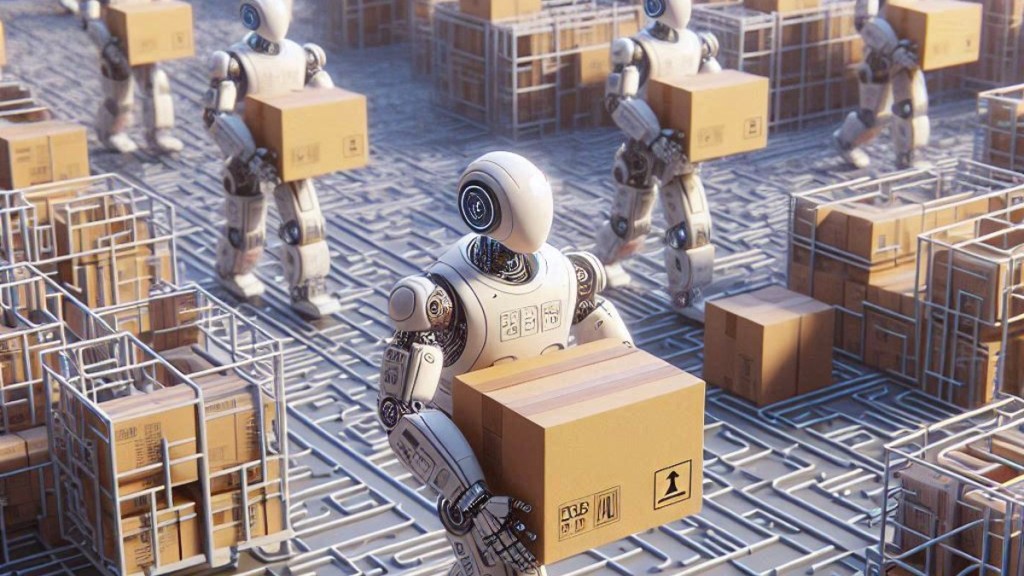
Foundation models have revolutionized the fields of computer vision and natural language processing. Now, a group of researchers believe the same principles can be applied to create foundation agents, AI systems that can perform open-ended decision-making tasks in the physical world.
In a new position paper, researchers at the University of Chinese Academy of Sciences describe foundation agents as “generally capable agents across physical and virtual worlds” that will be “the paradigm shift for decision making, akin to[large language models] LLMs as general-purpose language models to solve linguistic and knowledge-based tasks.”
Foundation agents will make it easier to create versatile AI systems for the real world and can have a great impact on fields that rely on brittle and task-specific AI systems.
Traditional approaches to AI decision-making have several shortcomings. Expert systems heavily rely on formalized human knowledge and manually crafted rules. Reinforcement learning systems (RL), which have become more popular in recent years, must be trained from scratch for every new task, which makes them sample-inefficient and limits their ability to generalize to new environments. Imitation learning (IL), where the AI learns decision-making from human demonstrations also requires extensive human efforts to craft training examples and action sequences.
In contrast, LLMs and vision language models (VLMs) can rapidly adapt to various tasks with minimal fine-tuning or prompting. The researchers believe that, with some adjustments, the same approach can be used to create foundation agents that can handle open-ended decision-making tasks in the physical and virtual worlds.
Some of the key characteristics of foundation models can help create foundation agents for the real world. First, LLMs can be pre-trained on large unlabeled datasets from the internet to gain a vast amount of knowledge. Second, the models can use this knowledge to quickly align with human preferences and specific tasks.
The researchers identify three fundamental characteristics of foundation agents:
1. A unified representation of environment states, agent actions, and feedback signals.
2. A unified policy interface that can be applied to various tasks and domains, from robotics and gameplay to healthcare and beyond.
3. A decision-making process based on reasoning about world knowledge, the environment, and other agents.
“These characteristics constitute the uniqueness and challenges for foundation agents, empowering them with multi-modality perception, multi-task and cross-domain adaptation as well as few- or zero-shot generalization,” the researchers write.
The researchers propose a roadmap for developing foundation agents, which includes three key components.
First, large-scale interactive data must be collected from the internet and physical environments. In environments where real-world interactive data is scarce or risky to obtain, simulators and generative models such as Sora can be used.
Second, the foundation agents are pre-trained on the unlabeled data. This step enables the agent to learn decision-related knowledge representations that become useful when the model is customized for specific tasks. For example, the model can be fine-tuned on a small dataset where rewards or outcomes are available or can be customized through prompt engineering. The knowledge obtained during the pretraining phase enables the model to adapt to new tasks with much fewer examples during this customization phase.
“Self-supervised (unsupervised) pretraining for decision making allows foundation agents to learn without reward signals and encourages the agent to learn from suboptimal offline datasets,” the researchers write. “This is particularly applicable when large, unlabeled data can be easily collected from internet or real-world simulators.”
Third, foundation agents must be aligned with large language models to integrate world knowledge and human values.
Developing foundation agents presents several challenges compared to language and vision models. The information in the physical world is composed of low-level details instead of high-level abstractions. This makes it more difficult to create unified representations for the variables involved in the decision-making process.
There is also a large domain gap between different decision-making scenarios, which makes it difficult to develop a unified policy interface for foundation agents. For example, one solution can be to create a unified foundation model that takes into account all modalities, environments and possible actions. However, it can make the model increasingly complex and uninterpretable.
While language and vision models focus on understanding and generating content, foundation agents must be involved in the dynamic process of choosing optimal actions based on complex environmental information.
The authors suggest several directions of research that can help bridge the gap between current foundation models and foundation agents that can perform open-ended tasks and adapt to unpredictable environments and novel situations.
There have already been interesting advances in robotics, where the principles of control systems and foundation models are brought together to create systems that are more versatile and generalize well to situations and tasks that were not included in the training data. These models use the vast commonsense knowledge of LLMs and VLMs to reason about the world and choose the correct actions in previously unseen situations.
Another critical domain is self-driving cars, where researchers are exploring how large language models can be used to integrate commonsense knowledge and human cognitive abilities into autonomous driving systems. The researchers suggest other domains such as healthcare and science, where foundation agents can accomplish tasks alongside human experts.
“Foundation agents hold the potential to alter the landscape of agent learning for decision making, akin to the revolutionary impact of foundation models in language and vision,” the researchers write. “The enhanced perception, adaptation, and reasoning abilities of agents not only address limitations of conventional RL, but also hold the key to unleash the full potential of foundation agents in real-world decision making.”
Time’s almost up! There’s only one week left to request an invite to The AI Impact Tour on June 5th. Don’t miss out on this incredible opportunity to explore various methods for auditing AI models. Find out how you can attend here.
Foundation models have revolutionized the fields of computer vision and natural language processing. Now, a group of researchers believe the same principles can be applied to create foundation agents, AI systems that can perform open-ended decision-making tasks in the physical world.
In a new position paper, researchers at the University of Chinese Academy of Sciences describe foundation agents as “generally capable agents across physical and virtual worlds” that will be “the paradigm shift for decision making, akin to[large language models] LLMs as general-purpose language models to solve linguistic and knowledge-based tasks.”
Foundation agents will make it easier to create versatile AI systems for the real world and can have a great impact on fields that rely on brittle and task-specific AI systems.
The challenges of AI decision-making
Traditional approaches to AI decision-making have several shortcomings. Expert systems heavily rely on formalized human knowledge and manually crafted rules. Reinforcement learning systems (RL), which have become more popular in recent years, must be trained from scratch for every new task, which makes them sample-inefficient and limits their ability to generalize to new environments. Imitation learning (IL), where the AI learns decision-making from human demonstrations also requires extensive human efforts to craft training examples and action sequences.
June 5th: The AI Audit in NYC
Join us next week in NYC to engage with top executive leaders, delving into strategies for auditing AI models to ensure optimal performance and accuracy across your organization. Secure your attendance for this exclusive invite-only event.
In contrast, LLMs and vision language models (VLMs) can rapidly adapt to various tasks with minimal fine-tuning or prompting. The researchers believe that, with some adjustments, the same approach can be used to create foundation agents that can handle open-ended decision-making tasks in the physical and virtual worlds.
Some of the key characteristics of foundation models can help create foundation agents for the real world. First, LLMs can be pre-trained on large unlabeled datasets from the internet to gain a vast amount of knowledge. Second, the models can use this knowledge to quickly align with human preferences and specific tasks.
Characteristics of foundation agents
The researchers identify three fundamental characteristics of foundation agents:
1. A unified representation of environment states, agent actions, and feedback signals.
2. A unified policy interface that can be applied to various tasks and domains, from robotics and gameplay to healthcare and beyond.
3. A decision-making process based on reasoning about world knowledge, the environment, and other agents.
“These characteristics constitute the uniqueness and challenges for foundation agents, empowering them with multi-modality perception, multi-task and cross-domain adaptation as well as few- or zero-shot generalization,” the researchers write.
A roadmap for foundation agents

The researchers propose a roadmap for developing foundation agents, which includes three key components.
First, large-scale interactive data must be collected from the internet and physical environments. In environments where real-world interactive data is scarce or risky to obtain, simulators and generative models such as Sora can be used.
Second, the foundation agents are pre-trained on the unlabeled data. This step enables the agent to learn decision-related knowledge representations that become useful when the model is customized for specific tasks. For example, the model can be fine-tuned on a small dataset where rewards or outcomes are available or can be customized through prompt engineering. The knowledge obtained during the pretraining phase enables the model to adapt to new tasks with much fewer examples during this customization phase.
“Self-supervised (unsupervised) pretraining for decision making allows foundation agents to learn without reward signals and encourages the agent to learn from suboptimal offline datasets,” the researchers write. “This is particularly applicable when large, unlabeled data can be easily collected from internet or real-world simulators.”
Third, foundation agents must be aligned with large language models to integrate world knowledge and human values.
Challenges and opportunities for foundation agents
Developing foundation agents presents several challenges compared to language and vision models. The information in the physical world is composed of low-level details instead of high-level abstractions. This makes it more difficult to create unified representations for the variables involved in the decision-making process.
There is also a large domain gap between different decision-making scenarios, which makes it difficult to develop a unified policy interface for foundation agents. For example, one solution can be to create a unified foundation model that takes into account all modalities, environments and possible actions. However, it can make the model increasingly complex and uninterpretable.
While language and vision models focus on understanding and generating content, foundation agents must be involved in the dynamic process of choosing optimal actions based on complex environmental information.
The authors suggest several directions of research that can help bridge the gap between current foundation models and foundation agents that can perform open-ended tasks and adapt to unpredictable environments and novel situations.
There have already been interesting advances in robotics, where the principles of control systems and foundation models are brought together to create systems that are more versatile and generalize well to situations and tasks that were not included in the training data. These models use the vast commonsense knowledge of LLMs and VLMs to reason about the world and choose the correct actions in previously unseen situations.
Another critical domain is self-driving cars, where researchers are exploring how large language models can be used to integrate commonsense knowledge and human cognitive abilities into autonomous driving systems. The researchers suggest other domains such as healthcare and science, where foundation agents can accomplish tasks alongside human experts.
“Foundation agents hold the potential to alter the landscape of agent learning for decision making, akin to the revolutionary impact of foundation models in language and vision,” the researchers write. “The enhanced perception, adaptation, and reasoning abilities of agents not only address limitations of conventional RL, but also hold the key to unleash the full potential of foundation agents in real-world decision making.”
Author: Ben Dickson
Source: Venturebeat
Reviewed By: Editorial Team