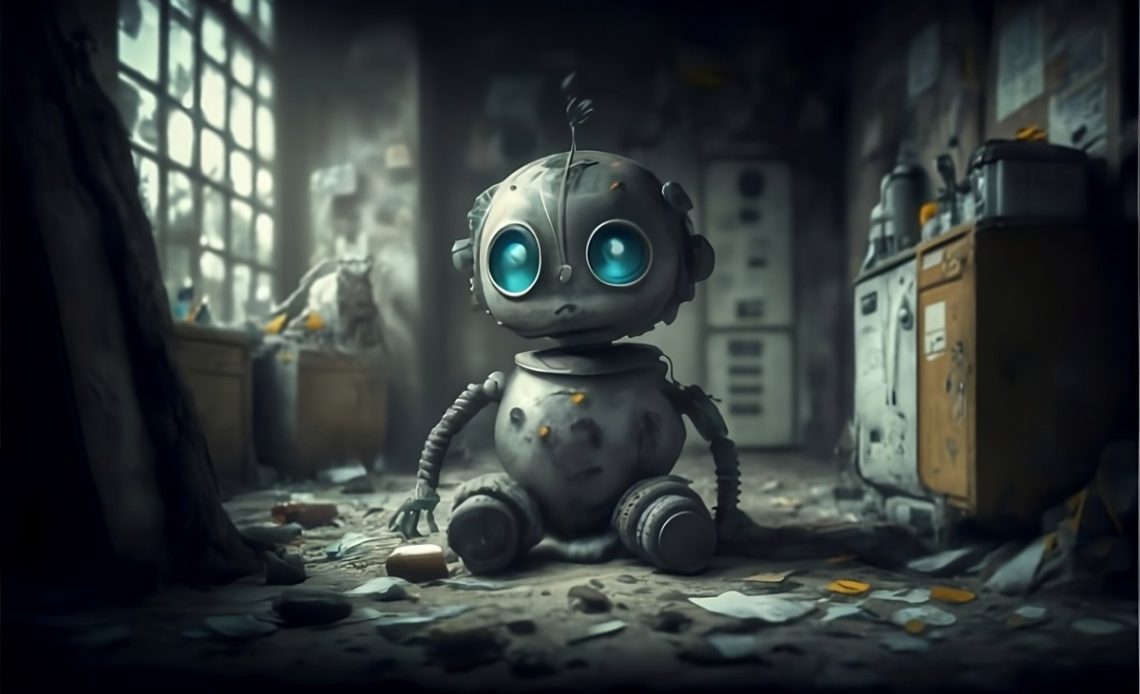
“It’s venture money, not adventure money.” That was the loving response a dear friend once got from a VC while pitching an idea. But when we are in the hype cycle phase of a new technology, that caution goes out the window. VCs, after all, have to deploy all the capital they raised, and the cost of missing out on something big is higher than the downside of swinging and missing, especially when everybody else is taking the same swing.
A similar dynamic plays out inside most companies — and the technology of the moment is AI and anything remotely associated with it. Large language models (LLMs): They are AI. Machine learning (ML): That’s AI. That project you’re told there’s no funding for every year — call it AI and try again.
Billions of dollars will be wasted on AI over the next decade. If that sounds like a contrary take, it shouldn’t. Every big technology wave comes with excitement — even before we know how real and transformative it is. Search, social and mobile have all had a wide and lasting impact, but virtual reality (VR) and crypto have been much more limited.
You wouldn’t know it from reading headlines five years ago, though. Right now, everybody is running to show how much they are spending on AI and how it will change everything. This shotgun approach to investing inevitably results in a few huge hits and many misses. The same dynamic at play for VCs also drives companies’ leadership to greenlight investments in the name of AI that are optimistic, at best, misplaced hope and adventures more often.
That doesn’t take away from the fact that LLMs are a game-changing technology. Just look at how fast ChatGPT reached 100 million users relative to other transformative companies:
Almost every single enterprise company has some work going to leverage LLMs and AI. So, how should you decide where to place your bets and where you have a right to win?
Get clear-eyed about these three things, and you’ll cut out 80% of the wasted spend:
As you think about saying yes to that next AI project, look at the cost of the needed resources, today and over time, to sustain that project. Ten hours of work from your data science team often has 5X the engineering, DevOps, QA, product and SysOps time buried underneath. Companies are littered with fragments of projects that were once a good idea but lacked ongoing investment to sustain them. Saying no to an AI initiative is hard today, but too frequent yes’ often come at the cost of fully funding the few things worth supporting tomorrow.
Another dimension to cost is the increasing marginal cost that AI drives. These large models are costly to train, run and maintain. Overusing AI without a corresponding increase in downstream value chews up your margins. Worse, pulling back released or promised functionality can lead to customer dissatisfaction and negative market perceptions, especially during a hype cycle. Look at how quickly a few missteps have tarnished Google’s reputation as an AI leader, not to mention the early days of IBM’s Watson.
Lessons you learn from textbooks are easy to forget. We have all read about commoditization. The same lesson learned by getting knocked around in real life sticks with you. When I worked as a chip designer at Micron, our core product was close to the perfect commodity — a memory chip. Nobody cares what brand of memory chip is in their laptop, just how much it costs. In that world, scale, and cost are the only sustainable advantages over time.
The tech industry can be bimodal. There are monopolies and commodities. When you say yes to the next AI initiative, ask yourself, “Why us?” Working on something that commoditizes over time is no fun, especially when you don’t have the scale/cost advantage. Take it from me. The only ones who will definitely benefit are Nvidia and AWS/Azure. The only way around this is to focus on something where you have a defensive moat. Preferential access to data, proprietary insights around a use case, or an application with strong network effects where you have a head start.
The simplest bets are the ones that better the business you are already in. The old BASF commercial comes to mind: “We don’t make the things you buy, we make the things you buy better.” If the application of AI provides you momentum in the products you already make, that bet is the easiest to make and scale. The second easiest bets are the ones that let you move up and down the value chain or laterally expand to other sectors.
The most challenging but important bets require you to cannibalize your current business with new technology — if you don’t, someone else will. Double down on the handful of bets that pass these two tests, and be prepared to see those bets through. Leave the rest to the VCs and startups.
So while the hype around AI is real and justified, if there’s one lesson we’ve learned throughout the years, it’s that with these cycles come not only sound investment, but also loads of waste. By following a few tips outlined above, you can make sure that your investments have the best chance at bearing some algorithmic fruit.
Mehul Nagrani is managing director for North America at InMoment.
Join Gen AI enterprise leaders in Boston on March 27 for an exclusive night of networking, insights, and conversations surrounding data integrity. Request an invite here.
“It’s venture money, not adventure money.” That was the loving response a dear friend once got from a VC while pitching an idea. But when we are in the hype cycle phase of a new technology, that caution goes out the window. VCs, after all, have to deploy all the capital they raised, and the cost of missing out on something big is higher than the downside of swinging and missing, especially when everybody else is taking the same swing.
A similar dynamic plays out inside most companies — and the technology of the moment is AI and anything remotely associated with it. Large language models (LLMs): They are AI. Machine learning (ML): That’s AI. That project you’re told there’s no funding for every year — call it AI and try again.
Billions of dollars will be wasted on AI over the next decade. If that sounds like a contrary take, it shouldn’t. Every big technology wave comes with excitement — even before we know how real and transformative it is. Search, social and mobile have all had a wide and lasting impact, but virtual reality (VR) and crypto have been much more limited.
You wouldn’t know it from reading headlines five years ago, though. Right now, everybody is running to show how much they are spending on AI and how it will change everything. This shotgun approach to investing inevitably results in a few huge hits and many misses. The same dynamic at play for VCs also drives companies’ leadership to greenlight investments in the name of AI that are optimistic, at best, misplaced hope and adventures more often.
That doesn’t take away from the fact that LLMs are a game-changing technology. Just look at how fast ChatGPT reached 100 million users relative to other transformative companies:

Almost every single enterprise company has some work going to leverage LLMs and AI. So, how should you decide where to place your bets and where you have a right to win?
Get clear-eyed about these three things, and you’ll cut out 80% of the wasted spend:
- Understand total cost over time;
- Ask why someone else can’t do it;
- Make a few bets you’re willing to follow through.
1: Understand total cost over time
As you think about saying yes to that next AI project, look at the cost of the needed resources, today and over time, to sustain that project. Ten hours of work from your data science team often has 5X the engineering, DevOps, QA, product and SysOps time buried underneath. Companies are littered with fragments of projects that were once a good idea but lacked ongoing investment to sustain them. Saying no to an AI initiative is hard today, but too frequent yes’ often come at the cost of fully funding the few things worth supporting tomorrow.
Another dimension to cost is the increasing marginal cost that AI drives. These large models are costly to train, run and maintain. Overusing AI without a corresponding increase in downstream value chews up your margins. Worse, pulling back released or promised functionality can lead to customer dissatisfaction and negative market perceptions, especially during a hype cycle. Look at how quickly a few missteps have tarnished Google’s reputation as an AI leader, not to mention the early days of IBM’s Watson.
2: Ask why can’t anyone else do this?
Lessons you learn from textbooks are easy to forget. We have all read about commoditization. The same lesson learned by getting knocked around in real life sticks with you. When I worked as a chip designer at Micron, our core product was close to the perfect commodity — a memory chip. Nobody cares what brand of memory chip is in their laptop, just how much it costs. In that world, scale, and cost are the only sustainable advantages over time.
The tech industry can be bimodal. There are monopolies and commodities. When you say yes to the next AI initiative, ask yourself, “Why us?” Working on something that commoditizes over time is no fun, especially when you don’t have the scale/cost advantage. Take it from me. The only ones who will definitely benefit are Nvidia and AWS/Azure. The only way around this is to focus on something where you have a defensive moat. Preferential access to data, proprietary insights around a use case, or an application with strong network effects where you have a head start.
3: Make a few bets you are willing to see through
The simplest bets are the ones that better the business you are already in. The old BASF commercial comes to mind: “We don’t make the things you buy, we make the things you buy better.” If the application of AI provides you momentum in the products you already make, that bet is the easiest to make and scale. The second easiest bets are the ones that let you move up and down the value chain or laterally expand to other sectors.
The most challenging but important bets require you to cannibalize your current business with new technology — if you don’t, someone else will. Double down on the handful of bets that pass these two tests, and be prepared to see those bets through. Leave the rest to the VCs and startups.
So while the hype around AI is real and justified, if there’s one lesson we’ve learned throughout the years, it’s that with these cycles come not only sound investment, but also loads of waste. By following a few tips outlined above, you can make sure that your investments have the best chance at bearing some algorithmic fruit.
Mehul Nagrani is managing director for North America at InMoment.
DataDecisionMakers
Welcome to the VentureBeat community!
DataDecisionMakers is where experts, including the technical people doing data work, can share data-related insights and innovation.
If you want to read about cutting-edge ideas and up-to-date information, best practices, and the future of data and data tech, join us at DataDecisionMakers.
You might even consider contributing an article of your own!
Author: Mehul Nagrani, InMoment
Source: Venturebeat
Reviewed By: Editorial Team