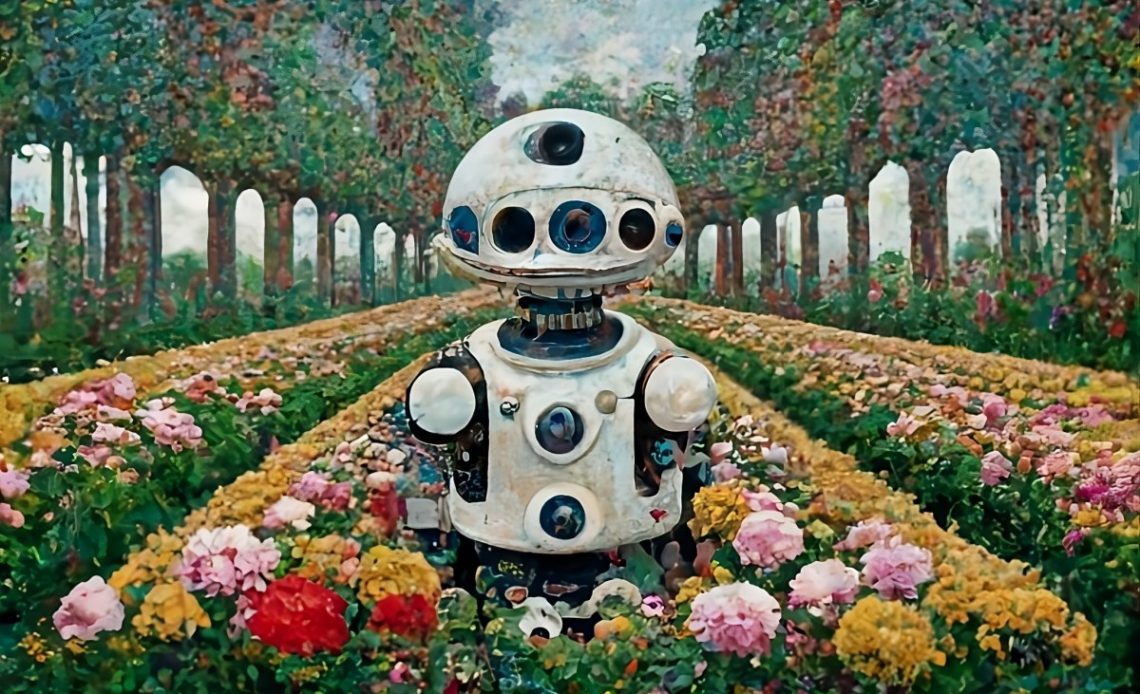
By any measure, 2023 was an amazing year for AI. Large language Models (LLMs) and their chatbot applications stole the show, but there were advances across a broad swath of uses. These include image, video and voice generation.
The combination of these digital technologies have led to new use cases and business models, even to the point where digital humans are becoming commonplace, replacing actual humans as influencers and newscasters.
Importantly, 2023 was the year when large numbers of people started to use and adopt AI intentionally as part of their daily work. Rapid AI innovation has fueled future predictions, as well, including everything from friendly home robots to artificial general intelligence (AGI) within a decade. That said, progress is never a straight line and challenges could sidetrack some of these predicted advances.
As AI increasingly weaves into the fabric of our daily lives and work, it begs the question: What can we expect next?”
While digital advancements continue to astonish, the physical realm of AI — particularly robotics — is not far behind in capturing our imagination. LLMs could provide the missing piece, essentially a brain, particularly when combined with image recognition capabilities through camera vision. With these technologies, robots could more readily understand and respond to requests and perceive the world around them.
In the Robot Report, Nvidia’s VP of robots and edge computing Deepu Talla said that LLMs will enable robots to better understand human instructions, learn from one another and comprehend their environments.
One way to improve robot performance is to use multiple models. MIT’s Improbable AI Lab, a group within the Computer Science and Artificial Intelligence Laboratory (CSAIL), for instance, has developed a framework that makes use of three different foundation models each tuned for specific tasks such as language, vision and action.
“Each foundation model captures a different part of the [robot] decision-making process and then works together when it’s time to make decisions,” lab researchers report.
Incorporating these models may not be enough for robots to be widely usable and practical in the real world. To address these limitations, a new AI system called Mobile ALOHA has been developed at Stanford University.
This system allows robots “to autonomously complete complex mobile manipulation tasks such as sautéing and serving a piece of shrimp, opening a two-door wall cabinet to store heavy cooking pots, calling and entering an elevator and lightly rinsing a used pan using a kitchen faucet.”
This led Jack Clark to opine in his ImportAI newsletter: “Robots may be nearing their ‘ImageNet moment’ when both the cost of learning robot behaviors falls, as does the data for learning their behaviors.”
ImageNet is a large dataset of labeled images started by Fei Fei Lee in 2006 and is widely used in advancing computer vision and deep learning research. Starting in 2010, ImageNet served as the dataset for an annual competition aimed at assessing the performance of computer vision algorithms in image classification, object detection and localization tasks.
The moment Clark references is from 2012, when several AI researchers including Alex Krizhevsky along with Ilya Sutskever and Geoffrey Hinton developed a convolutional neural network (CNN) architecture, a form of deep learning, that achieved a dramatic reduction in image classification error rates.
This moment demonstrated the potential of deep learning, and is what effectively jumpstarted the modern AI era. Clark’s view is that the industry could now be at a similar moment for physical robots. If true, biped robots could be collaborating with us within a decade, in hospitals and factories, in stores or helping at home. Imagine a future where your household chores are effortlessly managed by AI-powered robots.
Many such inflection points could be near. Nvidia CEO Jensen Huang said recently that AGI, the point at which AI can perform at human levels across a wide variety of tasks, might be achieved within five years. Jim Fan, senior research scientist and lead of AI agents at Nvidia, added that “the past year in AI is like leaping from Stone Age to Space Age.”
Consulting giant McKinsey has estimated that gen AI will add more than $4 trillion a year to the global economy. Securities from UBS recently updated their perspective on AI, calling it the tech theme of the decade and predicted the AI market will grow from $2.2 billion in 2022 to $225 billion by 2027. That represents a 152% compound annual growth rate (CAGR), a truly astonishing number.
Enthusiasm for the potential of AI to improve our quality of life runs high. Bill Gates said in his “Gates Notes” letter at the end of 2023 that “AI is about to supercharge the innovation pipeline.” A New York Times article quotes David Luan, CEO of AI start-up company Adept: “The rapid progress of A.I. will continue. It is inevitable.”
Given all of this, it shouldn’t come as a surprise that gen AI is at the peak of inflated expectations according to the Gartner Emerging Technology Hype Cycle, a gauge of enthusiasm for new technologies.
As we revel in the achievements of AI in 2023, we must also ponder what challenges lie ahead in the aftermath of this rapid growth period. The momentum behind AI is unlike anything we have ever seen, at least since the Internet boom that fueled the dot com era — and we saw how that turned out.
Might something like that occur with the AI boom in 2024? A Fortune article suggests as much: “This year is likely to be one of retrenchment, as investors discover many of the companies they threw money at don’t have a workable business model, and many big companies find that the cost of compute outweighs the benefit.”
That view aligns with Amara’s Law that states: “We tend to overestimate the effect of a technology in the short run and underestimate the effect in the long run.” Which is another way of stating that systems attempt to rebalance after disruption, or that hype often outpaces reality.
This view does not necessarily portend the AI industry falling from grace, although it has happened twice before. Since it was originally coined as a term at a 1956 Dartmouth College conference, AI has had at least two periods of elevated expectations that ended due to problems encountered in building and deploying applications when the speculative promises did not materialize. The periods, known as “AI winters,” occurred from 1974 to 1980 and again from 1987 to 1993.
Now amid a brilliant “AI summer,” is there a risk of another winter? In addition to the cost of computing, there are also issues with energy use in AI model training and inference that is running into a headwind of climate change and sustainability concerns.
Then too, there are what are sometimes referred to as the “Four Horsemen of the AI-pocalypse:” data bias, data security, copyright infringement and hallucination. The copyright issue is the most immediate, with the recent lawsuit brought by the New York Times against OpenAI and Microsoft. If the Times wins, some commentators have speculated, it could end the entire business model on which many gen AI companies have been built.
The biggest concern of all is the potential existential threat from AI. While some would welcome the advent of AGI, seeing this as a pathway to unlimited abundance, many others led by proponents of Effective Altruism are fearful that this could lead to the destruction of humanity.
A new survey of more than 2,700 AI researchers reveals the current extent of these existential fears. “Median respondents put 5% or more on advanced AI leading to human extinction or similar, and a third to a half of participants gave 10% or more.”
If nothing else, the known and potential problems function as a brake on AI enthusiasm. For now, however, the momentum marches forward as predictions abound for continued AI advances in 2024.
For example, the New York Times states: “The AI industry this year is set to be defined by one main characteristic: A remarkably rapid improvement of the technology as advancements build upon one another, enabling AI to generate new kinds of media, mimic human reasoning in new ways and seep into the physical world through a new breed of robot.”
Ethan Mollick, writing in his One Useful Thing blog, takes a similar view: “Most likely, AI development is actually going to accelerate for a while yet before it eventually slows down due to technical or economic or legal limits.”
The year ahead in AI will undoubtedly bring dramatic changes. Hopefully, these will include advances that improve our quality of life, such as the discovery of life saving new drugs. Likely, the most optimistic promises will not be realized in 2024, leading to some amount of pullback in market expectations. This is the nature of hype cycles. Hopefully, any such disappointments will not bring about another AI winter.
Gary Grossman is EVP of technology practice at Edelman and global lead of the Edelman AI Center of Excellence.
By any measure, 2023 was an amazing year for AI. Large language Models (LLMs) and their chatbot applications stole the show, but there were advances across a broad swath of uses. These include image, video and voice generation.
The combination of these digital technologies have led to new use cases and business models, even to the point where digital humans are becoming commonplace, replacing actual humans as influencers and newscasters.
Importantly, 2023 was the year when large numbers of people started to use and adopt AI intentionally as part of their daily work. Rapid AI innovation has fueled future predictions, as well, including everything from friendly home robots to artificial general intelligence (AGI) within a decade. That said, progress is never a straight line and challenges could sidetrack some of these predicted advances.
As AI increasingly weaves into the fabric of our daily lives and work, it begs the question: What can we expect next?”
Physical robots could arrive soon
While digital advancements continue to astonish, the physical realm of AI — particularly robotics — is not far behind in capturing our imagination. LLMs could provide the missing piece, essentially a brain, particularly when combined with image recognition capabilities through camera vision. With these technologies, robots could more readily understand and respond to requests and perceive the world around them.
In the Robot Report, Nvidia’s VP of robots and edge computing Deepu Talla said that LLMs will enable robots to better understand human instructions, learn from one another and comprehend their environments.
One way to improve robot performance is to use multiple models. MIT’s Improbable AI Lab, a group within the Computer Science and Artificial Intelligence Laboratory (CSAIL), for instance, has developed a framework that makes use of three different foundation models each tuned for specific tasks such as language, vision and action.
“Each foundation model captures a different part of the [robot] decision-making process and then works together when it’s time to make decisions,” lab researchers report.
Incorporating these models may not be enough for robots to be widely usable and practical in the real world. To address these limitations, a new AI system called Mobile ALOHA has been developed at Stanford University.
This system allows robots “to autonomously complete complex mobile manipulation tasks such as sautéing and serving a piece of shrimp, opening a two-door wall cabinet to store heavy cooking pots, calling and entering an elevator and lightly rinsing a used pan using a kitchen faucet.”
An ImageNet moment for robotics
This led Jack Clark to opine in his ImportAI newsletter: “Robots may be nearing their ‘ImageNet moment’ when both the cost of learning robot behaviors falls, as does the data for learning their behaviors.”
ImageNet is a large dataset of labeled images started by Fei Fei Lee in 2006 and is widely used in advancing computer vision and deep learning research. Starting in 2010, ImageNet served as the dataset for an annual competition aimed at assessing the performance of computer vision algorithms in image classification, object detection and localization tasks.
The moment Clark references is from 2012, when several AI researchers including Alex Krizhevsky along with Ilya Sutskever and Geoffrey Hinton developed a convolutional neural network (CNN) architecture, a form of deep learning, that achieved a dramatic reduction in image classification error rates.
This moment demonstrated the potential of deep learning, and is what effectively jumpstarted the modern AI era. Clark’s view is that the industry could now be at a similar moment for physical robots. If true, biped robots could be collaborating with us within a decade, in hospitals and factories, in stores or helping at home. Imagine a future where your household chores are effortlessly managed by AI-powered robots.
The pace of AI advancement is breathtaking
Many such inflection points could be near. Nvidia CEO Jensen Huang said recently that AGI, the point at which AI can perform at human levels across a wide variety of tasks, might be achieved within five years. Jim Fan, senior research scientist and lead of AI agents at Nvidia, added that “the past year in AI is like leaping from Stone Age to Space Age.”

Consulting giant McKinsey has estimated that gen AI will add more than $4 trillion a year to the global economy. Securities from UBS recently updated their perspective on AI, calling it the tech theme of the decade and predicted the AI market will grow from $2.2 billion in 2022 to $225 billion by 2027. That represents a 152% compound annual growth rate (CAGR), a truly astonishing number.
Enthusiasm for the potential of AI to improve our quality of life runs high. Bill Gates said in his “Gates Notes” letter at the end of 2023 that “AI is about to supercharge the innovation pipeline.” A New York Times article quotes David Luan, CEO of AI start-up company Adept: “The rapid progress of A.I. will continue. It is inevitable.”
Given all of this, it shouldn’t come as a surprise that gen AI is at the peak of inflated expectations according to the Gartner Emerging Technology Hype Cycle, a gauge of enthusiasm for new technologies.

Is AI progress inevitable?
As we revel in the achievements of AI in 2023, we must also ponder what challenges lie ahead in the aftermath of this rapid growth period. The momentum behind AI is unlike anything we have ever seen, at least since the Internet boom that fueled the dot com era — and we saw how that turned out.
Might something like that occur with the AI boom in 2024? A Fortune article suggests as much: “This year is likely to be one of retrenchment, as investors discover many of the companies they threw money at don’t have a workable business model, and many big companies find that the cost of compute outweighs the benefit.”
That view aligns with Amara’s Law that states: “We tend to overestimate the effect of a technology in the short run and underestimate the effect in the long run.” Which is another way of stating that systems attempt to rebalance after disruption, or that hype often outpaces reality.
This view does not necessarily portend the AI industry falling from grace, although it has happened twice before. Since it was originally coined as a term at a 1956 Dartmouth College conference, AI has had at least two periods of elevated expectations that ended due to problems encountered in building and deploying applications when the speculative promises did not materialize. The periods, known as “AI winters,” occurred from 1974 to 1980 and again from 1987 to 1993.

Not all rainbows and unicorns
Now amid a brilliant “AI summer,” is there a risk of another winter? In addition to the cost of computing, there are also issues with energy use in AI model training and inference that is running into a headwind of climate change and sustainability concerns.
Then too, there are what are sometimes referred to as the “Four Horsemen of the AI-pocalypse:” data bias, data security, copyright infringement and hallucination. The copyright issue is the most immediate, with the recent lawsuit brought by the New York Times against OpenAI and Microsoft. If the Times wins, some commentators have speculated, it could end the entire business model on which many gen AI companies have been built.
The biggest concern of all is the potential existential threat from AI. While some would welcome the advent of AGI, seeing this as a pathway to unlimited abundance, many others led by proponents of Effective Altruism are fearful that this could lead to the destruction of humanity.
A new survey of more than 2,700 AI researchers reveals the current extent of these existential fears. “Median respondents put 5% or more on advanced AI leading to human extinction or similar, and a third to a half of participants gave 10% or more.”
A balanced perspective
If nothing else, the known and potential problems function as a brake on AI enthusiasm. For now, however, the momentum marches forward as predictions abound for continued AI advances in 2024.
For example, the New York Times states: “The AI industry this year is set to be defined by one main characteristic: A remarkably rapid improvement of the technology as advancements build upon one another, enabling AI to generate new kinds of media, mimic human reasoning in new ways and seep into the physical world through a new breed of robot.”
Ethan Mollick, writing in his One Useful Thing blog, takes a similar view: “Most likely, AI development is actually going to accelerate for a while yet before it eventually slows down due to technical or economic or legal limits.”
The year ahead in AI will undoubtedly bring dramatic changes. Hopefully, these will include advances that improve our quality of life, such as the discovery of life saving new drugs. Likely, the most optimistic promises will not be realized in 2024, leading to some amount of pullback in market expectations. This is the nature of hype cycles. Hopefully, any such disappointments will not bring about another AI winter.
Gary Grossman is EVP of technology practice at Edelman and global lead of the Edelman AI Center of Excellence.
DataDecisionMakers
Welcome to the VentureBeat community!
DataDecisionMakers is where experts, including the technical people doing data work, can share data-related insights and innovation.
If you want to read about cutting-edge ideas and up-to-date information, best practices, and the future of data and data tech, join us at DataDecisionMakers.
You might even consider contributing an article of your own!
Author: Gary Grossman, Edelman
Source: Venturebeat
Reviewed By: Editorial Team