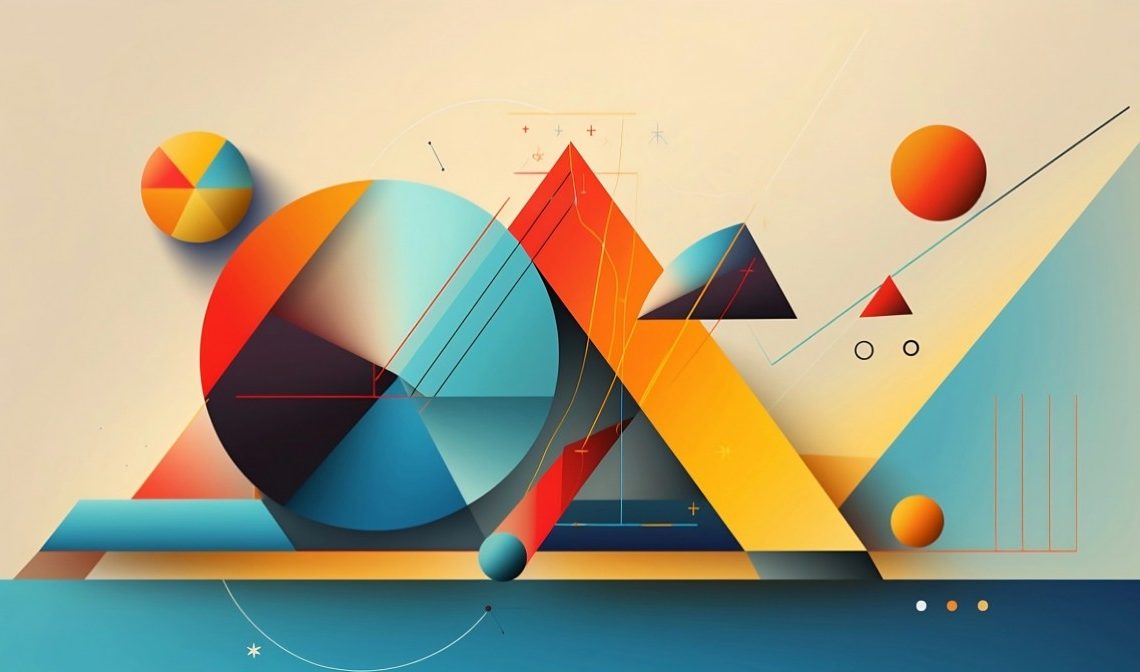
A new artificial intelligence system developed by Google DeepMind, one of the world’s leading AI labs, can solve complex geometry problems at a level comparable to a human gold medalist in the International Mathematical Olympiad (IMO), a prestigious competition for high-school students.
The system, called AlphaGeometry, combines two different approaches: a neural language model that generates intuitive ideas, and a symbolic deduction engine that verifies them using formal logic and rules. The language model is based on the same technology that powers Google’s search engine and natural language understanding systems. The deduction engine is inspired by a method devised by the Chinese mathematician Wen-Tsün Wu in 1978.
The researchers tested AlphaGeometry on 30 geometry problems from the IMO, which are considered challenging even for expert mathematicians. The system solved 25 problems within the standard time limit of 4.5 hours, matching the average score of human gold medalists on the same problems. The previous best system, based on Wu’s method, solved only 10 problems.
The results, published today in Nature, show that AI can reason logically and discover new mathematical knowledge.
Mathematics, and geometry in particular, have been a longstanding challenge for AI researchers, because they require both creativity and rigidity. Unlike text-based AI models, which can be trained on massive amounts of data from the web, there is relatively little data available for mathematics, which is more symbolic and domain-specific. Moreover, solving mathematics problems requires logical reasoning, something that most current AI models are not very good at.
To overcome these challenges, the researchers developed a novel neuro-symbolic approach that leverages the strengths of both neural networks and symbolic systems. Neural networks are good at recognizing patterns and predicting next steps, but they often make mistakes or lack explanations. Symbolic systems, on the other hand, are based on formal logic and strict rules, which allow them to correct and justify the neural network’s decisions.
The researchers compared their approach to the idea of “thinking, fast and slow”, popularized by the Nobel laureate Daniel Kahneman. One system provides fast, “intuitive” ideas, and the other, more deliberate, rational decision-making. These two systems, responsible for creative thinking and logical reasoning respectively, work together to solve difficult mathematical problems.
The researchers also showed that AlphaGeometry can generalize to unseen problems and discover new theorems that are not explicitly stated in the problem statement. For example, the system was able to prove a theorem about the angle bisector of a triangle, which was not given as a premise or a goal in the problem.
The researchers hope that their system, which they have open-sourced, will inspire further research and applications in mathematics, science, and AI. They also acknowledge the limitations and challenges of their work, such as the need for more human-readable proofs, the scalability to more complex problems, and the ethical implications of AI systems in mathematics.
While AlphaGeometry is currently limited to geometry proofs, the researchers believe their synthetic data methodology could allow AI reasoning to flourish in areas of math and science where human-generated training data is scarce. By automating the discovery and verification of new knowledge, machine learning may soon accelerate human understanding across many disciplines.
A new artificial intelligence system developed by Google DeepMind, one of the world’s leading AI labs, can solve complex geometry problems at a level comparable to a human gold medalist in the International Mathematical Olympiad (IMO), a prestigious competition for high-school students.
The system, called AlphaGeometry, combines two different approaches: a neural language model that generates intuitive ideas, and a symbolic deduction engine that verifies them using formal logic and rules. The language model is based on the same technology that powers Google’s search engine and natural language understanding systems. The deduction engine is inspired by a method devised by the Chinese mathematician Wen-Tsün Wu in 1978.
The researchers tested AlphaGeometry on 30 geometry problems from the IMO, which are considered challenging even for expert mathematicians. The system solved 25 problems within the standard time limit of 4.5 hours, matching the average score of human gold medalists on the same problems. The previous best system, based on Wu’s method, solved only 10 problems.

The results, published today in Nature, show that AI can reason logically and discover new mathematical knowledge.
Mathematics, and geometry in particular, have been a longstanding challenge for AI researchers, because they require both creativity and rigidity. Unlike text-based AI models, which can be trained on massive amounts of data from the web, there is relatively little data available for mathematics, which is more symbolic and domain-specific. Moreover, solving mathematics problems requires logical reasoning, something that most current AI models are not very good at.
To overcome these challenges, the researchers developed a novel neuro-symbolic approach that leverages the strengths of both neural networks and symbolic systems. Neural networks are good at recognizing patterns and predicting next steps, but they often make mistakes or lack explanations. Symbolic systems, on the other hand, are based on formal logic and strict rules, which allow them to correct and justify the neural network’s decisions.
The researchers compared their approach to the idea of “thinking, fast and slow”, popularized by the Nobel laureate Daniel Kahneman. One system provides fast, “intuitive” ideas, and the other, more deliberate, rational decision-making. These two systems, responsible for creative thinking and logical reasoning respectively, work together to solve difficult mathematical problems.
The researchers also showed that AlphaGeometry can generalize to unseen problems and discover new theorems that are not explicitly stated in the problem statement. For example, the system was able to prove a theorem about the angle bisector of a triangle, which was not given as a premise or a goal in the problem.
The researchers hope that their system, which they have open-sourced, will inspire further research and applications in mathematics, science, and AI. They also acknowledge the limitations and challenges of their work, such as the need for more human-readable proofs, the scalability to more complex problems, and the ethical implications of AI systems in mathematics.
While AlphaGeometry is currently limited to geometry proofs, the researchers believe their synthetic data methodology could allow AI reasoning to flourish in areas of math and science where human-generated training data is scarce. By automating the discovery and verification of new knowledge, machine learning may soon accelerate human understanding across many disciplines.
VentureBeat’s mission is to be a digital town square for technical decision-makers to gain knowledge about transformative enterprise technology and transact. Discover our Briefings.
Author: Michael Nuñez
Source: Venturebeat
Reviewed By: Editorial Team