrambles the label associated with the image, such as its title or description or other metadata and text attached to it.
Typically, AI training algorithms rely on pairs of both images and text metadata in order to train, learning that a furry creature with four legs, a tail, and a snout tends to be a dog, for example.
By disrupting either the image composition itself or the label, and offering scrambled versions of both, Kin.art seeks to make it technically impossible for AI training algorithms to accurately learn what is in any images that their creators scrape and feed to them, and thereby discard the data and not put it into the model in the first place.
“This dual approach guarantees that artists who showcase their portfolios on Kin.art are fully shielded from unauthorized AI training of their work,” Ronsmans De Vry stated in Kin.art’s press release.
Free to use
Like the rival tools from the University of Chicago Glaze Project team, Kin.art is free for artists to use: they simply need to create an account on the Kin.art website and upload their works. There, they will have the option to turn AI protection on or off for any works they choose.
How does Kin.art plan to make money then? Simple: by attaching a “low fee” to any artworks that are sold or monetized using e-commerce features already built into its online platform, such as custom commission-based works.
“In the future, we’ll charge a low fee on top of any commission processed by our platform to fuel our growth and allow us to keep building products for the people we care about,” Ronsmans De Vry stated in a follow-up email to VentureBeat.
A brief QA with creator Ronsmans De Vry
VentureBeat had the opportunity to email a set of questions to Ronsmans De Vry ahead of the announcement of Kin.art’s platform today that go into greater detail about the company’s approach, tech, and even the origin of its name. Here is the creator’s answers, edited and condensed for clarity.
VentureBeat: How did you come up with the idea to pair image segmentation with label fuzzing to prevent AI databases from ingesting artists’ works hosted on the Kin.art platform?
Ronsmans De Vry: Our journey with Kin.art began last year when we tried to commission an art piece for a friend’s birthday. We posted our commission request on an online forum and were quickly flooded by hundreds of replies, with no way to manage them. We spent hours on hours going through them, following up, asking for portfolios, and requesting quotes. As both engineers and art enthusiasts, we thought there had to be a better way, so we set out to build one.
This was around the time when image generation models started becoming scarily capable. Because we were following the progress so closely, it didn’t take long for us to catch wind of the infringements on artists’ rights that went into the training of these models. Faced with this new issue, we decided to put our heads together once again to try and figure out a way to help these artists.
While digging deeper into the training process of these new generative models, we were happy to discover that the damage done was not irreversible. It turned out that the most popular dataset for training generative models, Common Crawl, did not include the actual image files due to size constraints. This meant that not all hope was lost and that we could help artists whose art was included without permission by disrupting the images.
At the time, there were a few teams already working on this problem. We chose to target a different stage of AI training from most of them, playing into prevention by ensuring that the image-label pairs are never inserted correctly in the first place.
This approach led us to the techniques we ended up settling on, which seemed like a natural fit for the problem for us. We decided to disrupt both inputs, rather than just targeting the image or the label independently.
Is this solution applied uniquely to each image — or do all images get the same segmentation and fuzzing treatment?
Great question! All images go through the same segmentation/fuzzing pipeline, but not all of them come out with the same mutations. We’ve implemented some additional parameters internally which we’re currently experimenting with to find the perfect balance between the level of protection and user-friendliness. In the future, we might make the level of protection your artwork receives configurable for our power users.
How long does the segmentation and fuzzing process take on each image?
The process only takes a few hundred milliseconds and is done on our servers as soon as the image is uploaded. By the time your artwork is uploaded most of the work has already been done, meaning that there’s no waiting around later.
How does the image segmentation and label fuzzing appear to ordinary web users who wish to view the artwork on the portfolios?
As a visitor, you’ll almost never notice that the protection layer is there. We’ve done our best to make the experience as seamless as possible, with the only way to tell being when you try to download an image. Important to note is that we allow artists to opt out of the protection, so if they want their users to be able to freely download their images they can.
Do artists have the option to turn off these anti-AI features on Kin.art? If so, how? If not, why not?
When uploading art to the platform, users will have the option to opt out of the protection through a simple toggle. We recognize that everyone has a different level of comfort with their data being used for things like AI training, so we welcome users to enable/disable the protection as they please.
How much does the Kin.art platform cost artists who use it?
Anyone will be able to use the portfolio platform and its AI protection features completely free of charge and we don’t intend to ever monetize these features.
How many users are currently using Kin.art to host their art portfolios and will the automatically have the new AI defenses applied to their current work hosted on Kin.art?
This is such an amazing question! We worked with a select few artists to develop the platform and are announcing it to the public tomorrow for the first time ever, so we don’t have a substantial number of portfolios already created. We respect the preferences of our community a lot, so we didn’t want to forcibly migrate them to use our protection. They’ll have the option to re-upload their work to enable the AI protection features and we’ll be introducing a feature to make this easier by including the option in the edit window.
Where did the name Kin.art come from?
This is one I really wanted someone to ask, thanks! We chose the name Kin.art based on both the English and Japanese meanings of the word. In English, kin refers to family, while in Japense, kin can be interpreted as gold. With our goal being creating a community of thriving artists, we thought it was a perfect fit.
How does Kin.art make money/monetize?
We won’t be charging anything while we refine our product in its beta phase and even beyond that, our portfolio and AI protection features will remain free for anyone to use. In the future, we’ll charge a low fee on top of any commission processed by our platform to fuel our growth and allow us to keep building products for the people we care about.
Does Kin.art allow AI artists to upload their works to the platform and benefit from the new AI defense tools? Why or why not?
As much as we would prefer to keep the art landscape as it was, it’s unlikely that AI is going anywhere. The best we can do as a community is to create a way for both human and non-human art to co-exist, with both of them being clearly labeled to avoid any misrepresentation. While we work towards a solution, we take a neutral stance on this and allow generative artists to share their art on our platform when it is labeled as such. We recognize that there are people who have learned to harness AI in unexpected ways to create amazing work that was not possible before, but take issue with the ethical concerns surrounding the training data of these models.
Why would someone use Kin.art over Nightshade, which is free and user-controlled, and could be applied to an artwork hosted on any website? Your release notes that “Unlike previous solutions that assume artwork has already been added to a dataset and attempt to poison the dataset after the fact, Kin.art prevents artists’ work from successfully being entered into a dataset in the first place.”
Yet Nightshade itself also allows artists to apply a shade before uploading their work to the web, which would prevent their work from being accurately scraped and trained on. While it is true that Nightshade still enables AI models to scrape, the point is that the scraped material would not accurately reflect the artwork and cause the model to mislearn what it has trained on.
Thanks for bringing up Nightshade/Glaze! We love what the team at uChicago has built and encourage anyone to help us tackle this problem.
We believe prevention is always the most important thing to strive for, as not having your data included in the first place is the safest position you can be in.
We have a lot of respect for the team behind Nightshade and there’s no doubt that they’ve done some amazing research, but mutating images to poison datasets at scale remains extremely expensive.
For context: I just downloaded the recently released version of Nightshade and after downloading 5GB+ of dependencies it looks like shading one image on default settings will take anywhere from 30-180 minutes on an M1 Pro device.
We hope to see this change in the future, but for now, the poisoning approach does not seem viable at scale. Because we target different stages of the AI learning process, however, artists who have the means to run utilize Nightshade can use it together with our platform for added protection.
I see that the Kin.art website contains a list of press mentions in the middle (screenshot attached), with logos for Wired, Elle, Forbes, PBS, and Nas Daily. I searched for your name and Kin.art on several of these websites but did not find any articles about you, Kin.art, or Curious Addys (which I gather is your previous project) on these publications. Do you have links to the prior press coverage you can send me?
Those media platforms have all covered our co-founder team before so we decided to include them on our homepage, I’ve included links to most of them below.
Forbes
Wired Japan
PBS SoCal
Nas Daily
VentureBeat’s mission is to be a digital town square for technical decision-makers to gain knowledge about transformative enterprise technology and transact. Discover our Briefings.
Author: Carl Franzen
Source: Venturebeat
Reviewed By: Editorial Team