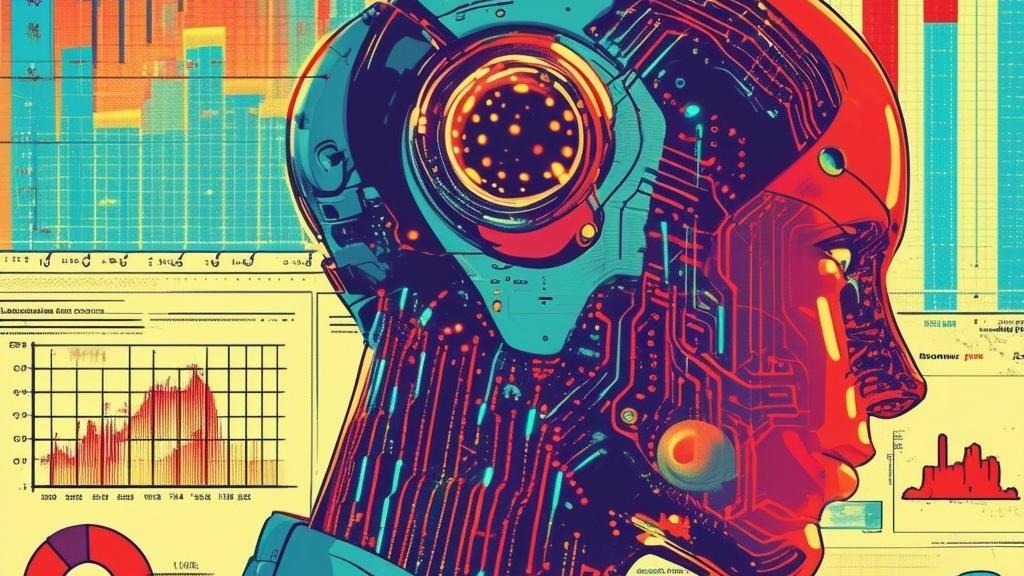
As I’ve been organizing VB Transform, our event next week focused on enterprise generative AI, I’ve noticed a stark shift in the scores of conversations I’m having with tech leaders. A year ago, it was all about how to embrace the magic of OpenAI’s GPT-4 throughout the company. Now their focus is on practical implementation and ROI. It’s as if the entire industry has hit reality mode.
As we enter the second half of 2024, the artificial intelligence landscape is undergoing a seismic shift. The initial excitement following OpenAI’s release of ChatGPT — which became the fastest product in history to attract 100 million users — has begun to wane. We’re moving from an era of near unbridled hype to one of reality, where enterprises are grappling with how to implement AI technologies in real products.
OpenAI CEO Sam Altman’s pronouncements of “magic intelligence in the sky” sparked a frenzy among Silicon Valley developers, many of whom came to believe we were on the cusp of achieving human-level machine intelligence across all domains, known as artificial general intelligence (AGI).
However, as 2024 progresses, a more nuanced narrative is emerging. Enterprises grounded in the practicalities of implementing AI in real-world applications are taking a more measured approach. The realization is setting in that while large language models (LLMs) like GPT-4o are incredibly powerful, generative AI overall has not lived up to Silicon Valley’s lofty expectations. LLM performance has plateaued, facing persistent challenges with factual accuracy. Legal and ethical concerns abound, and infrastructure and business use cases have proved more challenging than anticipated. We’re clearly not on a direct path to AGI as some had hoped. Even more modest promises, like autonomous AI agents, face plenty of limitations. And conservative technologies meant to “ground” AI with real data and accuracy, like RAG (retrieval-augmented generation), still have massive hurdles. Basically, LLMs still hallucinate like crazy.
Instead, companies are focusing on how to leverage the impressive basic capabilities of LLMs already available. This shift from hype to reality is underscored by six critical debates that are shaping the AI landscape. These debates represent fault lines between the zealous believers in imminent superintelligence and those advocating for a more pragmatic approach to AI adoption. For enterprise leaders, understanding these debates is crucial. There are significant stakes for companies looking to exploit this powerful technology, even if it’s not the godlike force its most ardent proponents claim.
Don’t read this wrong. Most enterprise leaders still believe that the technology has already produced profound benefits. During our recent AI Impact Tour, where meetings and events were held with Fortune 500 companies across the country, leaders openly discussed their efforts to embrace AI’s promise.
But these six debates will be central to discussions at our upcoming VB Transform event, scheduled for July 9-11 in the heart of San Francisco’s SOMA district. We’ve curated the event based on extensive conversations with executives from the largest players in AI.
The speaker lineup includes representatives from industry giants like OpenAI, Anthropic, Nvidia, Microsoft, Google, and Amazon, as well as AI leaders from Fortune 500 companies such as Kaiser Permanente, Walmart, and Bank of America.
The live debates and discussions at Transform promise to shed light on these critical issues, offering in-person attendees a unique opportunity to engage with leaders at the forefront of enterprise AI implementation.
Now, let’s dive into the six debates:
The race to develop the most advanced LLM has been a defining feature of the AI landscape since OpenAI’s GPT-3 burst onto the scene. But as we enter the second half of 2024, a question looms large: Is the LLM race over?
The answer appears to be yes, at least for now.
This matters because the differences between leading LLMs have become increasingly imperceptible, meaning enterprise companies can now select based on price, efficiency, and specific use-case fit rather than chasing the “best” model.
In 2023, we witnessed a dramatic race unfold. OpenAI surged ahead with the release of GPT-4 in March, showcasing significant improvements in reasoning, multi-modal capabilities, and multilingual proficiency. Pundits assumed that performance would continue to scale as more data was fed into these models. For a while, it looked like they were right.
But in 2024, the pace has slowed considerably. Despite vague promises from Altman suggesting more delights were coming, the company’s COO Mira Murati admitted in mid-June that OpenAI doesn’t have anything more in its labs than what is already public.
Now, we’re seeing clear signs of plateauing. OpenAI appears to have hit a wall, and its rival Anthropic has caught up, launching Claude 3.5 Sonnet, which outperforms GPT-4 on many measures. What’s notable is that Claude wasn’t able to leap far ahead; it’s only incrementally better. More tellingly, Sonnet is based on one of Anthropic’s smaller models, not its larger Opus model – suggesting that massive amounts of data training weren’t necessarily leading to improvements, but that efficiencies and fine-tuning of smaller models were the key.
Princeton computer science professor Arvind Narayanan wrote last week that the popular view that model scaling is on a path toward AGI “rests on a series of myths and misconceptions,” and that there’s virtually no chance that this scaling alone will lead to AGI.
For enterprise leaders, this plateauing has significant implications. It means they should be leveraging the best individual LLMs for their specific purposes — and there are now hundreds of these LLMs available. There’s no particular “magical unicorn” LLM that will rule them all. As they consider their LLM choices, enterprises should consider open LLMs, like those based on Meta’s Llama or IBM’s Granite, which offer more control and allow for easier fine-tuning to specific use cases.
At VB Transform, we’ll dive deeper into these dynamics with key speakers including Olivier Godement, Head of Product API at OpenAI; Jared Kaplan, Chief Scientist and Co-Founder of Anthropic; Colette Stallbaumer, Copilot GM at Microsoft; David Cox, VP of AI Models at IBM; and Yasmeen Ahmad, Managing Director at Google Cloud.
As the pace of LLM breakthroughs slows, a larger question emerges: Have we reached the peak of inflated expectations in the AGI hype cycle?
Our answer: Yes.
This matters because companies should focus on leveraging existing AI capabilities for real-world applications, rather than chasing the promise of AGI.
ChatGPT’s release unleashed a wave of excitement about the possibilities of AI. Its human-like interactions, powered by massive amounts of training data, gave the illusion of true intelligence. This breakthrough catapulted Altman to guru status in the tech world.
Altman embraced this role, making grandiose pronouncements about the future of AI. In November 2023, upon releasing GPT-4 Turbo, he claimed it would look “quaint” compared to what they were developing. He referred to AGI as possible in the next few years. These statements sparked massive enthusiasm among what we might call the spellbound zealots of Silicon Valley.
However, the spell began to wear off. Altman’s ejection from OpenAI’s board in late 2023 (albeit temporary) was the first crack in the armor. As we entered 2024, his professions that AGI was close began to seem less convincing — he tempered his predictions, emphasizing the need for further breakthroughs. In February, Altman said AGI might require up to $7 trillion of investment.
Competitors narrowed the gap with OpenAI’s leading LLM, and the steady improvements many had predicted failed to materialize. The cost of feeding more data to these models has increased, while their frequent logical errors and hallucinations persist. This has led experts like Yann LeCun, chief scientist at Meta, and others to argue that LLMs are a massive distraction and an “off-ramp” from true AGI. LeCun contends that while LLMs are impressive in their ability to process and generate human-like text, they lack the fundamental understanding and reasoning capabilities that would be required for AGI.
That’s not to say the hype has completely dissipated. The AI fever continues in some Silicon Valley circles, exemplified by the recent passionate four-hour video from Leopold Aschenbrenner, a former OpenAI employee, arguing that AGI could arrive within three years.
But many seasoned observers, including Princeton’s Narayanan, point to serious flaws in such arguments. It’s this more grounded perspective that most enterprise companies should adopt.
In conversations with enterprise AI leaders — from companies like Honeywell, Kaiser Permanente, Chevron and Verizon — I’ve consistently heard that the reality of AI implementation is much more complex and nuanced than the hype would suggest.
While leaders are still enthusiastic about its potential, it’s important not to get carried away with the idea that AI is improving so quickly that the next generation of the technology will solve the problems of the existing generation, says Steve Jones, EVP of CapGemini, a company that helps companies adopt AI. You’ve got to put in the controls now to harness it well: “Whether it’s 20% or 50% of decisions will be made by AI in the next five years. It doesn’t matter,” he said in an interview with VentureBeat. The point is that your career success is based on the success of that algorithm, he says, and your organization is depending on you to understand how it works, and ensuring that it works well.
“There’s all the nonsense around AGI that’s going on,” he said referring to the continued hype among Silicon Valley developers who aren’t really focused on enterprise deployments. But AI is “more of an organizational change than a technological one,” he said, adding that companies need to harness and control the real, basic advancements LLMs already provide.
Large companies are letting model providers do the heavy lifting of training, while they focus on fine-tuning models for their specific purposes. This more pragmatic approach is echoed by leaders across the finance, health and retail sectors we’ve been tracking.
For instance, at JPMorgan Chase, Citi, Wells Fargo, and other banks I’ve talked with, the focus is on using AI to enhance specific banking functions, leading to practical applications in fraud detection, risk management and customer service.
In healthcare, Dr. Ashley Beecy, medical director of AI operations at the NewYork-Presbyterian hospital system, provides another example of how big visions are being anchored instead by practical applications of AI. While she envisions an AI that knows everything about a patient, she says the hospital is starting with more practical applications like reducing the administrative burden on doctors by recording and transcribing patient visits.
Beecy notes that much of the technical capability for the more ambitious version of AI is in place, but it’s a matter of adjusting internal workflows and processes to allow this to happen, or what she called “change management.” This will take a lot of work and testing, she acknowledged, and also require the sharing of ideas by national health organizations, since it will require larger structural change beyond her own hospital.
At VB Transform, we’ll explore this tension between AGI hype and practical reality with speakers from across the industry spectrum, providing attendees with a clear-eyed view of where AI capabilities truly stand and how they can be leveraged effectively in the enterprise. Speakers like Jared Kaplan, Chief Scientist at Anthropic, will discuss the current state of AI capabilities and the challenges ahead. We’ll also hear from enterprise leaders who are successfully navigating this post-hype landscape, including Nhung Ho from Intuit and Bill Braun, CIO of Chevron.
Is there a GPU bottleneck hurting the scaling of GenAI? Our answer: Yes, but it’s more nuanced than headlines suggest.
Why it matters: Enterprise companies need to strategically plan their AI infrastructure investments, balancing immediate needs with long-term scalability.
The surge in AI development has led to an unprecedented demand for specialized hardware, particularly GPUs (Graphics Processing Units), that help run AI applications. Nvidia, the leading GPU manufacturer, has seen its market value skyrocket above $3 trillion, becoming the world’s most valuable companies. This demand has created a supply crunch, driving up costs and extending wait times for this critical AI infrastructure.
However, the bottleneck isn’t uniform across all AI applications. While training large models requires immense computational power, many enterprise use cases focus on inference – running pre-trained models to generate outputs. For these applications, the hardware requirements can be less demanding.
Jonathan Ross, CEO of Groq, a company developing innovative AI chips, argues that inference can be run efficiently on non-GPU hardware. Groq’s language processing units (LPUs) promise significant performance gains for certain AI tasks. Other startups are also entering this space, challenging Nvidia’s dominance and potentially alleviating the GPU bottleneck.
Despite these developments, the overall trend points towards increasing computational demands. AI labs and hyperscale cloud companies that are training advanced models and want to stay leaders are building massive data centers, with some joining what I call the “500K GPU club.” This arms race is spurring interest in alternative technologies like quantum computing, photonics, and even synthetic DNA for data storage to support AI scaling.
However, most enterprise companies don’t find themselves as constrained by GPU availability. Most will just use Azure, AWS and Google’s GCP clouds, letting those big players sweat the costs of the GPU buildout.
Take Intuit, one of the first companies to seriously embrace generative AI last year. The company’s VP of AI, Nhung Ho, told me last week that the company doesn’t need the latest GPUs for its work. “There are a lot of older GPUs that work just fine,” Ho said. “We’re using six or seven-year-old technology… it works beautifully.” This suggests that for many enterprise applications, creative solutions and efficient architectures can mitigate the hardware bottleneck.
At VB Transform, we’ll delve deeper into these infrastructure challenges. Speakers like Groq’s Jonathan Ross, Nvidia’s Nik Spirin, IBM’s director of Quantum Algorithms, Jamie Garcia, and HPE’s Chief Architect Kirk Bresniker will discuss the evolving AI hardware landscape. We’ll also hear from cloud providers like AWS, who are working on software optimizations to maximize existing hardware capabilities.
Is all content on the web free for training LLMs?
Our answer: No, and this presents significant legal and ethical challenges.
Why it matters: Enterprise companies need to be aware of potential copyright and privacy issues when deploying AI models, as the legal landscape is rapidly evolving.
The data used to train LLMs has become a contentious issue, with major implications for AI developers and enterprise users alike. The New York Times and the Center for Investigative Reporting have filed suits against OpenAI, alleging unauthorized use of its content for training, which is just the tip of the iceberg.
This legal battle highlights a crucial question: Do AI companies have the right to scrape and use online content for training without explicit permission or compensation? The answer is unclear, and legal experts suggest it could take up to a decade for this issue to be fully resolved in the courts.
While many AI companies offer indemnification for enterprises using their services, this doesn’t completely shield businesses from potential legal risks. The situation is further complicated by emerging AI-powered search engines and summarization tools. Perplexity AI, for instance, has faced criticism for summarizing paywalled articles, leading to a complaint from Forbes alleging copyright infringement.
As the founder of VentureBeat, I have a stake in this debate. Our business model, like many publishers, relies on page views and advertising. If AI models can freely summarize our content without driving traffic to our site, it threatens our ability to monetize our work. This isn’t just a concern for media companies, but any content creator.
Any enterprise using AI models trained on web data could potentially face legal challenges. Businesses must understand the provenance of the data used to train the AI models they deploy. This is also key for finance and banking companies, which face big regulations around privacy and the usage of personal information.
Some companies are taking proactive steps to address these concerns. On the training side, OpenAI is racing to strike deals with publishers and other companies. Apple has reportedly struck deals with news publishers to use their content for AI training. This could set a precedent for how AI companies and content creators collaborate in the future.
At VB Transform, we’ll explore these legal complexities in depth. Aravind Srinivas, CEO of Perplexity AI, will share insights on navigating these challenges. We’ll also hear from enterprise leaders on how they’re approaching these issues in their AI strategies.
Are gen AI applications disrupting the core offerings of most enterprise companies?
Our answer: No, not yet.
Why this is important: While AI is transformative, its impact is currently more pronounced in enhancing existing processes rather than revolutionizing core business models.
The narrative surrounding AI often suggests an imminent, wholesale disruption of enterprise operations. However, the reality on the ground tells a different story. Most companies are finding success by applying AI to peripheral functions rather than completely overhauling their core offerings.
Common applications include:
These applications are driving significant productivity gains and operational efficiencies. However, they’re not yet leading to the massive revenue gains or business model shifts that some predicted.
Executives at retail companies like Albertsons and AB InBev have told me they are eagerly looking for ways to impact their core, experimenting with “large application models” to predict customer purchasing patterns. In the pharmaceutical industry, there’s hope that AI could accelerate drug discovery, though progress has been slower than many realize.
Intuit provides an interesting case study here as well. Its business, based on tax and business code and terminology, is closer to the powerful language applications that LLMs provide, which explains why Intuit leaped ahead quickly, announcing its own Generative AI Operating System (GenOS) a year ago. It integrates AI assistants across products like TurboTax, QuickBooks, and Mailchimp. Still, its AI usage is focused on customer help, similar to what everyone else is using AI for.
Apple’s perspective is telling. They view AI as a feature, not a product – at least for now. This stance reflects the current state of AI in many enterprises: a powerful tool for enhancement rather than a standalone revolution.
Caroline Arnold, an executive vice president of StateStreet, a major Boston-based bank, exemplifies this sentiment that generative AI is about productivity gains, but not a core revenue driver. At our Boston event in March, she highlighted AI’s potential: “What gen AI allows you to do is to interact in a very natural way with large amounts of data, on the fly, and build scenarios… in a way that would take you much more time in a traditional way.”
While the bank’s new LLM-infused chatbot quickly outperformed the existing helpdesk, it wasn’t without challenges. The chatbot occasionally offered “weird answers,” requiring fine-tuning. Four months later, State Street has yet to release its apps publicly, underscoring the complexities of enterprise generative AI adoption even at the edges.
At VB Transform, we’ll explore this nuanced reality with speakers like Nhung Ho, VP of AI at Intuit, and Bill Braun, CIO of Chevron, Daniel Yang, VP of AI for Kaiser Permanente, Desirée Gosby, VP of Walmart, and Christian Mitchell, EVP of Northwestern. They’ll share insights on how they’re integrating AI into their operations and where they see the most significant impacts.
Are AI agents going to be the future of AI?
Our answer: Yes, but with caveats.
Why does this matter? AI agents represent a potential leap forward in automation and decision-making, but their current capabilities are often overstated.
The concept of AI agents – autonomous systems that can perform tasks or make decisions with minimal human intervention – has captured the imagination of many in the tech world. Some, like former OpenAI employee Leopold Aschenbrenner, envision a not-to-distant future where hundreds of millions of AGI-smart AI agents run various aspects of our world. This, in turn, would squeeze a decade of algorithmic progress into a year or less: “We would rapidly go from human-level to vastly superhuman AI systems,” he argues.
However, most people I’ve talked with believe this is a pipe dream. The current state of AI agents is, in fact, far more modest than Silicon Valley enthusiasts even assumed they would be just a year ago, when excitement exploded around Auto-GPT, an agent framework that would supposedly allow you to do all kinds of things, including starting your own company. While there are promising use cases in areas like customer service and marketing automation, fully autonomous AI agents are still in their infancy, and face many challenges of staying on track with their jobs.
Other emerging applications of AI agents include:
These agents often use a lead LLM to orchestrate the process, with sub-agents handling specific tasks like web searches or payments. However, they’re far from the general-purpose, fully autonomous systems some envision.
Intuit’s approach to AI agents is instructive. Nhung Ho revealed that while Intuit has built out infrastructure to support agentic frameworks, it has paused investments in that area. Intuit is waiting for the technology to mature before fully integrating it into their products.
This cautious approach reflects the broader industry sentiment. While AI agents show promise, they’re not yet reliable or versatile enough for widespread enterprise adoption in critical roles.
At VB Transform, we’ll explore the current state and future potential of AI agents. Speakers like Itamar Friedman, CEO of Codium AI, which is developing an autonomous coding agent, and Jerry Liu, CEO of LlamaIndex, will share their insights on this emerging technology.
As we’ve explored the six critical AI debates shaping enterprise strategy in 2024, a clear theme emerges: the shift from hype to practical implementation. The key takeaways for enterprise leaders:
The real AI revolution isn’t happening in research labs pursuing AGI, but in offices worldwide where AI is being integrated into everyday operations. As Steve Jones of Capgemini said, “AI is more of an organizational change than a technological change.”
As we head toward VB Transform and into the second half of the year, remember that the most valuable AI implementation might not make headlines. It might be the one that saves your customer service team a few hours each day or helps your developers catch bugs more quickly. The question is no longer “Will AI change everything?” but “How can we harness AI to do what we do, better?” That’s what will separate the AI leaders from the laggards in the years to come.
And that’s the conversion I believe will dominate at VB Transform.
We want to hear from you! Take our quick AI survey and share your insights on the current state of AI, how you’re implementing it, and what you expect to see in the future. Learn More
As I’ve been organizing VB Transform, our event next week focused on enterprise generative AI, I’ve noticed a stark shift in the scores of conversations I’m having with tech leaders. A year ago, it was all about how to embrace the magic of OpenAI’s GPT-4 throughout the company. Now their focus is on practical implementation and ROI. It’s as if the entire industry has hit reality mode.
As we enter the second half of 2024, the artificial intelligence landscape is undergoing a seismic shift. The initial excitement following OpenAI’s release of ChatGPT — which became the fastest product in history to attract 100 million users — has begun to wane. We’re moving from an era of near unbridled hype to one of reality, where enterprises are grappling with how to implement AI technologies in real products.
OpenAI CEO Sam Altman’s pronouncements of “magic intelligence in the sky” sparked a frenzy among Silicon Valley developers, many of whom came to believe we were on the cusp of achieving human-level machine intelligence across all domains, known as artificial general intelligence (AGI).
However, as 2024 progresses, a more nuanced narrative is emerging. Enterprises grounded in the practicalities of implementing AI in real-world applications are taking a more measured approach. The realization is setting in that while large language models (LLMs) like GPT-4o are incredibly powerful, generative AI overall has not lived up to Silicon Valley’s lofty expectations. LLM performance has plateaued, facing persistent challenges with factual accuracy. Legal and ethical concerns abound, and infrastructure and business use cases have proved more challenging than anticipated. We’re clearly not on a direct path to AGI as some had hoped. Even more modest promises, like autonomous AI agents, face plenty of limitations. And conservative technologies meant to “ground” AI with real data and accuracy, like RAG (retrieval-augmented generation), still have massive hurdles. Basically, LLMs still hallucinate like crazy.
Countdown to VB Transform 2024
Join enterprise leaders in San Francisco from July 9 to 11 for our flagship AI event. Connect with peers, explore the opportunities and challenges of Generative AI, and learn how to integrate AI applications into your industry. Register Now
Instead, companies are focusing on how to leverage the impressive basic capabilities of LLMs already available. This shift from hype to reality is underscored by six critical debates that are shaping the AI landscape. These debates represent fault lines between the zealous believers in imminent superintelligence and those advocating for a more pragmatic approach to AI adoption. For enterprise leaders, understanding these debates is crucial. There are significant stakes for companies looking to exploit this powerful technology, even if it’s not the godlike force its most ardent proponents claim.
Don’t read this wrong. Most enterprise leaders still believe that the technology has already produced profound benefits. During our recent AI Impact Tour, where meetings and events were held with Fortune 500 companies across the country, leaders openly discussed their efforts to embrace AI’s promise.
But these six debates will be central to discussions at our upcoming VB Transform event, scheduled for July 9-11 in the heart of San Francisco’s SOMA district. We’ve curated the event based on extensive conversations with executives from the largest players in AI.
The speaker lineup includes representatives from industry giants like OpenAI, Anthropic, Nvidia, Microsoft, Google, and Amazon, as well as AI leaders from Fortune 500 companies such as Kaiser Permanente, Walmart, and Bank of America.
The live debates and discussions at Transform promise to shed light on these critical issues, offering in-person attendees a unique opportunity to engage with leaders at the forefront of enterprise AI implementation.
Now, let’s dive into the six debates:
1. The LLM race: a plateau in sight?
The race to develop the most advanced LLM has been a defining feature of the AI landscape since OpenAI’s GPT-3 burst onto the scene. But as we enter the second half of 2024, a question looms large: Is the LLM race over?
The answer appears to be yes, at least for now.
This matters because the differences between leading LLMs have become increasingly imperceptible, meaning enterprise companies can now select based on price, efficiency, and specific use-case fit rather than chasing the “best” model.
In 2023, we witnessed a dramatic race unfold. OpenAI surged ahead with the release of GPT-4 in March, showcasing significant improvements in reasoning, multi-modal capabilities, and multilingual proficiency. Pundits assumed that performance would continue to scale as more data was fed into these models. For a while, it looked like they were right.
But in 2024, the pace has slowed considerably. Despite vague promises from Altman suggesting more delights were coming, the company’s COO Mira Murati admitted in mid-June that OpenAI doesn’t have anything more in its labs than what is already public.
Now, we’re seeing clear signs of plateauing. OpenAI appears to have hit a wall, and its rival Anthropic has caught up, launching Claude 3.5 Sonnet, which outperforms GPT-4 on many measures. What’s notable is that Claude wasn’t able to leap far ahead; it’s only incrementally better. More tellingly, Sonnet is based on one of Anthropic’s smaller models, not its larger Opus model – suggesting that massive amounts of data training weren’t necessarily leading to improvements, but that efficiencies and fine-tuning of smaller models were the key.
Princeton computer science professor Arvind Narayanan wrote last week that the popular view that model scaling is on a path toward AGI “rests on a series of myths and misconceptions,” and that there’s virtually no chance that this scaling alone will lead to AGI.
For enterprise leaders, this plateauing has significant implications. It means they should be leveraging the best individual LLMs for their specific purposes — and there are now hundreds of these LLMs available. There’s no particular “magical unicorn” LLM that will rule them all. As they consider their LLM choices, enterprises should consider open LLMs, like those based on Meta’s Llama or IBM’s Granite, which offer more control and allow for easier fine-tuning to specific use cases.
At VB Transform, we’ll dive deeper into these dynamics with key speakers including Olivier Godement, Head of Product API at OpenAI; Jared Kaplan, Chief Scientist and Co-Founder of Anthropic; Colette Stallbaumer, Copilot GM at Microsoft; David Cox, VP of AI Models at IBM; and Yasmeen Ahmad, Managing Director at Google Cloud.
2. The AGI hype cycle: peak or trough?
As the pace of LLM breakthroughs slows, a larger question emerges: Have we reached the peak of inflated expectations in the AGI hype cycle?
Our answer: Yes.
This matters because companies should focus on leveraging existing AI capabilities for real-world applications, rather than chasing the promise of AGI.
ChatGPT’s release unleashed a wave of excitement about the possibilities of AI. Its human-like interactions, powered by massive amounts of training data, gave the illusion of true intelligence. This breakthrough catapulted Altman to guru status in the tech world.
Altman embraced this role, making grandiose pronouncements about the future of AI. In November 2023, upon releasing GPT-4 Turbo, he claimed it would look “quaint” compared to what they were developing. He referred to AGI as possible in the next few years. These statements sparked massive enthusiasm among what we might call the spellbound zealots of Silicon Valley.
However, the spell began to wear off. Altman’s ejection from OpenAI’s board in late 2023 (albeit temporary) was the first crack in the armor. As we entered 2024, his professions that AGI was close began to seem less convincing — he tempered his predictions, emphasizing the need for further breakthroughs. In February, Altman said AGI might require up to $7 trillion of investment.
Competitors narrowed the gap with OpenAI’s leading LLM, and the steady improvements many had predicted failed to materialize. The cost of feeding more data to these models has increased, while their frequent logical errors and hallucinations persist. This has led experts like Yann LeCun, chief scientist at Meta, and others to argue that LLMs are a massive distraction and an “off-ramp” from true AGI. LeCun contends that while LLMs are impressive in their ability to process and generate human-like text, they lack the fundamental understanding and reasoning capabilities that would be required for AGI.
That’s not to say the hype has completely dissipated. The AI fever continues in some Silicon Valley circles, exemplified by the recent passionate four-hour video from Leopold Aschenbrenner, a former OpenAI employee, arguing that AGI could arrive within three years.
But many seasoned observers, including Princeton’s Narayanan, point to serious flaws in such arguments. It’s this more grounded perspective that most enterprise companies should adopt.
In conversations with enterprise AI leaders — from companies like Honeywell, Kaiser Permanente, Chevron and Verizon — I’ve consistently heard that the reality of AI implementation is much more complex and nuanced than the hype would suggest.
While leaders are still enthusiastic about its potential, it’s important not to get carried away with the idea that AI is improving so quickly that the next generation of the technology will solve the problems of the existing generation, says Steve Jones, EVP of CapGemini, a company that helps companies adopt AI. You’ve got to put in the controls now to harness it well: “Whether it’s 20% or 50% of decisions will be made by AI in the next five years. It doesn’t matter,” he said in an interview with VentureBeat. The point is that your career success is based on the success of that algorithm, he says, and your organization is depending on you to understand how it works, and ensuring that it works well.
“There’s all the nonsense around AGI that’s going on,” he said referring to the continued hype among Silicon Valley developers who aren’t really focused on enterprise deployments. But AI is “more of an organizational change than a technological one,” he said, adding that companies need to harness and control the real, basic advancements LLMs already provide.
Large companies are letting model providers do the heavy lifting of training, while they focus on fine-tuning models for their specific purposes. This more pragmatic approach is echoed by leaders across the finance, health and retail sectors we’ve been tracking.
For instance, at JPMorgan Chase, Citi, Wells Fargo, and other banks I’ve talked with, the focus is on using AI to enhance specific banking functions, leading to practical applications in fraud detection, risk management and customer service.
In healthcare, Dr. Ashley Beecy, medical director of AI operations at the NewYork-Presbyterian hospital system, provides another example of how big visions are being anchored instead by practical applications of AI. While she envisions an AI that knows everything about a patient, she says the hospital is starting with more practical applications like reducing the administrative burden on doctors by recording and transcribing patient visits.
Beecy notes that much of the technical capability for the more ambitious version of AI is in place, but it’s a matter of adjusting internal workflows and processes to allow this to happen, or what she called “change management.” This will take a lot of work and testing, she acknowledged, and also require the sharing of ideas by national health organizations, since it will require larger structural change beyond her own hospital.
At VB Transform, we’ll explore this tension between AGI hype and practical reality with speakers from across the industry spectrum, providing attendees with a clear-eyed view of where AI capabilities truly stand and how they can be leveraged effectively in the enterprise. Speakers like Jared Kaplan, Chief Scientist at Anthropic, will discuss the current state of AI capabilities and the challenges ahead. We’ll also hear from enterprise leaders who are successfully navigating this post-hype landscape, including Nhung Ho from Intuit and Bill Braun, CIO of Chevron.
3. The GPU bottleneck: infrastructure realities
Is there a GPU bottleneck hurting the scaling of GenAI? Our answer: Yes, but it’s more nuanced than headlines suggest.
Why it matters: Enterprise companies need to strategically plan their AI infrastructure investments, balancing immediate needs with long-term scalability.
The surge in AI development has led to an unprecedented demand for specialized hardware, particularly GPUs (Graphics Processing Units), that help run AI applications. Nvidia, the leading GPU manufacturer, has seen its market value skyrocket above $3 trillion, becoming the world’s most valuable companies. This demand has created a supply crunch, driving up costs and extending wait times for this critical AI infrastructure.
However, the bottleneck isn’t uniform across all AI applications. While training large models requires immense computational power, many enterprise use cases focus on inference – running pre-trained models to generate outputs. For these applications, the hardware requirements can be less demanding.
Jonathan Ross, CEO of Groq, a company developing innovative AI chips, argues that inference can be run efficiently on non-GPU hardware. Groq’s language processing units (LPUs) promise significant performance gains for certain AI tasks. Other startups are also entering this space, challenging Nvidia’s dominance and potentially alleviating the GPU bottleneck.
Despite these developments, the overall trend points towards increasing computational demands. AI labs and hyperscale cloud companies that are training advanced models and want to stay leaders are building massive data centers, with some joining what I call the “500K GPU club.” This arms race is spurring interest in alternative technologies like quantum computing, photonics, and even synthetic DNA for data storage to support AI scaling.
However, most enterprise companies don’t find themselves as constrained by GPU availability. Most will just use Azure, AWS and Google’s GCP clouds, letting those big players sweat the costs of the GPU buildout.
Take Intuit, one of the first companies to seriously embrace generative AI last year. The company’s VP of AI, Nhung Ho, told me last week that the company doesn’t need the latest GPUs for its work. “There are a lot of older GPUs that work just fine,” Ho said. “We’re using six or seven-year-old technology… it works beautifully.” This suggests that for many enterprise applications, creative solutions and efficient architectures can mitigate the hardware bottleneck.
At VB Transform, we’ll delve deeper into these infrastructure challenges. Speakers like Groq’s Jonathan Ross, Nvidia’s Nik Spirin, IBM’s director of Quantum Algorithms, Jamie Garcia, and HPE’s Chief Architect Kirk Bresniker will discuss the evolving AI hardware landscape. We’ll also hear from cloud providers like AWS, who are working on software optimizations to maximize existing hardware capabilities.
4. Content rights and LLM training: legal landmines ahead
Is all content on the web free for training LLMs?
Our answer: No, and this presents significant legal and ethical challenges.
Why it matters: Enterprise companies need to be aware of potential copyright and privacy issues when deploying AI models, as the legal landscape is rapidly evolving.
The data used to train LLMs has become a contentious issue, with major implications for AI developers and enterprise users alike. The New York Times and the Center for Investigative Reporting have filed suits against OpenAI, alleging unauthorized use of its content for training, which is just the tip of the iceberg.
This legal battle highlights a crucial question: Do AI companies have the right to scrape and use online content for training without explicit permission or compensation? The answer is unclear, and legal experts suggest it could take up to a decade for this issue to be fully resolved in the courts.
While many AI companies offer indemnification for enterprises using their services, this doesn’t completely shield businesses from potential legal risks. The situation is further complicated by emerging AI-powered search engines and summarization tools. Perplexity AI, for instance, has faced criticism for summarizing paywalled articles, leading to a complaint from Forbes alleging copyright infringement.
As the founder of VentureBeat, I have a stake in this debate. Our business model, like many publishers, relies on page views and advertising. If AI models can freely summarize our content without driving traffic to our site, it threatens our ability to monetize our work. This isn’t just a concern for media companies, but any content creator.
Any enterprise using AI models trained on web data could potentially face legal challenges. Businesses must understand the provenance of the data used to train the AI models they deploy. This is also key for finance and banking companies, which face big regulations around privacy and the usage of personal information.
Some companies are taking proactive steps to address these concerns. On the training side, OpenAI is racing to strike deals with publishers and other companies. Apple has reportedly struck deals with news publishers to use their content for AI training. This could set a precedent for how AI companies and content creators collaborate in the future.
At VB Transform, we’ll explore these legal complexities in depth. Aravind Srinivas, CEO of Perplexity AI, will share insights on navigating these challenges. We’ll also hear from enterprise leaders on how they’re approaching these issues in their AI strategies.
5. Gen AI applications: transforming edges, not cores
Are gen AI applications disrupting the core offerings of most enterprise companies?
Our answer: No, not yet.
Why this is important: While AI is transformative, its impact is currently more pronounced in enhancing existing processes rather than revolutionizing core business models.
The narrative surrounding AI often suggests an imminent, wholesale disruption of enterprise operations. However, the reality on the ground tells a different story. Most companies are finding success by applying AI to peripheral functions rather than completely overhauling their core offerings.
Common applications include:
- Customer support chatbots
- Knowledge base assistants for employees
- Generative marketing materials
- Code generation and debugging tools
These applications are driving significant productivity gains and operational efficiencies. However, they’re not yet leading to the massive revenue gains or business model shifts that some predicted.
Executives at retail companies like Albertsons and AB InBev have told me they are eagerly looking for ways to impact their core, experimenting with “large application models” to predict customer purchasing patterns. In the pharmaceutical industry, there’s hope that AI could accelerate drug discovery, though progress has been slower than many realize.
Intuit provides an interesting case study here as well. Its business, based on tax and business code and terminology, is closer to the powerful language applications that LLMs provide, which explains why Intuit leaped ahead quickly, announcing its own Generative AI Operating System (GenOS) a year ago. It integrates AI assistants across products like TurboTax, QuickBooks, and Mailchimp. Still, its AI usage is focused on customer help, similar to what everyone else is using AI for.
Apple’s perspective is telling. They view AI as a feature, not a product – at least for now. This stance reflects the current state of AI in many enterprises: a powerful tool for enhancement rather than a standalone revolution.
Caroline Arnold, an executive vice president of StateStreet, a major Boston-based bank, exemplifies this sentiment that generative AI is about productivity gains, but not a core revenue driver. At our Boston event in March, she highlighted AI’s potential: “What gen AI allows you to do is to interact in a very natural way with large amounts of data, on the fly, and build scenarios… in a way that would take you much more time in a traditional way.”
While the bank’s new LLM-infused chatbot quickly outperformed the existing helpdesk, it wasn’t without challenges. The chatbot occasionally offered “weird answers,” requiring fine-tuning. Four months later, State Street has yet to release its apps publicly, underscoring the complexities of enterprise generative AI adoption even at the edges.
At VB Transform, we’ll explore this nuanced reality with speakers like Nhung Ho, VP of AI at Intuit, and Bill Braun, CIO of Chevron, Daniel Yang, VP of AI for Kaiser Permanente, Desirée Gosby, VP of Walmart, and Christian Mitchell, EVP of Northwestern. They’ll share insights on how they’re integrating AI into their operations and where they see the most significant impacts.
6. AI agents: the next frontier or overblown hype?
Are AI agents going to be the future of AI?
Our answer: Yes, but with caveats.
Why does this matter? AI agents represent a potential leap forward in automation and decision-making, but their current capabilities are often overstated.
The concept of AI agents – autonomous systems that can perform tasks or make decisions with minimal human intervention – has captured the imagination of many in the tech world. Some, like former OpenAI employee Leopold Aschenbrenner, envision a not-to-distant future where hundreds of millions of AGI-smart AI agents run various aspects of our world. This, in turn, would squeeze a decade of algorithmic progress into a year or less: “We would rapidly go from human-level to vastly superhuman AI systems,” he argues.
However, most people I’ve talked with believe this is a pipe dream. The current state of AI agents is, in fact, far more modest than Silicon Valley enthusiasts even assumed they would be just a year ago, when excitement exploded around Auto-GPT, an agent framework that would supposedly allow you to do all kinds of things, including starting your own company. While there are promising use cases in areas like customer service and marketing automation, fully autonomous AI agents are still in their infancy, and face many challenges of staying on track with their jobs.
Other emerging applications of AI agents include:
- Travel planning and booking
- E-commerce product searches and purchases
- Automated coding assistants
- Financial trading algorithms
These agents often use a lead LLM to orchestrate the process, with sub-agents handling specific tasks like web searches or payments. However, they’re far from the general-purpose, fully autonomous systems some envision.
Intuit’s approach to AI agents is instructive. Nhung Ho revealed that while Intuit has built out infrastructure to support agentic frameworks, it has paused investments in that area. Intuit is waiting for the technology to mature before fully integrating it into their products.
This cautious approach reflects the broader industry sentiment. While AI agents show promise, they’re not yet reliable or versatile enough for widespread enterprise adoption in critical roles.
At VB Transform, we’ll explore the current state and future potential of AI agents. Speakers like Itamar Friedman, CEO of Codium AI, which is developing an autonomous coding agent, and Jerry Liu, CEO of LlamaIndex, will share their insights on this emerging technology.
Conclusion: Navigating the AI landscape in 2024 and beyond
As we’ve explored the six critical AI debates shaping enterprise strategy in 2024, a clear theme emerges: the shift from hype to practical implementation. The key takeaways for enterprise leaders:
- The LLM race has plateaued: Focus on selecting models based on specific use cases, cost-efficiency, and ease of integration rather than chasing the “best” model.
- AGI hype is cooling, practical AI is heating up: The immediate focus should be on leveraging existing AI capabilities for tangible business outcomes.
- Infrastructure challenges require creative solutions: Explore alternative hardware solutions and optimize AI workflows to maximize efficiency on existing hardware.
- Legal and ethical considerations are paramount: Carefully vet AI providers and understand the provenance of their training data to mitigate legal risks.
- Focus on enhancing core functions, not replacing them: Look for opportunities to integrate AI into customer support, employee assistance, and operational efficiency improvements.
- AI Agents show promise, but aren’t ready for prime time: Build out the infrastructure to support agentic frameworks, but be prepared to wait for the technology to mature before full implementation.
The real AI revolution isn’t happening in research labs pursuing AGI, but in offices worldwide where AI is being integrated into everyday operations. As Steve Jones of Capgemini said, “AI is more of an organizational change than a technological change.”
As we head toward VB Transform and into the second half of the year, remember that the most valuable AI implementation might not make headlines. It might be the one that saves your customer service team a few hours each day or helps your developers catch bugs more quickly. The question is no longer “Will AI change everything?” but “How can we harness AI to do what we do, better?” That’s what will separate the AI leaders from the laggards in the years to come.
And that’s the conversion I believe will dominate at VB Transform.
Author: Matt Marshall
Source: Venturebeat
Reviewed By: Editorial Team