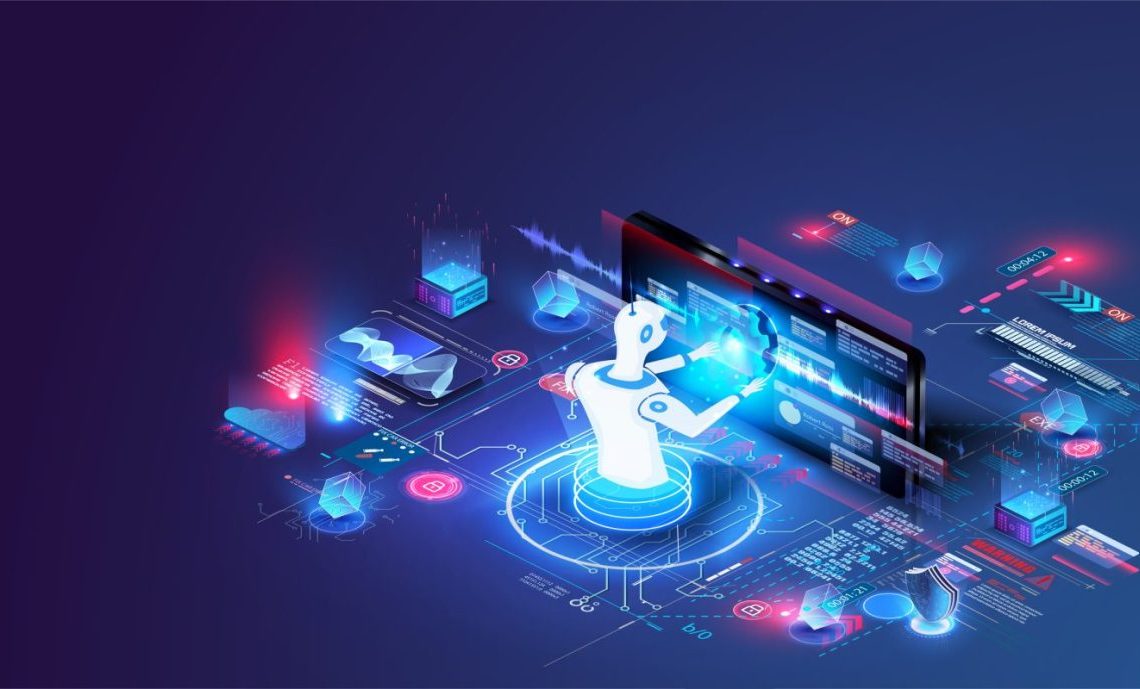
As the demand for AI continues to grow, a new category of tools helping with development and deployment is coming to the scene. Case in point: RagaAI, a California-based startup offering a platform to test and fix AI, today emerged from stealth with $4.7 million seed funding from pi Ventures. Global investors Anorak Ventures, TenOneTen Ventures, Arka Ventures, Mana Ventures and Exfinity Venture Partners also participated in the round.
Founded by former Nvidia executive Gaurav Agarwal, RagaAI will use the capital to advance research and strengthen its automated testing platform to establish a robust framework for safe and reliable AI.
“Guided by our core values, we are committed to pushing the boundaries of automated AI issue detection, automated root cause analysis and fixing the issues, staying at the forefront of cutting-edge methods,” Agarwal said in a statement. He noted the company is already serving Fortune 500 companies to address issues such as bias, accuracy and hallucinations in different use cases.
Building and deploying AI into production is not a walk in the park. Teams have to gather data, train the models and then be vigilant about how they work in production to see if they are delivering what’s expected — or veering off track into uncharted territories. A small gap here or there and the whole effort comes crashing down, leading to high costs and missed opportunities.
Agarwal saw this problem firsthand when working with Nvidia and Indian mobility company Ola. He decided to tackle it with an automated testing platform that could detect AI issues, diagnose them and fix them on the fly. This led him to build RagaAI. But, here’s the interesting part: the platform does not check for a few dozen issues. It carries out as many as 300 tests, covering all sorts of problems that can lead an AI model to fail, right from data and model issues to operational gaps.
Once the platform identifies a problem, it helps users triage the issue to its root cause. This can be as varied as bias in the training data, poor labeling, data drift, poor hyperparameter optimization while training or a lack of model robustness to adversarial attacks. Then, as the last step, it provides actionable recommendations to fix the issue, like helping teams remove poorly labeled data points in one click or suggesting retraining the model to fix issues with data and concept drift.
At its core lies RagaDNA foundation models that generate high-quality embedding – representations of data in a compressed and meaningful format. Most tests on the platform use these embeddings as a basis for issue detection, diagnosis and remediation.
“RagaAI DNA represents vertical specific foundational models which are custom trained for testing purposes. This allows RagaAI to automatically add intelligence to the testing workflows like defining the Operational Design Domain (ODD), identify edge cases where to model performs poorly or correlate it with missing or poor-quality training data,” Jigar Gupta, the head of product at RagaAI, writes in a blog post.
While the testing platform has just launched publicly, RagaAI claims that several Fortune 500 companies are already using the technology, including AI-first companies such as LightMetrics and SatSure. In one case of implementation, an e-commerce company was able to identify hallucinations and reduce errors in its chatbot. In another, an automotive company was able to improve the accuracy of its model aimed at detecting vehicles in low-light scenarios.
Broadly, RagaAI believes that the technology can reduce 90% of the risks in AI development while accelerating the time to production by more than three times. With this funding, it plans to advance its research and development efforts and improve the testing and remediation platform. It also plans to expand its team and raise awareness about the importance of developing safe and transparent AI.
However, it is important to note that the company is not the only one working to streamline AI deployment. Over the last year, several players have cropped up with the mission to accelerate the safe deployment of AI, including Arize’s Pheonix open-source library, Context AI and Braintrust Data. Many observability players, including Acceldata, are also looking at generative AI monitoring to help teams with deployment.
Given that AI is expected to become a $2 trillion opportunity by 2030, this number is only expected to grow. Raga believes as much as 25% of this will go towards tools ensuring AI is safe and reliable.
As the demand for AI continues to grow, a new category of tools helping with development and deployment is coming to the scene. Case in point: RagaAI, a California-based startup offering a platform to test and fix AI, today emerged from stealth with $4.7 million seed funding from pi Ventures. Global investors Anorak Ventures, TenOneTen Ventures, Arka Ventures, Mana Ventures and Exfinity Venture Partners also participated in the round.
Founded by former Nvidia executive Gaurav Agarwal, RagaAI will use the capital to advance research and strengthen its automated testing platform to establish a robust framework for safe and reliable AI.
“Guided by our core values, we are committed to pushing the boundaries of automated AI issue detection, automated root cause analysis and fixing the issues, staying at the forefront of cutting-edge methods,” Agarwal said in a statement. He noted the company is already serving Fortune 500 companies to address issues such as bias, accuracy and hallucinations in different use cases.
What does RagaAI bring to the table?
Building and deploying AI into production is not a walk in the park. Teams have to gather data, train the models and then be vigilant about how they work in production to see if they are delivering what’s expected — or veering off track into uncharted territories. A small gap here or there and the whole effort comes crashing down, leading to high costs and missed opportunities.
Agarwal saw this problem firsthand when working with Nvidia and Indian mobility company Ola. He decided to tackle it with an automated testing platform that could detect AI issues, diagnose them and fix them on the fly. This led him to build RagaAI. But, here’s the interesting part: the platform does not check for a few dozen issues. It carries out as many as 300 tests, covering all sorts of problems that can lead an AI model to fail, right from data and model issues to operational gaps.
Once the platform identifies a problem, it helps users triage the issue to its root cause. This can be as varied as bias in the training data, poor labeling, data drift, poor hyperparameter optimization while training or a lack of model robustness to adversarial attacks. Then, as the last step, it provides actionable recommendations to fix the issue, like helping teams remove poorly labeled data points in one click or suggesting retraining the model to fix issues with data and concept drift.
At its core lies RagaDNA foundation models that generate high-quality embedding – representations of data in a compressed and meaningful format. Most tests on the platform use these embeddings as a basis for issue detection, diagnosis and remediation.
“RagaAI DNA represents vertical specific foundational models which are custom trained for testing purposes. This allows RagaAI to automatically add intelligence to the testing workflows like defining the Operational Design Domain (ODD), identify edge cases where to model performs poorly or correlate it with missing or poor-quality training data,” Jigar Gupta, the head of product at RagaAI, writes in a blog post.
Significant customer impact
While the testing platform has just launched publicly, RagaAI claims that several Fortune 500 companies are already using the technology, including AI-first companies such as LightMetrics and SatSure. In one case of implementation, an e-commerce company was able to identify hallucinations and reduce errors in its chatbot. In another, an automotive company was able to improve the accuracy of its model aimed at detecting vehicles in low-light scenarios.

Broadly, RagaAI believes that the technology can reduce 90% of the risks in AI development while accelerating the time to production by more than three times. With this funding, it plans to advance its research and development efforts and improve the testing and remediation platform. It also plans to expand its team and raise awareness about the importance of developing safe and transparent AI.
However, it is important to note that the company is not the only one working to streamline AI deployment. Over the last year, several players have cropped up with the mission to accelerate the safe deployment of AI, including Arize’s Pheonix open-source library, Context AI and Braintrust Data. Many observability players, including Acceldata, are also looking at generative AI monitoring to help teams with deployment.
Given that AI is expected to become a $2 trillion opportunity by 2030, this number is only expected to grow. Raga believes as much as 25% of this will go towards tools ensuring AI is safe and reliable.
VentureBeat’s mission is to be a digital town square for technical decision-makers to gain knowledge about transformative enterprise technology and transact. Discover our Briefings.
Author: Shubham Sharma
Source: Venturebeat
Reviewed By: Editorial Team