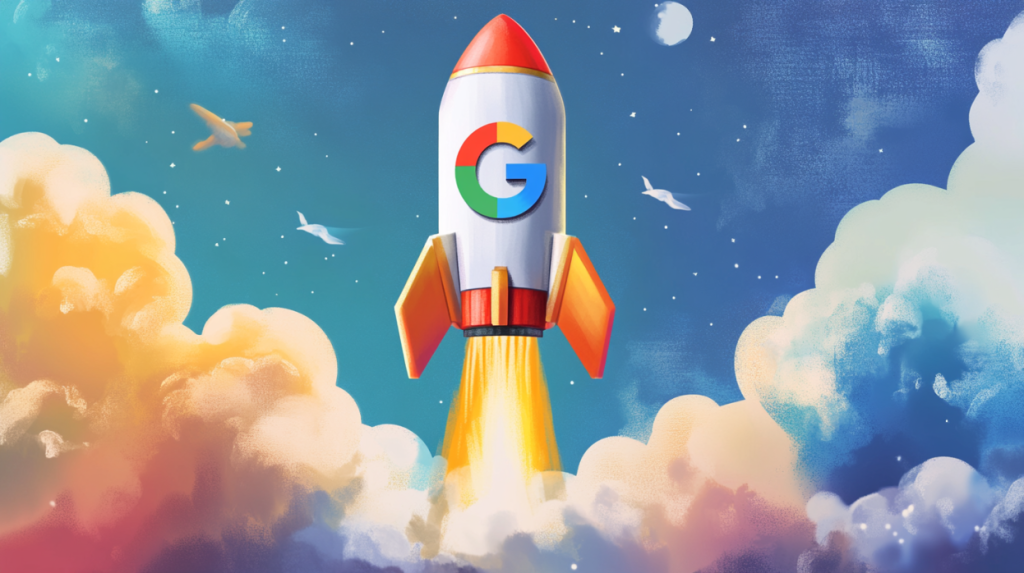
Just a year ago, the narrative around Google and enterprise AI felt stuck. Despite inventing core technologies like the Transformer, the tech giant seemed perpetually on the back foot, overshadowed by OpenAI‘s viral success, Anthropic‘s coding prowess and Microsoft‘s aggressive enterprise push.
But witness the scene at Google Cloud Next 2025 in Las Vegas last week: A confident Google, armed with benchmark-topping models, formidable infrastructure and a cohesive enterprise strategy, declaring a stunning turnaround. In a closed-door analyst meeting with senior Google executives, one analyst summed it up. This feels like the moment, he said, when Google went from “catch up, to catch us.”
This sentiment that Google has not only caught up with but even surged ahead of OpenAI and Microsoft in the enterprise AI race prevailed throughout the event. And it’s more than just Google’s marketing spin. Evidence suggests Google has leveraged the past year for intense, focused execution, translating its technological assets into a performant, integrated platform that’s rapidly winning over enterprise decision-makers. From boasting the world’s most powerful AI models running on hyper-efficient custom silicon, to a burgeoning ecosystem of AI agents designed for real-world business problems, Google is making a compelling case that it was never actually lost – but that its stumbles masked a period of deep, foundational development.
Now, with its integrated stack firing on all cylinders, Google appears positioned to lead the next phase of the enterprise AI revolution. And in my interviews with several Google executives at Next, they said Google wields advantages in infrastructure and model integration that competitors like OpenAI, Microsoft or AWS will struggle to replicate.
The shadow of doubt: acknowledging the recent past
It’s impossible to appreciate the current momentum without acknowledging the recent past. Google was the birthplace of the Transformer architecture, which sparked the modern revolution in large language models (LLMs). Google also started investing in specialized AI hardware (TPUs), which are now driving industry-leading efficiency, a decade ago. And yet, two and a half years ago, it inexplicably found itself playing defense.
OpenAI’s ChatGPT captured the public imagination and enterprise interest at breathtaking speed and became the fastest-growing app in history. Competitors like Anthropic carved out niches in areas like coding.
Google’s own public steps sometimes seemed tentative or flawed. The infamous Bard demo fumbles in 2023 and the later controversy over its image generator producing historically inaccurate depictions fed a narrative of a company potentially hampered by internal bureaucracy or overcorrection on alignment. It felt like Google was lost: The AI stumbles seemed to fit a pattern, first shown by Google’s initial slowness in the cloud competition, where it remained a distant third in market share behind Amazon and Microsoft. Google Cloud CTO Will Grannis acknowledged the early questions about whether Google Cloud would stand behind in the long run. “Is it even a real thing?,” he recalled people asking him. The question lingered: Could Google translate its undeniable research brilliance and infrastructure scale into enterprise AI dominance?
The pivot: a conscious decision to lead
Behind the scenes, however, a shift was underway, catalyzed by a conscious decision at the highest levels to reclaim leadership. Mat Velloso, VP of product for Google DeepMind’s AI Developer Platform, described sensing a pivotal moment upon joining Google in Feb. 2024, after leaving Microsoft. “When I came to Google, I spoke with Sundar [Pichai], I spoke with several leaders here, and I felt like that was the moment where they were deciding, okay, this [generative AI] is a thing the industry clearly cares about. Let’s make it happen,” Velloso shared in an interview with VentureBeat during Next last week.
This renewed push wasn’t hampered by a feared “brain drain” that some outsiders felt was depleting Google. In fact, the company quietly doubled down on execution in early 2024 – a year marked by aggressive hiring, internal unification and customer traction. While competitors made splashy hires, Google retained its core AI leadership, including DeepMind CEO Demis Hassabis and Google Cloud CEO Thomas Kurian, providing stability and deep expertise.
Moreover, talent began flowing towards Google’s focused mission. Logan Kilpatrick, for instance, returned to Google from OpenAI, drawn by the opportunity to build foundational AI within the company, creating it. He joined Velloso in what he described as a “zero to one experience,” tasked with building developer traction for Gemini from the ground up. “It was like the team was me on day one… we actually have no users on this platform, we have no revenue. No one is interested in Gemini at this moment,” Kilpatrick recalled of the starting point. People familiar with the internal dynamics also credit leaders like Josh Woodward, who helped start AI Studio and now leads the Gemini App and Labs. More recently, Noam Shazeer, a key co-author of the original “Attention Is All You Need” Transformer paper during his first tenure at Google, returned to the company in late 2024 as a technical co-lead for the crucial Gemini project
This concerted effort, combining these hires, research breakthroughs, refinements to its database technology and a sharpened enterprise focus overall, began yielding results. These cumulative advances, combined with what CTO Will Grannis termed “hundreds of fine-grain” platform elements, set the stage for the announcements at Next ’25, and cemented Google’s comeback narrative.
Pillar 1: Gemini 2.5 and the era of thinking models
It’s true that a leading enterprise mantra has become “it’s not just about the model.” After all, the performance gap between leading models has narrowed dramatically, and tech insiders acknowledge that true intelligence is coming from technology packaged around the model, not just the model itself – for example, agentic technologies that allow a model to use tools and explore the web around it.
Despite this, to possess the demonstrably best-performing LLM is an important feat – and a powerful validator, a sign that the model-owning company has things like superior research and the most efficient underlying technology architecture. With the release of Gemini 2.5 Pro just weeks before Next ’25, Google definitively seized that mantle. It quickly topped the independent Chatbot Arena leaderboard, significantly outperforming even OpenAI’s latest GPT-4o variant, and aced notoriously difficult reasoning benchmarks like Humanity’s Last Exam. As Pichai stated in the keynote, “It’s our most intelligent AI model ever. And it is the best model in the world.” The model had driven an 80 percent increase in Gemini usage within a month, he Tweeted separately.
For the first time, Google’s Gemini demand was on fire. As I detailed previously, aside from Gemini 2.5 Pro’s raw intelligence, what impressed me is its demonstrable reasoning. Google has engineered a “thinking” capability, allowing the model to perform multi-step reasoning, planning, and even self-reflection before finalizing a response. The structured, coherent chain-of-thought (CoT) – using numbered steps and sub-bullets – avoids the rambling or opaque nature of outputs from other models from DeepSeek or OpenAI. For technical teams evaluating outputs for critical tasks, this transparency allows validation, correction, and redirection with unprecedented confidence.
But more importantly for enterprise users, Gemini 2.5 Pro also dramatically closed the gap in coding, which is one of the biggest application areas for generative AI. In an interview with VentureBeat, CTO Fiona Tan, the CTO of leading retailer Wayfair, said that after initial tests, the company found it “stepped up quite a bit” and was now “pretty comparable” to Anthropic’s Claude 3.7 Sonnet, previously the preferred choice for many developers.
Google also added a massive 1 million token context window to the model, enabling reasoning across entire codebases or lengthy documentation, far exceeding the capabilities of the models of OpenAI or Anthropic. (OpenAI responded this week with models featuring similarly large context windows, though benchmarks suggest Gemini 2.5 Pro retains an edge in overall reasoning). This advantage allows for complex, multi-file software engineering tasks.
Complementing Pro is Gemini 2.5 Flash, announced at Next ’25 and released just yesterday. Also, a “thinking” model, Flash is optimized for low latency and cost-efficiency. You can control how much the model reasons and balance performance with your budget. This tiered approach further reflects the “intelligence per dollar” strategy championed by Google executives.
Velloso showed a chart revealing that across the intelligence spectrum, Google models offer the best value. “If we had this conversation one year ago… I would have nothing to show,” Velloso admitted, highlighting the rapid turnaround. “And now, like, across the board, we are, if you’re looking for whatever model, whatever size, like, if you’re not Google, you’re losing money.” Similar charts have been updated to account for OpenAI’s latest model releases this week, all showing the same thing: Google’s models offer the best intelligence per dollar. See below:

For any given price, Google’s models offer more intelligence than other models, about 90 percent of the time. Source: Pierre Bongrand.
Wayfair’s Tan said she also observed promising latency improvements with 2.5 Pro: “Gemini 2.5 came back faster,” making it viable for “more customer-facing sort of capabilities,” she said, something she said hasn’t been the case before with other models. Gemini could become the first model Wayfair uses for these customer interactions, she said.
The Gemini family’s capabilities extend to multimodality, integrating seamlessly with Google’s other leading models like Imagen 3 (image generation), Veo 2 (video generation), Chirp 3 (audio), and the newly announced Lyria (text-to-music), all accessible via Google’s platform for Enterprise users, Vertex. Google is the only company that offers its own generative media models across all modalities on its platform. Microsoft, AWS and OpenAI have to partner with other companies to do this.
Pillar 2: Infrastructure prowess – the engine under the hood
The ability to rapidly iterate and efficiently serve these powerful models stems from Google’s arguably unparalleled infrastructure, honed over decades of running planet-scale services. Central to this is the Tensor Processing Unit (TPU).
At Next ’25, Google unveiled Ironwood, its seventh-generation TPU, explicitly designed for the demands of inference and “thinking models.” The scale is immense, tailored for demanding AI workloads: Ironwood pods pack over 9,000 liquid-cooled chips, delivering a claimed 42.5 exaflops of compute power. Google’s VP of ML Systems Amin Vahdat said on stage at Next that this is “more than 24 times” the compute power of the world’s current #1 supercomputer.
Google stated that Ironwood offers 2x perf/watt relative to Trillium, the previous generation of TPU. This is significant since enterprise customers increasingly say energy costs and availability constrain large-scale AI deployments.
Google Cloud CTO Will Grannis emphasized the consistency of this progress. Year over year, Google is making 10x, 8x, 9x, 10x improvements in its processors, he told VentureBeat in an interview, creating what he called a “hyper Moore’s law” for AI accelerators. He said customers are buying Google’s roadmap, not just its technology.
Google’s position fueled this sustained TPU investment. It needs to efficiently power massive services like Search, YouTube, and Gmail for more than 2 billion users. This necessitated developing custom, optimized hardware long before the current generative AI boom. While Meta operates at a similar consumer scale, other competitors lacked this specific internal driver for decade-long, vertically integrated AI hardware development.
Now these TPU investments are paying off because they are driving the efficiency not only for its own apps, but they also allow Google to offer Gemini to other users at a better intelligence per dollar, everything equal.
Why can’t Google’s competitors buy efficient processors from Nvidia, you ask? It’s true that Nvidia’s GPU processors dominate the process pre-training of LLMs. But market demand has pushed up the price of these GPUs, and Nvidia takes a healthy cut for itself as profit. This passes significant costs along to users of its chips. And also, while pre-training has dominated the usage of AI chips so far, this is changing now that enterprises are actually deploying these applications. This is where ” inference” comes in, and here TPUs are considered more efficient than GPUs for workloads at scale.
When you ask Google executives where their main technology advantage in AI comes from, they usually fall back to the TPU as the most important. Mark Lohmeyer, the VP who runs Google’s computing infrastructure, was unequivocal: TPUs are “certainly a highly differentiated part of what we do… OpenAI, they don’t have those capabilities.”
Significantly, Google presents TPUs not in isolation, but as part of the wider, more complex enterprise AI architecture. For technical insiders, it’s understood that top-tier performance hinges on integrating increasingly specialized technology breakthroughs. Many updates were detailed at Next. Vahdat described this as a “supercomputing system,” integrating hardware (TPUs, the latest Nvidia GPUs like Blackwell and upcoming Vera Rubin, advanced storage like Hyperdisk Exapools, Anywhere Cache, and Rapid Storage) with a unified software stack. This software includes Cluster Director for managing accelerators, Pathways (Gemini’s distributed runtime, now available to customers), and bringing optimizations like vLLM to TPUs, allowing easier workload migration for those previously on Nvidia/PyTorch stacks. This integrated system, Vahdat argued, is why Gemini 2.0 Flash achieves 24 times higher intelligence per dollar, compared to GPT-4o.
Google is also extending its physical infrastructure reach. Cloud WAN makes Google’s low-latency 2-million-mile private fiber network available to enterprises, promising up to 40% faster performance and 40% lower total cost of ownership (TCO) compared to customer-managed networks.
Furthermore, Google Distributed Cloud (GDC) allows Gemini and Nvidia hardware (via a Dell partnership) to run in sovereign, on-premises, or even air-gapped environments – a capability Nvidia CEO Jensen Huang lauded as “utterly gigantic” for bringing state-of-the-art AI to regulated industries and nations. At Next, Huang called Google’s infrastructure the best in the world: “No company is better at every single layer of computing than Google and Google Cloud,” he said.
Pillar 3: The integrated full stack – connecting the dots
Google’s strategic advantage grows when considering how these models and infrastructure components are woven into a cohesive platform. Unlike competitors, which often rely on partnerships to bridge gaps, Google controls nearly every layer, enabling tighter integration and faster innovation cycles.
So why does this integration matter, if a competitor like Microsoft can simply partner with OpenAI to match infrastructure breadth with LLM model prowess? The Googlers I talked with said it makes a huge difference, and they came up with anecdotes to back it up.
Take the significant improvement of Google’s enterprise database BigQuery. The database now offers a knowledge graph that allows LLMs to search over data much more efficiently, and it now boasts more than five times the customers of competitors like Snowflake and Databricks, VentureBeat reported yesterday. Yasmeen Ahmad, Head of Product for Data Analytics at Google Cloud, said the vast improvements were only possible because Google’s data teams were working closely with the DeepMind team. They worked through use cases that were hard to solve, and this led to the database providing 50 percent more accuracy based on common queries, at least according to Google’s internal testing, in getting to the right data than the closest competitors, Ahmad told VentureBeat in an interview. Ahmad said this sort of deep integration across the stack is how Google has “leapfrogged” the industry.
This internal cohesion contrasts sharply with the “frenemies” dynamic at Microsoft. While Microsoft partners with OpenAI to distribute its models on the Azure cloud, Microsoft is also building its own models. Mat Velloso, the Google executive who now leads the AI developer program, left Microsoft after getting frustrated trying to align Windows Copilot plans with OpenAI’s model offerings. “How do you share your product plans with another company that’s actually competing with you… The whole thing is a contradiction,” he recalled. “Here I sit side by side with the people who are building the models.”
This integration speaks to what Google leaders see as their core advantage: its unique ability to connect deep expertise across the full spectrum, from foundational research and model building to “planet-scale” application deployment and infrastructure design.
Vertex AI serves as the central nervous system for Google’s enterprise AI efforts. And the integration goes beyond just Google’s own offerings. Vertex’s Model Garden offers over 200 curated models, including Google’s, Meta’s Llama 4, and numerous open-source options. Vertex provides tools for tuning, evaluation (including AI-powered Evals, which Grannis highlighted as a key accelerator), deployment, and monitoring. Its grounding capabilities leverage internal AI-ready databases alongside compatibility with external vector databases. Add to that Google’s new offerings to ground models with Google Search, the world’s best search engine.
Integration extends to Google Workspace. New features announced at Next ’25, like “Help Me Analyze” in Sheets (yes, Sheets now has an “=AI” formula), Audio Overviews in Docs and Workspace Flows, further embed Gemini’s capabilities into daily workflows, creating a powerful feedback loop for Google to use to improve the experience.
While driving its integrated stack, Google also champions openness where it serves the ecosystem. Having driven Kubernetes adoption, it’s now promoting JAX for AI frameworks and now open protocols for agent communication (A2A) alongside support for existing standards (MCP). Google is also offering hundreds of connectors to external platforms from within Agentspace, which is Google’s new unified interface for employees to find and use agents. This hub concept is compelling. The keynote demonstration of Agentspace (starting at 51:40) illustrates this. Google offers users pre-built agents, or employees or developers can build their own using no-code AI capabilities. Or they can pull in agents from the outside via A2A connectors. It integrates into the Chrome browser for seamless access.
Pillar 4: Focus on enterprise value and the agent ecosystem
Perhaps the most significant shift is Google’s sharpened focus on solving concrete enterprise problems, particularly through the lens of AI agents. Thomas Kurian, Google Cloud CEO, outlined three reasons customers choose Google: the AI-optimized platform, the open multi-cloud approach allowing connection to existing IT, and the enterprise-ready focus on security, sovereignty, and compliance.
Agents are key to this strategy. Aside from AgentSpace, this also includes:
Building Blocks: The open-source Agent Development Kit (ADK), announced at Next, has already seen significant interest from developers. The ADK simplifies creating multi-agent systems, while the proposed Agent2Agent (A2A) protocol aims to ensure interoperability, allowing agents built with different tools (Gemini ADK, LangGraph, CrewAI, etc.) to collaborate. Google’s Grannis said that A2A anticipates the scale and security challenges of a future with potentially hundreds of thousands of interacting agents.
This A2A protocol is really important. In a background interview with VentureBeat this week, the CISO of a major US retailer, who requested anonymity because of the sensitivity around security issues. But they said the A2A protocol was helpful because the retailer is looking for a solution to distinguish between real people and bots who are using agents to buy products. This retailer wants to avoid selling to scalper bots, and with A2A, it’s easier to negotiate with agents to verify their owner identities.
Purpose-built Agents: Google showcased expert agents integrated into Agentspace (like NotebookLM, Idea Generation, Deep Research) and highlighted five key categories gaining traction: Customer Agents (powering tools like Reddit Answers, Verizon’s support assistant, Wendy’s drive-thru), Creative Agents (used by WPP, Brandtech, Sphere), Data Agents (driving insights at Mattel, Spotify, Bayer), Coding Agents (Gemini Code Assist), and Security Agents (integrated into the new Google Unified Security platform).
This comprehensive agent strategy appears to be resonating. Conversations with executives at three other large enterprises this past week, also speaking anonymously due to competitive sensitivities, echoed this enthusiasm for Google’s agent strategy. Google Cloud COO Francis DeSouza confirmed in an interview: “Every conversation includes AI. Specifically, every conversation includes agents.”
Kevin Laughridge, an executive at Deloitte, a big user of Google’s AI products, and a distributor of them to other companies, described the agent market as a “land grab” where Google’s early moves with protocols and its integrated platform offer significant advantages. “Whoever is getting out first and getting the most agents that actually deliver value – is who is going to win in this race,” Laughridge said in an interview. He said Google’s progress was “astonishing,” noting that custom agents Deloitte built just a year ago could now be replicated “out of the box” using Agentspace. Deloitte itself is building 100 agents on the platform, targeting mid-office functions like finance, risk and engineering, he said.
The customer proof points are mounting. At Next, Google cited “500 plus customers in production” with generative AI, up from just “dozens of prototypes” a year ago. If Microsoft was perceived as way ahead a year ago, that doesn’t seem so obviously the case anymore. Given the PR war from all sides, it’s difficult to say who is really winning right now definitively. Metrics vary. Google’s 500 number isn’t directly comparable to the 400 case studies Microsoft promotes (and Microsoft, in response, told VentureBeat at press time that it plans to update this public count to 600 shortly, underscoring the intense marketing). And if Google’s distribution of AI through its apps is significant, Microsoft’s Copilot distribution through its 365 offering is equally impressive. Both are now hitting millions of developers through APIs.
[Editor’s note: Understanding how enterprises are navigating this ‘agent land grab,’ and successfully deploying these complex AI solutions, will be central to the discussions at VentureBeat’s Transform event this June 24-25 in San Francisco.]
But examples abound of Google’s traction:
- Wendy’s: Deployed an AI drive-thru system to thousands of locations in just one year, improving employee experience and order accuracy. Google Cloud CTO Will Grannis noted that the AI system is capable of understanding slang and filtering out background noise, significantly reducing the stress of live customer interactions. That frees up staff to focus on food prep and quality — a shift Grannis called “a great example of AI streamlining real-world operations.”
- Salesforce: Announced a major expansion, enabling its platform to run on Google Cloud for the first time (beyond AWS), citing Google’s ability to help them “innovate and optimize.”
- Honeywell & Intuit: Companies previously strongly associated with Microsoft and AWS, respectively, now partnering with Google Cloud on AI initiatives.
- Major Banks (Deutsche Bank, Wells Fargo): Leveraging agents and Gemini for research, analysis, and modernizing customer service.
- Retailers (Walmart, Mercado Libre, Lowe’s): Using search, agents, and data platforms.
This enterprise traction fuels Google Cloud’s overall growth, which has outpaced AWS and Azure for the last three quarters. Google Cloud reached a $44 billion annualized run rate in 2024, up from just $5 billion in 2018.
Navigating the competitive waters
Google’s ascent doesn’t mean competitors are standing still. OpenAI’s rapid releases this week of GPT-4.1 (focused on coding and long context) and the o-series (multimodal reasoning, tool use) demonstrate OpenAI’s continued innovation. Moreover, OpenAI’s new image generation feature update in GPT-4o fueled massive growth over just the last month, helping ChatGPT reach 800 million users. Microsoft continues to leverage its vast enterprise footprint and OpenAI partnership, while Anthropic remains a strong contender, particularly in coding and safety-conscious applications.
However, it’s indisputable that Google’s narrative has improved remarkably. Just a year ago, Google was viewed as a stodgy, halting, blundering competitor that perhaps was about to blow its chance at leading AI at all. Instead, its unique, integrated stack and corporate steadfastness has revealed something else: Google possesses world-class capabilities across the entire spectrum – from chip design (TPUs) and global infrastructure to foundational model research (DeepMind), application development (Workspace, Search, YouTube), and enterprise cloud services (Vertex AI, BigQuery, Agentspace). “We’re the only hyperscaler that’s in the foundational model conversation,” deSouza stated flatly. This end-to-end ownership allows for optimizations (like “intelligence per dollar”) and integration depth that partnership-reliant models struggle to match. Competitors often need to stitch together disparate pieces, potentially creating friction or limiting innovation speed.
Google’s moment is now
While the AI race remains dynamic, Google has assembled all these pieces at the precise moment the market demands them. As Deloitte’s Laughridge put it, Google hit a point where its capabilities aligned perfectly “where the market demanded it.” If you were waiting for Google to prove itself in enterprise AI, you may have missed the moment — it already has. The company that invented many of the core technologies powering this revolution appears to have finally caught up – and more than that, it is now setting the pace that competitors need to match.
In the video below, recorded right after Next, AI expert Sam Witteveen and I break down the current landscape and emerging trends, and why Google’s AI ecosystem feels so strong:
Author: Matt Marshall
Source: Venturebeat
Reviewed By: Editorial Team