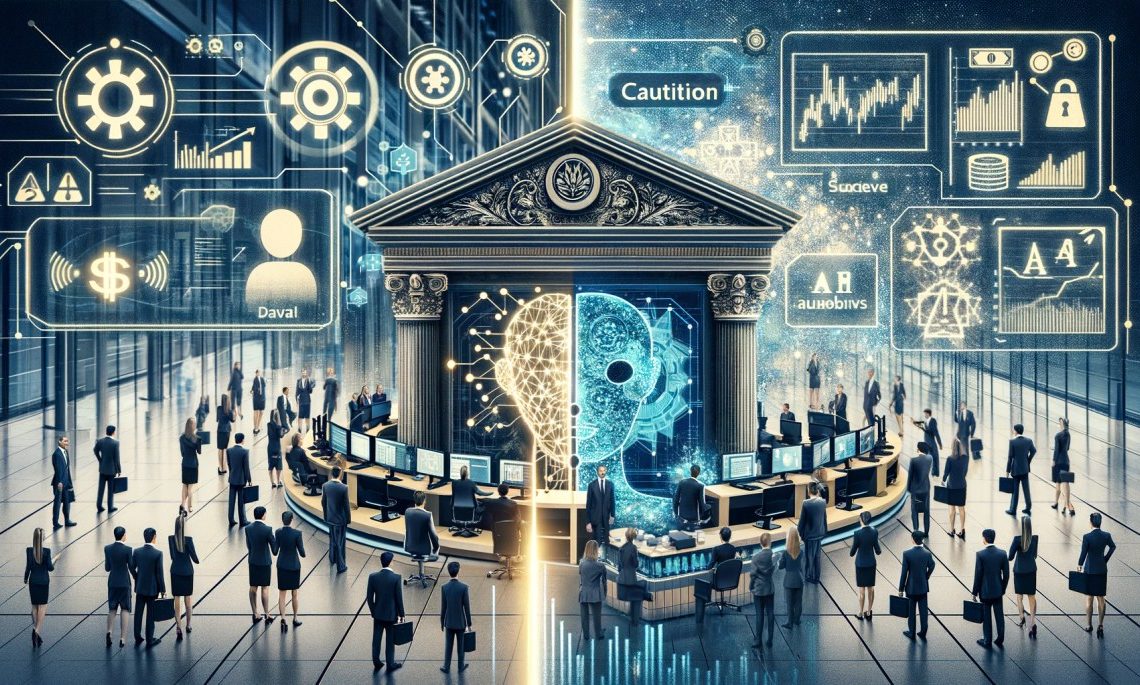
Generative AI has created a profound and positive shift inside of Citi toward data-driven decision making, but for now the nation’s top-three bank has decided against an external facing chatbot because the risks are still too high.
Those remarks, from Citi’s Promiti Dutta, head of analytics technology and innovation, came during a talk she gave during VB’s AI Impact Tour in New York on Friday.
“When I joined Citi four and a half years ago, data science or analytics before I even talk about AI, was often an afterthought. We used to think: ‘We’ll use analysis to prove a point that the business already had in mind,’” she said during a conversation that I moderated. “The advent of gen AI was a big paradigm shift for us,” she said. “It actually put data and analytics at the forefront of everything. All of a sudden, everyone wanted to solve everything with Gen AI. “
She said that this created a fun environment, where employees across the organization started proposing AI projects. The bank’s technology leaders realized not everything needed to be solved with gen AI, “but we didn’t say no, we actually let it happen. We could at least start having conversations around what data could do for them,” Dutta said. She welcomed the onset of the cultural curiosity around data. (See her full comments in the video below.)
The bank began to sort generative AI project priorities according to “meaningful outcomes that can drive time value and where there is certainty attached to them.”
Desirable projects fall into three main buckets. First was “agent assist,” where large language models (LLMs) can provide call center agents with summarized notes about what Citi knows about the customers, or jot down the notes more easily during the conversation and find information for the agent so they’re more easily able to respond to customer’s needs. It’s not customer-facing, but still providing information to the customer, she said.
Second, LLMs could automate manual tasks, such as reading through extensive compliance documents around things like risk and control, by summarizing texts and helping employees find documents they were looking for.
Finally, Citi internally created an internal search engine that centralized data into a single place, to let analysts and other Citi employees derive data-driven insights more easily. The bank is now integrating generative AI into the product so that employees can use natural language to create analysis on the fly, she said. The tool will be available for thousands of employees later this year, she said.
However, when it comes to using generative AI externally – to interact with customers via a support chatbot, for example – the bank has decided it is still too risky for prime time, she said.
Over the past year, there’s been a lot of publicity around how LLMs hallucinate, an inherent quality of generative AI that can be an asset in certain use cases where say, writers are looking for creativity, but can be problematic when precision is the goal: “Things can go wrong very quickly, and there’s a still a lot to be learned,” Dutta said.
“In an industry where every single customer interaction really matters, and everything we do has to build trust with customers, we can’t afford anything going wrong with any interaction,” she said.
She said in some industries LLMs are acceptable for external communication with customers, for example in a shopping experience where an LLM might suggest the wrong pair of shoes. A customer isn’t likely to get too upset with that, she said. “But if we tell you to get a loan product that you don’t necessarily want or need, you lose a little bit of interest in us because it’s like, “Oh, my bank really doesn’t understand who I am.”
The bank does use elements of conversational AI that became standard before generative AI emerged in late 2022, including natural language processing (NLP) responses that are pre-scripted, she said.
She said the bank hasn’t ruled out using LLMs externally in the future but needs to “work toward” it. The bank needs to make sure that there’s always a human in the loop, so that the bank learns what the technology can not do, and “branching out from there as the technology matures.” She noted that banks are also highly regulated and must go through a lot of testing and proofing before they can deploy new technology.
However, the approach contrasts with Wells Fargo, a bank that uses generative AI in its Fargo virtual assistant, which provides answers to customers’ everyday banking questions on their smartphone, using voice or text. The bank says Fargo is on track to hit a run rate of 100 million interactions a year, the bank’s CIO Chintan Mehta said during another talk I moderated in January. Fargo leverages multiple LLMs in its flow as it fulfills different tasks, he said. Wells Fargo also integrates LLMs in its Livesync product, which provides customers advice for goal-setting and planning.
Another way generative AI is transforming the bank is by forcing it to reevaluate where to use cloud resources, versus stay on-premise. The bank is exploring using OpenAI’s GPT models, through Azure’s cloud services, to do this, even though the bank has largely avoided cloud tools in the past, preferring to keep its infrastructure on-premise, Dutta said. The bank is also exploring open source models, like Llama and others that allow the bank to bring models in-house to use on its on-premise GPUs, she said.
An internal bank task force reviews all generative AI projects, in a process that goes all the way up to Jane Fraser, the bank’s chief executive, Dutta said. Fraser and the executive team are hands-on because it requires financial and other resource investments to make these projects happen. The task force makes sure any project is executed responsibly and that customers are safe during any usage of generative AI, Dutta said. The taskforce asks questions like: “What does it mean for our model risk management, what does it mean for our data security, what does it mean for how our data is being accessed by others?
Dutta said that generative AI has produced a unique environment where there’s enthusiasm from the top and the bottom rungs of the bank, to the point where there are too many hands in the pot, and perhaps a need to curb the enthusiasm.
Responding to Dutta’s talk, Sarah Bird, Microsoft’s global head of responsible AI engineering, said that Citi’s thorough approach to generative AI reflected best practice.
She said a lot of work is being put into fixing instances where LLMs can still make mistakes, even after they’ve been grounded with a source of truth. For example, many applications are being built with retrieval augmented generation (RAG), where the LLMs can query a data store to get the correct information to answer questions in real time, but that process still isn’t perfect.
“It can add additional information that wasn’t meant to be there,” Bird said, and she acknowledged that this is unacceptable in many applications.
Microsoft has been looking for ways to eliminate these kinds of grounding errors, Bird said, during a talk that followed Dutta’s, and which I also moderated. “That’s an area where we’ve actually seen a lot of progress and, you know, there’s still more to go there, but there are quite a few techniques that can greatly improve how effective that is.” She said Microsoft is spending a lot of time testing for this, and finding other ways to detect grounding errors. Microsoft is seeing “really rapid progress in terms of what’s possible and I think over the next year, I hope we can see a lot more.”
Full disclosure: Microsoft sponsored this New York event stop of VentureBeat’s AI Impact Tour, but the speakers from Citi and NewYork-Presbyterian were independently selected by VentureBeat. Check out our next stops on the AI Impact Tour, including how to apply for an invite for the next events in Boston on March 27 and Atlanta on April 10.
Join leaders in Boston on March 27 for an exclusive night of networking, insights, and conversation. Request an invite here.
Generative AI has created a profound and positive shift inside of Citi toward data-driven decision making, but for now the nation’s top-three bank has decided against an external facing chatbot because the risks are still too high.
Those remarks, from Citi’s Promiti Dutta, head of analytics technology and innovation, came during a talk she gave during VB’s AI Impact Tour in New York on Friday.
“When I joined Citi four and a half years ago, data science or analytics before I even talk about AI, was often an afterthought. We used to think: ‘We’ll use analysis to prove a point that the business already had in mind,’” she said during a conversation that I moderated. “The advent of gen AI was a big paradigm shift for us,” she said. “It actually put data and analytics at the forefront of everything. All of a sudden, everyone wanted to solve everything with Gen AI. “
Citi’s “three buckets” of generative AI applications
She said that this created a fun environment, where employees across the organization started proposing AI projects. The bank’s technology leaders realized not everything needed to be solved with gen AI, “but we didn’t say no, we actually let it happen. We could at least start having conversations around what data could do for them,” Dutta said. She welcomed the onset of the cultural curiosity around data. (See her full comments in the video below.)
The bank began to sort generative AI project priorities according to “meaningful outcomes that can drive time value and where there is certainty attached to them.”
Desirable projects fall into three main buckets. First was “agent assist,” where large language models (LLMs) can provide call center agents with summarized notes about what Citi knows about the customers, or jot down the notes more easily during the conversation and find information for the agent so they’re more easily able to respond to customer’s needs. It’s not customer-facing, but still providing information to the customer, she said.
Second, LLMs could automate manual tasks, such as reading through extensive compliance documents around things like risk and control, by summarizing texts and helping employees find documents they were looking for.
Finally, Citi internally created an internal search engine that centralized data into a single place, to let analysts and other Citi employees derive data-driven insights more easily. The bank is now integrating generative AI into the product so that employees can use natural language to create analysis on the fly, she said. The tool will be available for thousands of employees later this year, she said.
External facing LLMs are still too risky
However, when it comes to using generative AI externally – to interact with customers via a support chatbot, for example – the bank has decided it is still too risky for prime time, she said.
Over the past year, there’s been a lot of publicity around how LLMs hallucinate, an inherent quality of generative AI that can be an asset in certain use cases where say, writers are looking for creativity, but can be problematic when precision is the goal: “Things can go wrong very quickly, and there’s a still a lot to be learned,” Dutta said.
“In an industry where every single customer interaction really matters, and everything we do has to build trust with customers, we can’t afford anything going wrong with any interaction,” she said.
She said in some industries LLMs are acceptable for external communication with customers, for example in a shopping experience where an LLM might suggest the wrong pair of shoes. A customer isn’t likely to get too upset with that, she said. “But if we tell you to get a loan product that you don’t necessarily want or need, you lose a little bit of interest in us because it’s like, “Oh, my bank really doesn’t understand who I am.”
The bank does use elements of conversational AI that became standard before generative AI emerged in late 2022, including natural language processing (NLP) responses that are pre-scripted, she said.
Citi in learning process about how much LLMs can do
She said the bank hasn’t ruled out using LLMs externally in the future but needs to “work toward” it. The bank needs to make sure that there’s always a human in the loop, so that the bank learns what the technology can not do, and “branching out from there as the technology matures.” She noted that banks are also highly regulated and must go through a lot of testing and proofing before they can deploy new technology.
However, the approach contrasts with Wells Fargo, a bank that uses generative AI in its Fargo virtual assistant, which provides answers to customers’ everyday banking questions on their smartphone, using voice or text. The bank says Fargo is on track to hit a run rate of 100 million interactions a year, the bank’s CIO Chintan Mehta said during another talk I moderated in January. Fargo leverages multiple LLMs in its flow as it fulfills different tasks, he said. Wells Fargo also integrates LLMs in its Livesync product, which provides customers advice for goal-setting and planning.
Another way generative AI is transforming the bank is by forcing it to reevaluate where to use cloud resources, versus stay on-premise. The bank is exploring using OpenAI’s GPT models, through Azure’s cloud services, to do this, even though the bank has largely avoided cloud tools in the past, preferring to keep its infrastructure on-premise, Dutta said. The bank is also exploring open source models, like Llama and others that allow the bank to bring models in-house to use on its on-premise GPUs, she said.
LLMs are driving internal transformation at Citi
An internal bank task force reviews all generative AI projects, in a process that goes all the way up to Jane Fraser, the bank’s chief executive, Dutta said. Fraser and the executive team are hands-on because it requires financial and other resource investments to make these projects happen. The task force makes sure any project is executed responsibly and that customers are safe during any usage of generative AI, Dutta said. The taskforce asks questions like: “What does it mean for our model risk management, what does it mean for our data security, what does it mean for how our data is being accessed by others?
Dutta said that generative AI has produced a unique environment where there’s enthusiasm from the top and the bottom rungs of the bank, to the point where there are too many hands in the pot, and perhaps a need to curb the enthusiasm.
Responding to Dutta’s talk, Sarah Bird, Microsoft’s global head of responsible AI engineering, said that Citi’s thorough approach to generative AI reflected best practice.
Microsoft is working to fix LLM mistakes
She said a lot of work is being put into fixing instances where LLMs can still make mistakes, even after they’ve been grounded with a source of truth. For example, many applications are being built with retrieval augmented generation (RAG), where the LLMs can query a data store to get the correct information to answer questions in real time, but that process still isn’t perfect.
“It can add additional information that wasn’t meant to be there,” Bird said, and she acknowledged that this is unacceptable in many applications.
Microsoft has been looking for ways to eliminate these kinds of grounding errors, Bird said, during a talk that followed Dutta’s, and which I also moderated. “That’s an area where we’ve actually seen a lot of progress and, you know, there’s still more to go there, but there are quite a few techniques that can greatly improve how effective that is.” She said Microsoft is spending a lot of time testing for this, and finding other ways to detect grounding errors. Microsoft is seeing “really rapid progress in terms of what’s possible and I think over the next year, I hope we can see a lot more.”
Full disclosure: Microsoft sponsored this New York event stop of VentureBeat’s AI Impact Tour, but the speakers from Citi and NewYork-Presbyterian were independently selected by VentureBeat. Check out our next stops on the AI Impact Tour, including how to apply for an invite for the next events in Boston on March 27 and Atlanta on April 10.
VentureBeat’s mission is to be a digital town square for technical decision-makers to gain knowledge about transformative enterprise technology and transact. Discover our Briefings.
Author: Matt Marshall
Source: Venturebeat
Reviewed By: Editorial Team