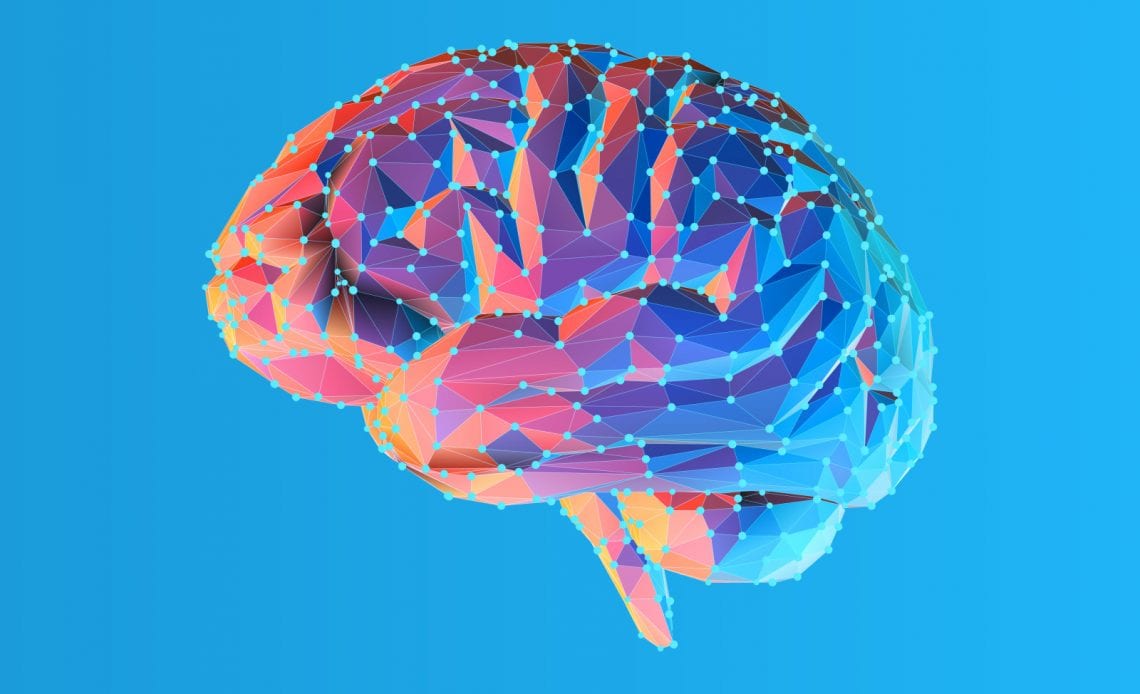
Welcome to Hitting the Books. With less than one in five Americans reading just for fun these days, we’ve done the hard work for you by scouring the internet for the most interesting, thought provoking books on science and technology we can find and delivering an easily digestible nugget of their stories.
Our modern world wouldn’t exist if not for machine learning. From telecommunications to transportation, medicine to aerospace, the accelerating advancement of artificial intelligence has proven a boon for humanity and the public good. However the same ability that allows them to learn from past experience can and likely will be leveraged for underhanded purposes like stifling commercial competition. In the excerpt below from Who’s Afraid of AI? author Thomas Ramge examines feedback loops’ impact on automation and how controlling the data generated by them could enable companies to unfairly influence the market.
For computer learning systems, the human platitude holds true: You never know until you try. As with people, however, it becomes true for computers only if the computer system recognizes whether its attempt succeeded or failed. Therefore, feedback data play a decisive (and often overlooked) role in learning computer systems. The more frequently and precisely a learning system receives feedback as to whether it has found the right telephone number, actually calculated the best route, or correctly diagnosed a skin condition from a photograph, the better and more quickly it learns.
Feedback is the technological core of all methods of controlling machines automatically. The American mathematician Norbert Wiener established the theoretical foundation for this — cybernetics — in the 1940s. Every technological system can be controlled and redirected according to its goals through feedback data. That sounds more complicated than it is.
Some of the first cybernetic systems were the US Army’s automatic rocket-defense systems used to defend British cities against German V-1 cruise missiles. Radar detected the German rockets, informed anti-aircraft cannons of the bomb’s position in a continuous feedback loop, and calculated its future flight path. The cannons aimed themselves according to the continuous feedback signals and then fired at (hopefully) just the right moment. At the end of the war, the British and Americans were shooting about 70 percent of the “vengeance weapons” out of the sky.
Thankfully, feedback loops have led to more than military innovations. Without them, the Apollo missions would never have landed on the Moon, no jetliner would fly across the oceans safely, no injection pump could provide gasoline to pistons with perfect timing, and no elevator door would reopen when a human leg is caught in it. But in no other field are feedback loops as valuable as they are in artificial intelligence. They are its most important raw material.
Feedback data are at work when we begin to type a term into Google and Google immediately suggests what it presumes we are looking for. In fact, Google’s suggestion might be an even better search term, because many other Google users have already given the system feedback that the term is frequently searched for when they clicked on a Google suggestion as they typed in the same or a similar search term. Then, when we accept a suggestion, we create additional feedback data. If we instead type out a different term, we do the same thing, as well. Amazon optimizes its recommendation algorithms using feedback data, and Facebook does the same for the constellation of posts that a user sees in his or her timeline. These data help PayPal predict with ever-improving accuracy whether a payment might be fraudulent; and as you can imagine, feedback about fraudulent charges tends to be quite vehement.
data has a similar effect in the age of artificial intelligence that economies of scale had for mass production during industrialization and network effects have had for the digital economy of the last twenty-five years. Economies of scale reduced the cost per item for physical products ranging from Ford’s Model T, to Sony’s tube televisions, to Huawei’s smartphones, to a degree that Frederick Winslow Taylor, the inventor of scientific management, could hardly have imagined. The network effect—extensively investigated by the Stanford economists Carl Shapiro and Hal Varian—led to monopoly positions for digital platforms such as Amazon, eBay, and Alibaba, Facebook and WeChat, and Uber and DiDi. The network effect means that with each new participant, the platform becomes more attractive to everyone who uses it. The more people who use WhatsApp, the more users install the app, because it’s more possible to contact friends or acquaintances through the app or to participate in group chats. The more smartphones that run the Android operating system, the more attractive it is for developers to develop apps for Android, again raising the attractiveness of the operating system.
The feedback effect in artificial intelligence, on the other hand, leads to systems becoming more intelligent as more people provide the machine with feedback data. Feedback data are at the center of intelligent technology’s learning processes. Over the next several years, digital feedback will lead to commercially viable autonomous driving systems, language translation programs, and image recognition. And feedback data will cause lawmakers considerable headaches, because without new measures to guard against monopolies, the accumulation of feedback data over the long term will lead almost inexorably to data monopolies. The most popular products and services will quickly improve because the most feedback data will be fed into them. Machine learning will to some degree be built into these products, which means that innovative newcomers will have a chance against the top dogs of the AI-driven economy only in exceptional cases. Self-improving technology shuts out competition. Human beings will have to find a legal answer to this technological problem.
Excerpted from Who’s Afraid of AI?: Fear and Promise in the Age of Thinking Machines © Thomas Ramge, 2018. Translation © Thomas Ramge, 2019. Reprinted by permission of the publisher, The Experiment. Available wherever books are sold. theexperimentpublishing.com
Author: Andrew Tarantola
Source: Engadget
Tags: AI, Amazon, column, data, Facebook, Google, hitting the books, hittingthebooks, monopoly, PayPal, robots, thomasramge, tomorrow, who’safraidofai